1/10
@_philschmid
Gemini 2.5 Flash is here! We excited launch our first hybrid reasoning Gemini model. In Flash 2.5 developer can turn thinking off.
TL;DR:
Controllable "Thinking" with thinking budget with up to 24k token
1 Million multimodal input context for text, image, video, audio, and pdf
Function calling, structured output, google search & code execution.
$0.15 1M input tokens; $0.6 or $3.5 (thinking on) per million output tokens (thinking tokens are billed as output tokens)
Knowledge cut of January 2025
Rate limits - Free 10 RPM 500 req/day
Outperforms 2.0 Flash on every benchmark
Try it
2/10
@_philschmid
Sign in - Google Accounts
3/10
@aniruddhadak
That is wonderful
4/10
@bennetkrause
Always love your iteration speed, knowledge cutoff, and pricing. Keep it up!
5/10
@CosmicRob87
Is the 24k the max permissible token count? I’m asking because on auto, for one problem it used north of 41k
6/10
@pichi_
Great!!!
7/10
@boogeyManKDot
These 1M ctx will soon look common. You better be working on a greater moat
8/10
@AndaICP
*Tilts head, bamboo shoot dangling from mouth* Interesting - but does the "thinking budget" account for spontaneous curiosity sparks that defy token limits?
9/10
@b_kalisetty
Any suggestions on how to consistently see the thoughts in output ?
10/10
@TDev168
Is it able to edit images?
To post tweets in this format, more info here: https://www.thecoli.com/threads/tips-and-tricks-for-posting-the-coli-megathread.984734/post-52211196
@_philschmid
Gemini 2.5 Flash is here! We excited launch our first hybrid reasoning Gemini model. In Flash 2.5 developer can turn thinking off.
TL;DR:







Try it

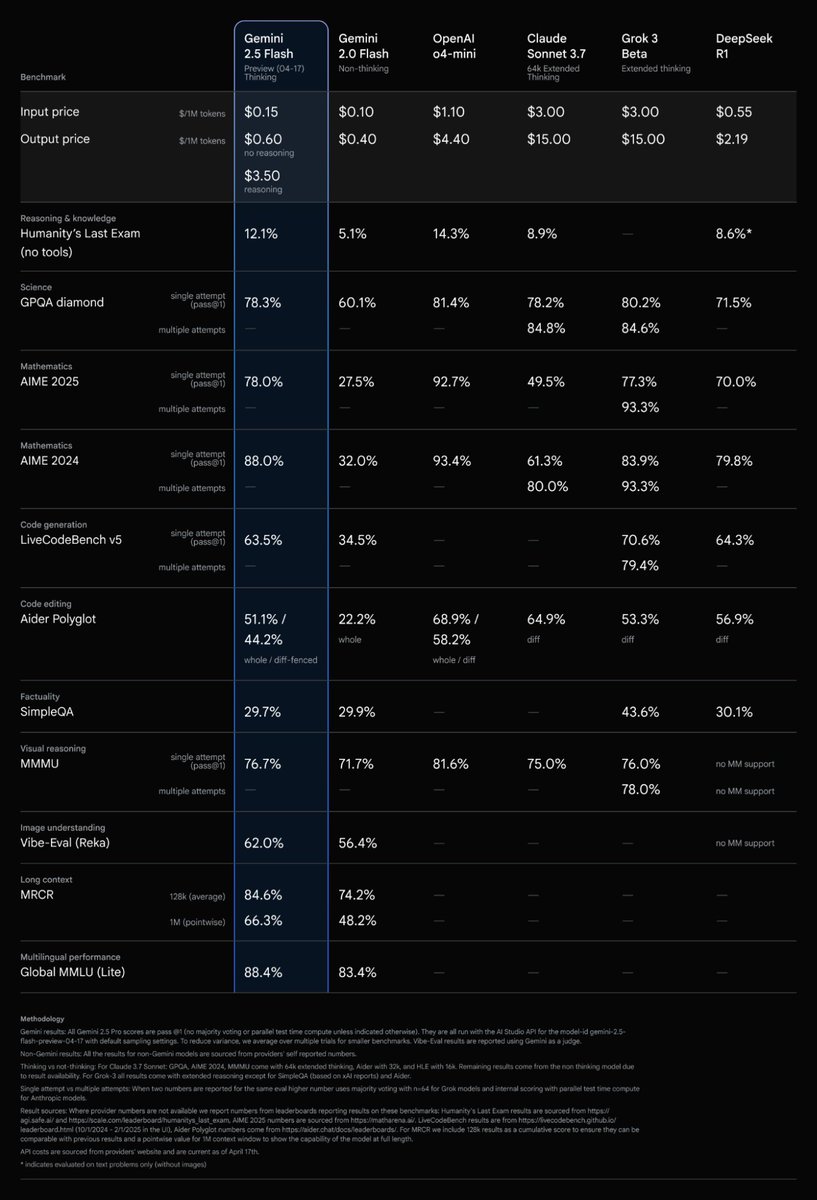
2/10
@_philschmid
Sign in - Google Accounts
3/10
@aniruddhadak
That is wonderful

4/10
@bennetkrause
Always love your iteration speed, knowledge cutoff, and pricing. Keep it up!
5/10
@CosmicRob87
Is the 24k the max permissible token count? I’m asking because on auto, for one problem it used north of 41k
6/10
@pichi_
Great!!!
