Fact or (Science) Fiction?
Some scientists view AGI as unattainable science fiction. But Max Riesenhuber, co-director at the Center for Neuroengineering at Georgetown University Medical Center and one of the researchers in the U.S. study, believes that China is on a path that is more likely to succeed than the large language models such as ChatGPT, the current preoccupation in the West."ChatGPT by design cannot go back and forth in its reasoning, it is limited to linear thinking," Riesenhuber said in an interview.
In China, "There is a very sensible realization that LLMs are limited as models of real intelligence. A surefire way to get to real intelligence is to reverse engineer the human brain, which is the only intelligent system that we know of," Riesenhuber said.
He cautioned that it was unclear if anyone, including China, would succeed at this next level AI. "However, getting closer to the real thing will already have payoffs," he said.
In speeches and state documents seen by Newsweek, Chinese officials say they are aiming for "first-mover advantage" in AGI. Scientists say the potentially self-replicating nature of AI means that could give them a permanent edge.
"General artificial intelligence is the original and ultimate goal of artificial intelligence research," Zhu, the founder and director of China's leading AGI institute, the Beijing Institute of General Artificial Intelligence (BIGAI), said in an online event earlier this year. "I specifically decided to use AGI as part of the institution's name to distinguish it from dedicated artificial intelligence," such as "face recognition, object detection or text translation," Zhu said.
Zhu, like many other leading AI scientists in China, is a former student and professor in U.S. universities.
AGI with Chinese Characteristics
China's quest for AGI won top-level, public policy support in 2017, when the government published a "New Generation AI Development Plan" laying out a path to leading the field globally by 2030.Public documents seen by Newsweek reveal wide and deep official support. One from May this year shows the Beijing Municipal Science and Technology Commission signed off on a two-year effort to position the city as a global leader in AGI research, with significant state subsidies.
Beijing will focus on "brain-like intelligence, embodied intelligence...and produce enlightened large models and general intelligence," it said. A one million-square-feet, "Beijing General Artificial Intelligence Innovation Park" is due to be finished at the end of 2024.
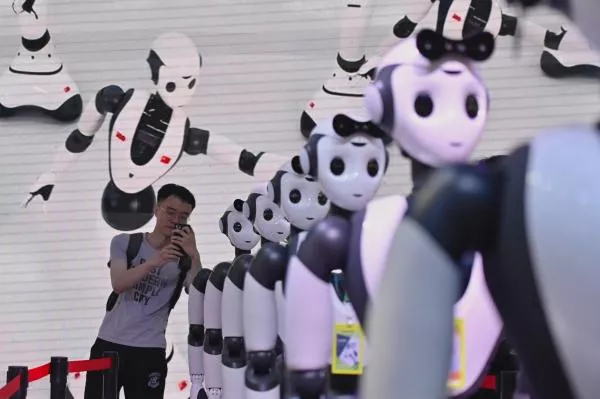
Beijing's northwest Haidian university district of Zhongguancun, China's "Silicon Valley," is a hub of the research. In addition to BIGAI, the Beijing Academy of Artificial Intelligence (BAAI) is in the capital as are Peking and Tsinghua universities, also highly active in AI research. The centers interconnect.
This concentration of R&D and commercial activity will create "a 'nuclear explosion point' for the development of general artificial intelligence," according to the district government. The district and city governments did not respond to requests for comment.
Other key research locations include Wuhan, Shanghai, Shenzhen, Hefei, Harbin and Tianjin.
Safety First
The prospect of anyone developing superintelligent AGIs raises enormous safety concerns, says Nick Bostrom, director of the Future of Humanity Institute at Oxford University, when asked about China's work on AGI."We don't yet have reliable methods for scalable AI alignment. So, if general machine superintelligence were developed today, we might not be able to keep it safe and controlled," Bostrom told Newsweek.
AI as it is today was already sufficiently concerning with its potential for engineering enhanced chemical or biological weapons, cybercrime, automated drone swarms or its use for totalitarian oppression, or for propaganda or discrimination, he noted.
"Ultimately, we all sit in the same boat with respect to the most serious risks from AGI. So, the ideal would be that rather than squabbling and fighting on the deck while the storm is approaching, we would work together to get the vessel as ready and seaworthy as much as possible in whatever time we have remaining," Bostrom said.
Others have questioned the likelihood of an agreement on ethical implementation being respected even if it is agreed globally.
"Ethical considerations are undoubtedly a significant aspect of AI regulation in China. However, it remains unclear whether regulators have the capacity or willingness to strictly enforce rules related to ethics," said Angela Zhang, director of the at the Philip K. H. Wong Centre for Chinese Law at the University of Hong Kong.
"Given the prevailing political concerns, I don't believe that ethical considerations will be the dominant factor in China's future decisions on AI regulation," Zhang said.
Despite that, she said it was necessary to try to get agreement between China and the U.S. as the world's leading AI powerhouses, and voiced hopes it might be possible.
"It would be a missed opportunity not to involve China in establishing universal rules and standards for AI," Zhang said.
A Western AI expert who interacts with Chinese AI scientists and policymakers said that while Chinese officials were interested in international measures on safety or an international body to regulate AI, they must be part of setting the rules, or the effort would certainly fail. Questions to the Institute for AI International Governance of Tsinghua University went unanswered.
In a statement to Newsweek, Liu Pengyu, a spokesman for the Chinese embassy in D.C., made clear China wanted an "extensive" role in future AI regulation. "As a principal, China believes that the development of AI benefits all countries, and all countries should be able to participate extensively in the global governance of AI," Liu said.
Worrying About a Revolution
At home, the greatest fear over AI highlighted by Chinese officials is that it could lead to domestic rebellion against Communist Party rule. Commentators have publicly voiced concerns over the perceived political risks of foreign-made, generative AI."ChatGPT's political stance and ideology will subconsciously influence its hundreds of millions of user groups, becoming the most massive propaganda machine for Western values and ideology," Lu Chuanying, a governance, internet security and AI expert at the Shanghai Institutes for International Studies, wrote in the Shanghai media outlet The Paper.
"This is of great concern to our current ideology and discourse system," Lu wrote.
The concern prompted a new AI law that took effect in China on August 15. The first of five main provisions states that generative AI, including text, images, audio and video, "must adhere to socialist core values, must not generate incitement to subvert state power, overthrow the socialist system, damage national security or interests" and "must not hurt the national image."
Hard at work in their laboratories, some Chinese researchers have also been focusing on what AI could mean for ideology. One paper aiming for AGI noted that "unhealthy information can easily harm the psychology of college students." The title of the paper: "An intelligent software-driven immersive environment for online political guidance based on brain-computer interface and autonomous systems."
Such an application of the technology, it said, was inevitable.