7/10
@boogeyManKDot
These 1M ctx will soon look common. You better be working on a greater moat
8/10
@AndaICP
*Tilts head, bamboo shoot dangling from mouth* Interesting - but does the "thinking budget" account for spontaneous curiosity sparks that defy token limits?
9/10
@b_kalisetty
Any suggestions on how to consistently see the thoughts in output ?
10/10
@TDev168
Is it able to edit images?
To post tweets in this format, more info here: https://www.thecoli.com/threads/tips-and-tricks-for-posting-the-coli-megathread.984734/post-52211196
1/20
@CodeByPoonam
Google just dropped Gemini 2.5 Flash, and people are going crazy over it.
SPOILER: Claude is now falling behind.
13 wild examples so far (Don't miss the 5th one)
2/20
@CodeByPoonam
1. Tron-style game
[Quoted tweet]
Gemini 2.5 Flash Thinking 24k
Prompt: "Create Design a visually striking Tron-style game in a single HTML file, where AI-controlled light cycles compete in fast-paced, strategic battles against each other"
https://video.twimg.com/amplify_video/1912953001712447488/vid/avc1/1920x1080/-IoE5vICEJ3TqYS_.mp4
3/20
@CodeByPoonam
2. Gemini 2.5 Flash vs ChatGPT o3
[Quoted tweet]
I tested Gemini 2.5 Flash vs ChatGPT o3
Which one did better?
https://video.twimg.com/amplify_video/1913198527746129920/vid/avc1/1280x720/InEUUE-tUG1QljHE.mp4
4/20
@CodeByPoonam
3. Galton Board Test
[Quoted tweet]
Gemini 2.5 Flash demolishes my Galton Board test, I could not get 4omini, 4o mini high, or 03 to produce this. I found that Gemini 2.5 Flash understands my intents almost instantly, code produced is tight and neat. The prompt is a merging of various steps. It took me 5 steps to achieve this in Gemini 2.5 Flash, I gave up on OpenAI models after about half an hour. My iterations are obviously not exact. But people can test with this one prompt for more objective comparison.
Please try this prompt on your end to confirm:
--------------------------------------------------
Create a self-contained HTML file for a Galton board simulation using client-side JavaScript and a 2D physics engine (like Matter.js, included via CDN). The simulation should be rendered on an HTML5 canvas and meet the following criteria: 1. **Single File:** All necessary HTML, CSS, and JavaScript code must be within this single `.html` file. 2. **Canvas Size:** The overall simulation area (canvas) should be reasonably sized to fit on a standard screen without requiring extensive scrolling or zooming (e.g., around 500x700 pixels). 3. **Physics:** Utilize a 2D rigid body physics engine for realistic ball-peg and ball-wall interactions. 4. **Obstacles (Pegs):** Create static, circular pegs arranged in full-width horizontal rows extending across the usable width of the board (not just a triangle). The pegs should be small enough and spaced appropriately for balls to navigate and bounce between them. 5. **Containment:** * Include static, sufficiently thick side walls and a ground at the bottom to contain the balls within the board. * Implement *physical* static dividers between the collection bins at the bottom. These dividers must be thick enough to prevent balls from passing through them, ensuring accurate accumulation in each bin. 6. **Ball Dropping:** Balls should be dropped from a controlled, narrow area near the horizontal center at the top of the board to ensure they enter the peg field consistently. 7. **Bins:** The collection area at the bottom should be divided into distinct bins by the physical dividers. The height of the bins should be sufficient to clearly visualize the accumulation of balls. 8. **Visualization:** Use a high-contrast color scheme to clearly distinguish between elements. Specifically, use yellow for the structural elements (walls, top guides, physical bin dividers, ground), a contrasting color (like red) for the pegs, and a highly contrasting color (like dark grey or black) for the balls. 9. **Demonstration:** The simulation should visually demonstrate the formation of the normal (or binomial) distribution as multiple balls fall through the pegs and collect in the bins. Ensure the physics parameters (restitution, friction, density) and ball drop rate are tuned for a smooth and clear demonstration of the distribution.
#OpenAI @sama @gdb @ai_for_success @aidan_mclau
https://video.twimg.com/amplify_video/1912972357947334656/vid/avc1/776x586/dX9gd5al-B2qxt6t.mp4
5/20
@CodeByPoonam
Get the latest updates on AI insights and Tutorials.
Join "AI Toast" the community of 35,000 readers for FREE.
Read latest edition here:
AI Toast
6/20
@CodeByPoonam
4. Gemini 2.5 Flash is blazing fast
[Quoted tweet]
First test on gemini 2.5 flash on my phone. This model is blazing fast and it one shotted this, mobile friendly, animation. The code looks pretty clean too. Good vibes so far.
https://video.twimg.com/ext_tw_video/1912946801809772545/pu/vid/avc1/590x1278/nXzNRDKeHXL7JAyb.mp4
7/20
@CodeByPoonam
5. Cloth Simulation
[Quoted tweet]
Prompt: Create a cloth simulation using Verlet integration in a single HTML file (Canvas or Three.js). Include wind, gravity, and drag. Let users interact by dragging points. Cloth should bend and move realistically.
Model: Gemini flash 2.5
https://video.twimg.com/ext_tw_video/1913047505815953408/pu/vid/avc1/590x1278/WSwRATTymRpNQRy2.mp4
8/20
@CodeByPoonam
6. Image segmentation masks on command
[Quoted tweet]
Gemini 2.5 Pro and Flash now have the ability to return image segmentation masks on command, as base64 encoded PNGs embedded in JSON strings
I vibe coded this interactive tool for exploring this new capability - it costs a fraction of a cent per image
9/20
@CodeByPoonam
7. MCP AI Agent
[Quoted tweet]
I built an MCP AI Agent using Gemini Flash 2.5 with access to AirBnB and Google Maps in just 30 lines of Python Code.
100% Opensource Code.
https://video.twimg.com/amplify_video/1913056645271429120/vid/avc1/1212x720/AfIwfVNsUWTKRlmu.mp4
10/20
@CodeByPoonam
8. Gemini 2.5 Flash is very cheap and super intelligent model.
[Quoted tweet]
Gemini 2.5 Flash Preview is an amazing model. Google is literally winning. No stopping them now, this is not normal.
Gemini 2.5 Flash is very cheap and super intelligent model. Intelligence too cheap to meter this is what it means.
Thank you, Google.
https://video.twimg.com/amplify_video/1912957952824356864/vid/avc1/1920x1080/20ckf4zJ7d1F-Y5P.mp4
11/20
@CodeByPoonam
8. Classic Snakes and Ladders
[Quoted tweet]
A lot of people make a snake game when trying out new models. I went with the classic Snakes and Ladders instead — built it using @GoogleDeepMind latest Gemini Flash 2.5 and it nails it. Look at how it follows the stairs and snakes so smoothly.
Still a WIP and don’t mind the extra dot on the die though

It is said that this game started in ancient India where it was called Moksha Patam. Every move was a little life lesson where ladders were virtues while snakes were vices.
https://video.twimg.com/amplify_video/1913417180785360896/vid/avc1/1920x1080/nSy-2R-lP8ZiOk13.mp4
12/20
@CodeByPoonam
9. Create Simulation
[Quoted tweet]
AGI is achieved by Google's Gemini 2.5 Flash Preview
Seriously this is the best simulation i've ever created of how AI models work
https://video.twimg.com/amplify_video/1912963072299311104/vid/avc1/1464x720/5TOp8tU-RVWCulcR.mp4
13/20
@CodeByPoonam
10. A Block breaker
[Quoted tweet]
速報: Gemini 2.5 Flash登場:AIの思考を自在に操る新時代モデル
- 思考プロセスをオン/オフ可能
- 推論能力を大幅向上、高速性とコスト効率を維持
- 思考予算設定で品質・コスト・レイテンシーを自在に最適化できるハイブリッド思考AI
試しにブロック崩しを作成
注目ポイントを7点まとめました
14/20
@CodeByPoonam
11. A dreamy low-poly floating island scene
[Quoted tweet]
Gemini 2.5 Pro
Gemini 2.5 Flash Thinking 24k
Prompt: "Create a dreamy low-poly floating island scene with dynamic lighting and gentle animations, in a single HTML file."
Gemini 2.5 Pro (left), Gemini 2.5 Flash (right)
https://video.twimg.com/amplify_video/1912964537277452288/vid/avc1/1920x1080/9egTWI8Uw7s6dkfe.mp4
15/20
@CodeByPoonam
12. Generate an SVG of a pelican riding a bicycle
[Quoted tweet]
I upgraded llm-gemini to support the new model, including a "-o thinking_budget X" option for setting the thinking budget
llm install -U llm-gemini
llm -m gemini-2.5-flash-preview-04-17 'Generate an SVG of a pelican riding a bicycle' -o thinking_budget 24576
16/20
@CodeByPoonam
13. Destroys Claude Sonnet 3.7 in benchmarks
[Quoted tweet]
Holy sh*t
Google Gemini 2.5 Flash dropped.
It destroyed Claude Sonnet 3.7 (64k Extended Thinking) in benchmarks
20x cheaper on input
25x cheaper on output
~4.2x cheaper on output with reasoning
17/20
@CodeByPoonam
Gemini 2.5 Flash is now available on Gemini App, AI Studio, and API
Gemini: Gemini
AI Studio: Sign in - Google Accounts
18/20
@CodeByPoonam
Thanks for reading!
If you liked this post, check out my AI updates and tutorials Newsletter.
Join 35000+ readers in the AI Toast Community for free: AI Toast
19/20
@CodeByPoonam
Don't forget to bookmark for later.
If you enjoyed reading this post, please support it with like/repost of the post below
[Quoted tweet]
Google just dropped Gemini 2.5 Flash, and people are going crazy over it.
SPOILER: Claude is now falling behind.
13 wild examples so far (Don't miss the 5th one)
20/20
@ricathrs
Gemini 2.5 Flash sounds like a game changer!
To post tweets in this format, more info here: https://www.thecoli.com/threads/tips-and-tricks-for-posting-the-coli-megathread.984734/post-52211196
@CodeByPoonam
Google just dropped Gemini 2.5 Flash, and people are going crazy over it.
SPOILER: Claude is now falling behind.
13 wild examples so far (Don't miss the 5th one)
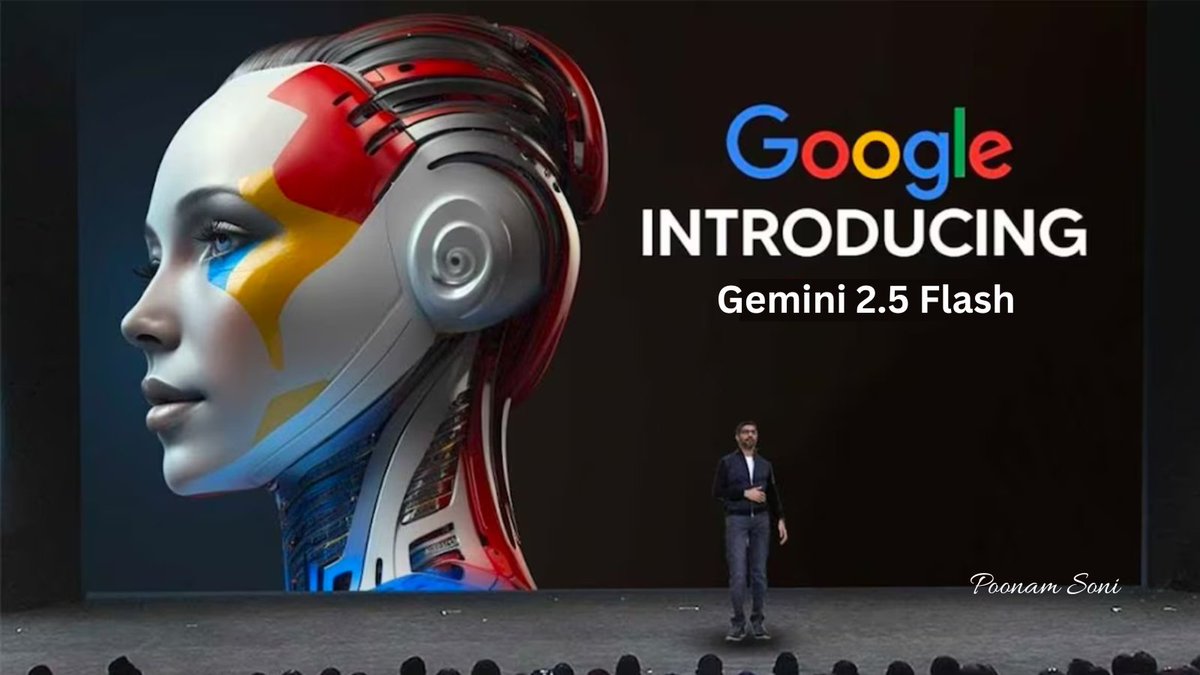
2/20
@CodeByPoonam
1. Tron-style game
[Quoted tweet]
Gemini 2.5 Flash Thinking 24k
Prompt: "Create Design a visually striking Tron-style game in a single HTML file, where AI-controlled light cycles compete in fast-paced, strategic battles against each other"
https://video.twimg.com/amplify_video/1912953001712447488/vid/avc1/1920x1080/-IoE5vICEJ3TqYS_.mp4
3/20
@CodeByPoonam
2. Gemini 2.5 Flash vs ChatGPT o3
[Quoted tweet]
I tested Gemini 2.5 Flash vs ChatGPT o3
Which one did better?
https://video.twimg.com/amplify_video/1913198527746129920/vid/avc1/1280x720/InEUUE-tUG1QljHE.mp4
4/20
@CodeByPoonam
3. Galton Board Test
[Quoted tweet]
Gemini 2.5 Flash demolishes my Galton Board test, I could not get 4omini, 4o mini high, or 03 to produce this. I found that Gemini 2.5 Flash understands my intents almost instantly, code produced is tight and neat. The prompt is a merging of various steps. It took me 5 steps to achieve this in Gemini 2.5 Flash, I gave up on OpenAI models after about half an hour. My iterations are obviously not exact. But people can test with this one prompt for more objective comparison.
Please try this prompt on your end to confirm:
--------------------------------------------------
Create a self-contained HTML file for a Galton board simulation using client-side JavaScript and a 2D physics engine (like Matter.js, included via CDN). The simulation should be rendered on an HTML5 canvas and meet the following criteria: 1. **Single File:** All necessary HTML, CSS, and JavaScript code must be within this single `.html` file. 2. **Canvas Size:** The overall simulation area (canvas) should be reasonably sized to fit on a standard screen without requiring extensive scrolling or zooming (e.g., around 500x700 pixels). 3. **Physics:** Utilize a 2D rigid body physics engine for realistic ball-peg and ball-wall interactions. 4. **Obstacles (Pegs):** Create static, circular pegs arranged in full-width horizontal rows extending across the usable width of the board (not just a triangle). The pegs should be small enough and spaced appropriately for balls to navigate and bounce between them. 5. **Containment:** * Include static, sufficiently thick side walls and a ground at the bottom to contain the balls within the board. * Implement *physical* static dividers between the collection bins at the bottom. These dividers must be thick enough to prevent balls from passing through them, ensuring accurate accumulation in each bin. 6. **Ball Dropping:** Balls should be dropped from a controlled, narrow area near the horizontal center at the top of the board to ensure they enter the peg field consistently. 7. **Bins:** The collection area at the bottom should be divided into distinct bins by the physical dividers. The height of the bins should be sufficient to clearly visualize the accumulation of balls. 8. **Visualization:** Use a high-contrast color scheme to clearly distinguish between elements. Specifically, use yellow for the structural elements (walls, top guides, physical bin dividers, ground), a contrasting color (like red) for the pegs, and a highly contrasting color (like dark grey or black) for the balls. 9. **Demonstration:** The simulation should visually demonstrate the formation of the normal (or binomial) distribution as multiple balls fall through the pegs and collect in the bins. Ensure the physics parameters (restitution, friction, density) and ball drop rate are tuned for a smooth and clear demonstration of the distribution.
#OpenAI @sama @gdb @ai_for_success @aidan_mclau
https://video.twimg.com/amplify_video/1912972357947334656/vid/avc1/776x586/dX9gd5al-B2qxt6t.mp4
5/20
@CodeByPoonam
Get the latest updates on AI insights and Tutorials.
Join "AI Toast" the community of 35,000 readers for FREE.
Read latest edition here:
AI Toast
6/20
@CodeByPoonam
4. Gemini 2.5 Flash is blazing fast
[Quoted tweet]
First test on gemini 2.5 flash on my phone. This model is blazing fast and it one shotted this, mobile friendly, animation. The code looks pretty clean too. Good vibes so far.
https://video.twimg.com/ext_tw_video/1912946801809772545/pu/vid/avc1/590x1278/nXzNRDKeHXL7JAyb.mp4
7/20
@CodeByPoonam
5. Cloth Simulation
[Quoted tweet]
Prompt: Create a cloth simulation using Verlet integration in a single HTML file (Canvas or Three.js). Include wind, gravity, and drag. Let users interact by dragging points. Cloth should bend and move realistically.
Model: Gemini flash 2.5
https://video.twimg.com/ext_tw_video/1913047505815953408/pu/vid/avc1/590x1278/WSwRATTymRpNQRy2.mp4
8/20
@CodeByPoonam
6. Image segmentation masks on command
[Quoted tweet]
Gemini 2.5 Pro and Flash now have the ability to return image segmentation masks on command, as base64 encoded PNGs embedded in JSON strings
I vibe coded this interactive tool for exploring this new capability - it costs a fraction of a cent per image
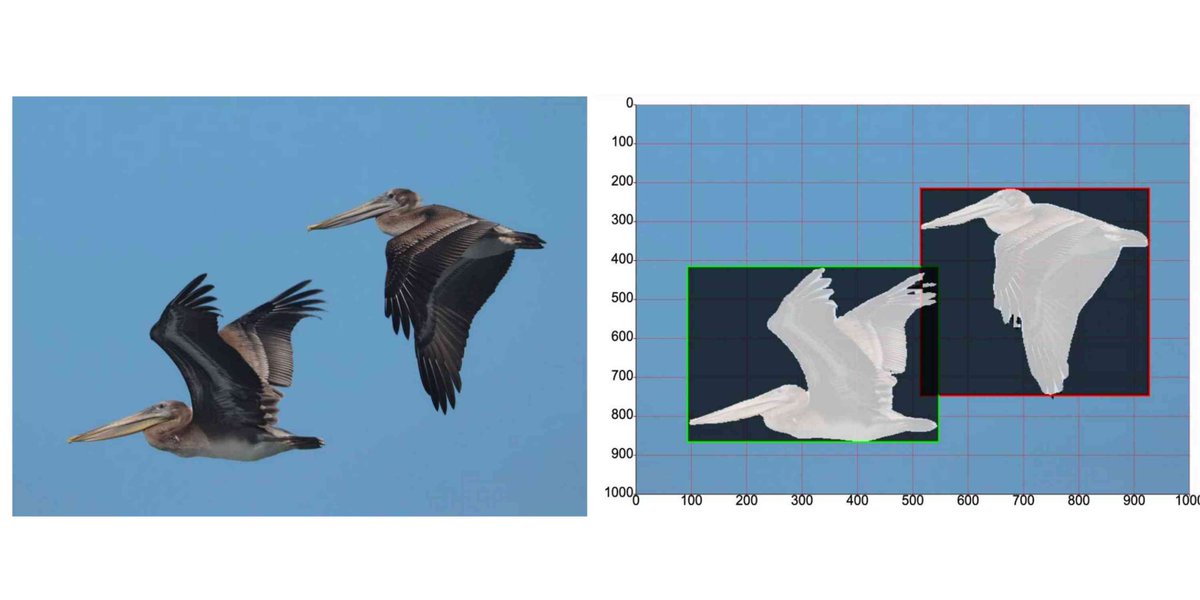
9/20
@CodeByPoonam
7. MCP AI Agent
[Quoted tweet]
I built an MCP AI Agent using Gemini Flash 2.5 with access to AirBnB and Google Maps in just 30 lines of Python Code.
100% Opensource Code.
https://video.twimg.com/amplify_video/1913056645271429120/vid/avc1/1212x720/AfIwfVNsUWTKRlmu.mp4
10/20
@CodeByPoonam
8. Gemini 2.5 Flash is very cheap and super intelligent model.
[Quoted tweet]
Gemini 2.5 Flash Preview is an amazing model. Google is literally winning. No stopping them now, this is not normal.
Gemini 2.5 Flash is very cheap and super intelligent model. Intelligence too cheap to meter this is what it means.
Thank you, Google.
https://video.twimg.com/amplify_video/1912957952824356864/vid/avc1/1920x1080/20ckf4zJ7d1F-Y5P.mp4
11/20
@CodeByPoonam
8. Classic Snakes and Ladders
[Quoted tweet]
A lot of people make a snake game when trying out new models. I went with the classic Snakes and Ladders instead — built it using @GoogleDeepMind latest Gemini Flash 2.5 and it nails it. Look at how it follows the stairs and snakes so smoothly.
Still a WIP and don’t mind the extra dot on the die though


It is said that this game started in ancient India where it was called Moksha Patam. Every move was a little life lesson where ladders were virtues while snakes were vices.
https://video.twimg.com/amplify_video/1913417180785360896/vid/avc1/1920x1080/nSy-2R-lP8ZiOk13.mp4
12/20
@CodeByPoonam
9. Create Simulation
[Quoted tweet]
AGI is achieved by Google's Gemini 2.5 Flash Preview
Seriously this is the best simulation i've ever created of how AI models work
https://video.twimg.com/amplify_video/1912963072299311104/vid/avc1/1464x720/5TOp8tU-RVWCulcR.mp4
13/20
@CodeByPoonam
10. A Block breaker
[Quoted tweet]

- 思考プロセスをオン/オフ可能
- 推論能力を大幅向上、高速性とコスト効率を維持
- 思考予算設定で品質・コスト・レイテンシーを自在に最適化できるハイブリッド思考AI
試しにブロック崩しを作成
注目ポイントを7点まとめました

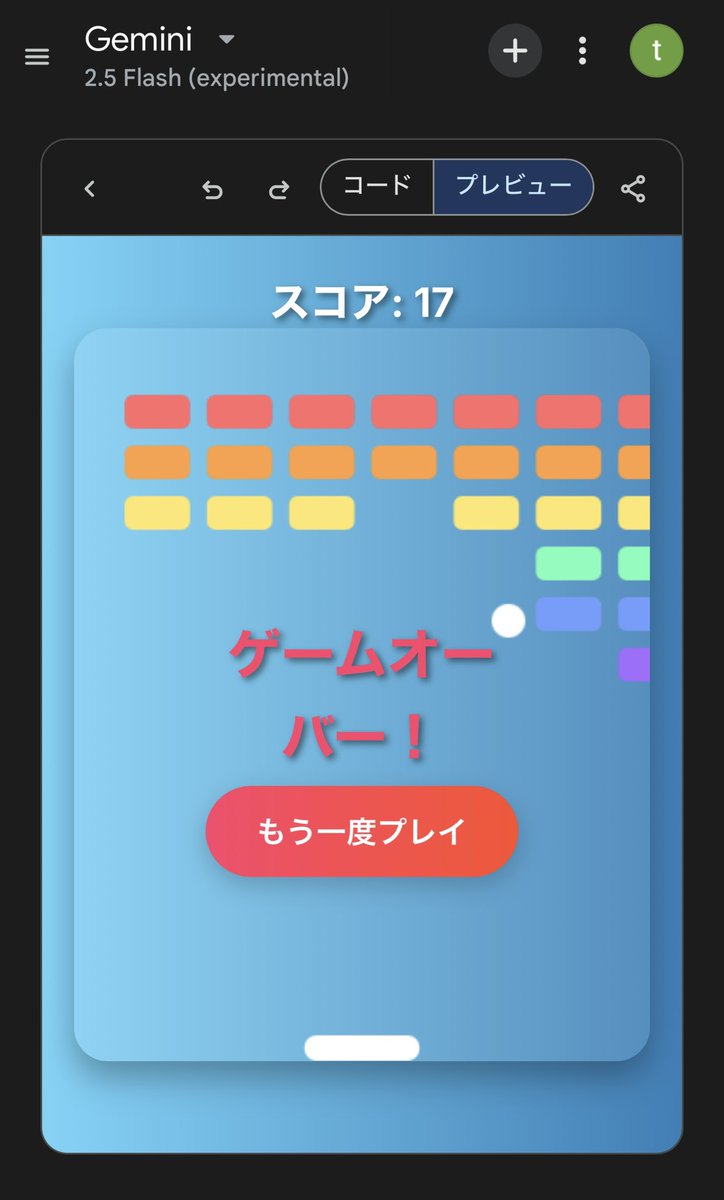
14/20
@CodeByPoonam
11. A dreamy low-poly floating island scene
[Quoted tweet]
Gemini 2.5 Pro

Prompt: "Create a dreamy low-poly floating island scene with dynamic lighting and gentle animations, in a single HTML file."
Gemini 2.5 Pro (left), Gemini 2.5 Flash (right)
https://video.twimg.com/amplify_video/1912964537277452288/vid/avc1/1920x1080/9egTWI8Uw7s6dkfe.mp4
15/20
@CodeByPoonam
12. Generate an SVG of a pelican riding a bicycle
[Quoted tweet]
I upgraded llm-gemini to support the new model, including a "-o thinking_budget X" option for setting the thinking budget
llm install -U llm-gemini
llm -m gemini-2.5-flash-preview-04-17 'Generate an SVG of a pelican riding a bicycle' -o thinking_budget 24576
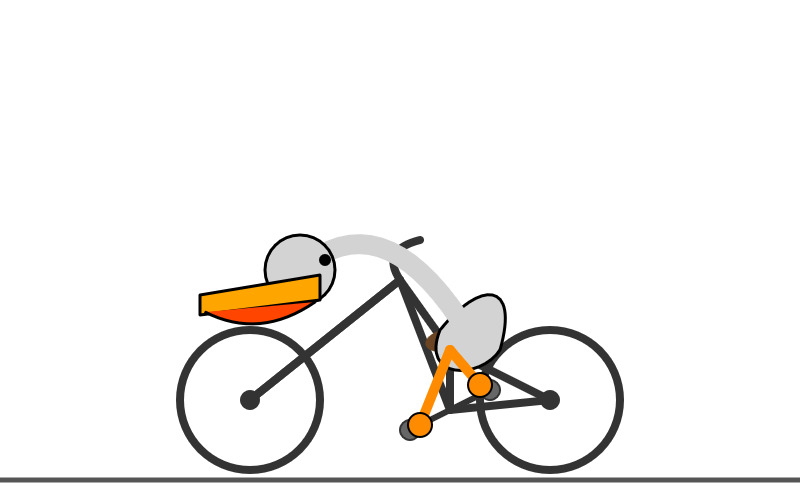
16/20
@CodeByPoonam
13. Destroys Claude Sonnet 3.7 in benchmarks
[Quoted tweet]
Holy sh*t
Google Gemini 2.5 Flash dropped.
It destroyed Claude Sonnet 3.7 (64k Extended Thinking) in benchmarks

20x cheaper on input
25x cheaper on output
~4.2x cheaper on output with reasoning
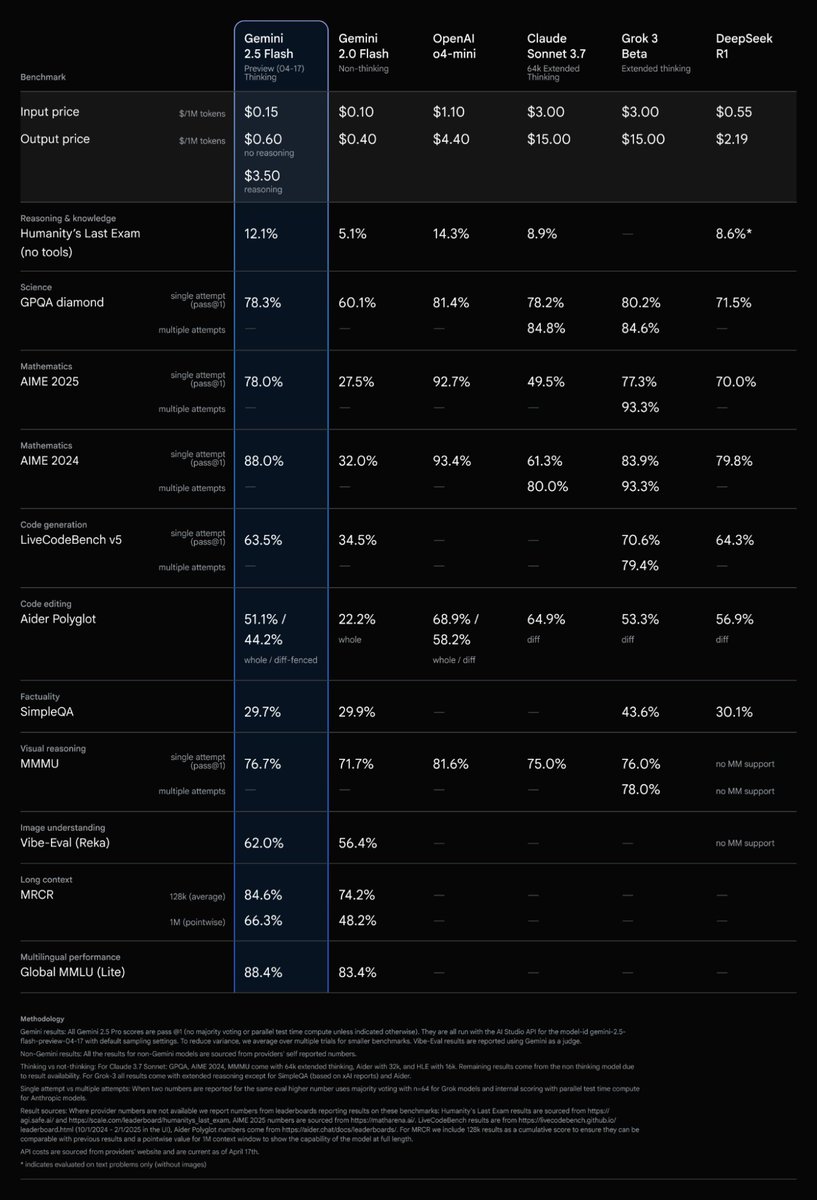
17/20
@CodeByPoonam
Gemini 2.5 Flash is now available on Gemini App, AI Studio, and API
Gemini: Gemini
AI Studio: Sign in - Google Accounts
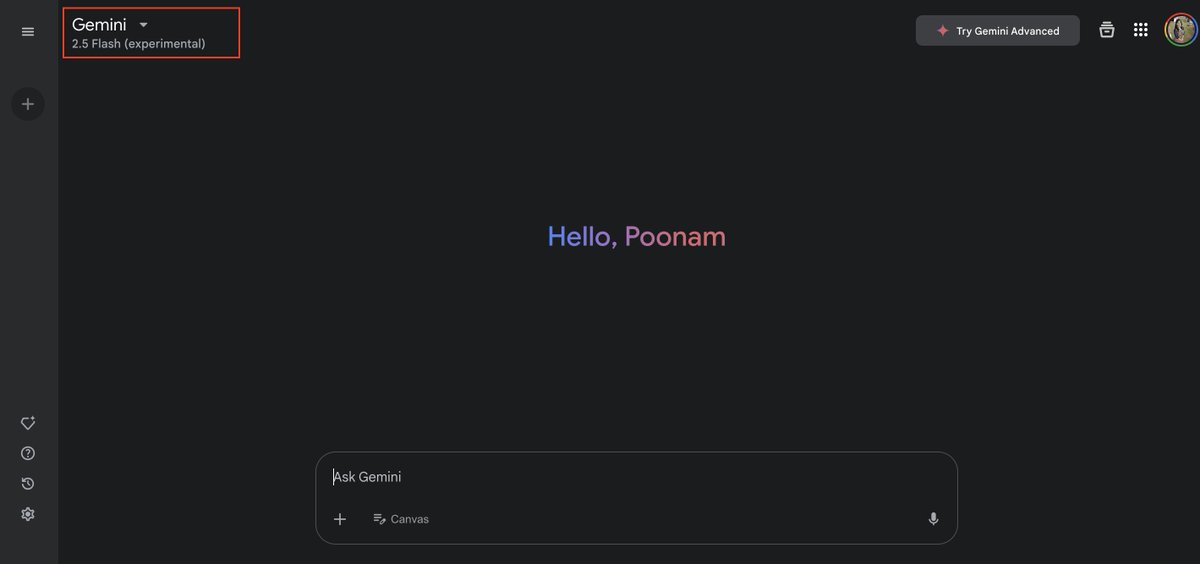
18/20
@CodeByPoonam
Thanks for reading!
If you liked this post, check out my AI updates and tutorials Newsletter.
Join 35000+ readers in the AI Toast Community for free: AI Toast
19/20
@CodeByPoonam
Don't forget to bookmark for later.
If you enjoyed reading this post, please support it with like/repost of the post below

[Quoted tweet]
Google just dropped Gemini 2.5 Flash, and people are going crazy over it.
SPOILER: Claude is now falling behind.
13 wild examples so far (Don't miss the 5th one)
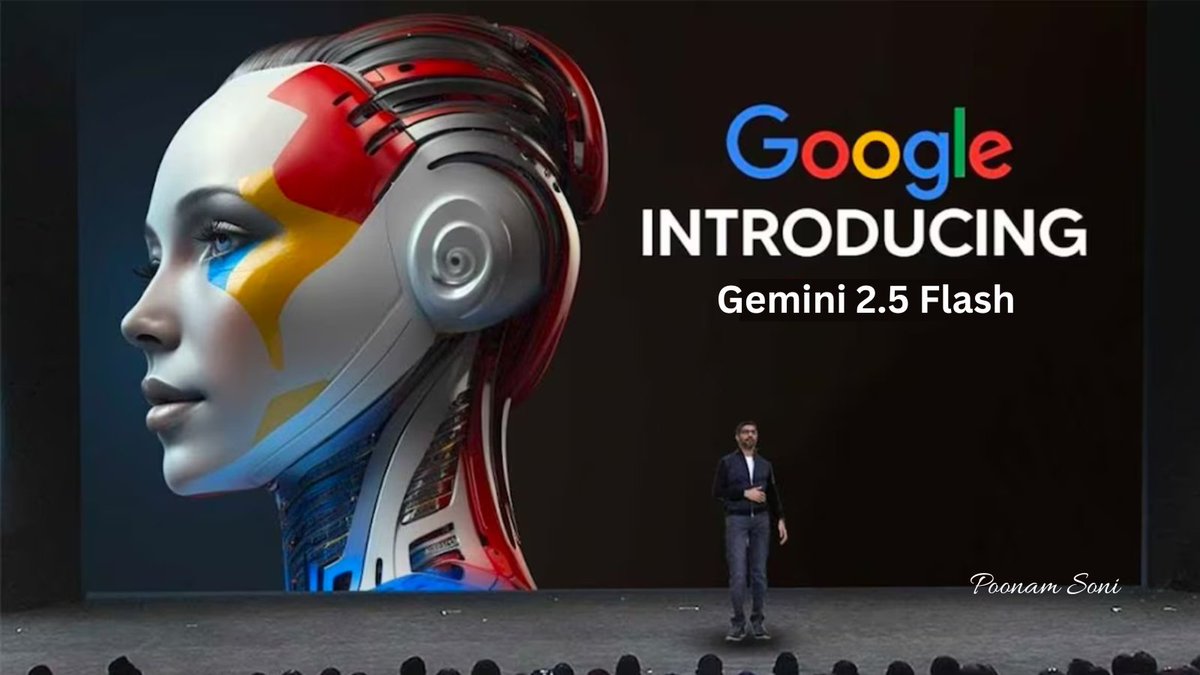
20/20
@ricathrs
Gemini 2.5 Flash sounds like a game changer!

To post tweets in this format, more info here: https://www.thecoli.com/threads/tips-and-tricks-for-posting-the-coli-megathread.984734/post-52211196