3 hours ago - Technology
New AI polyglot launched to help fill massive language gap in field
- Alison Snyder
, author of
Axios Science
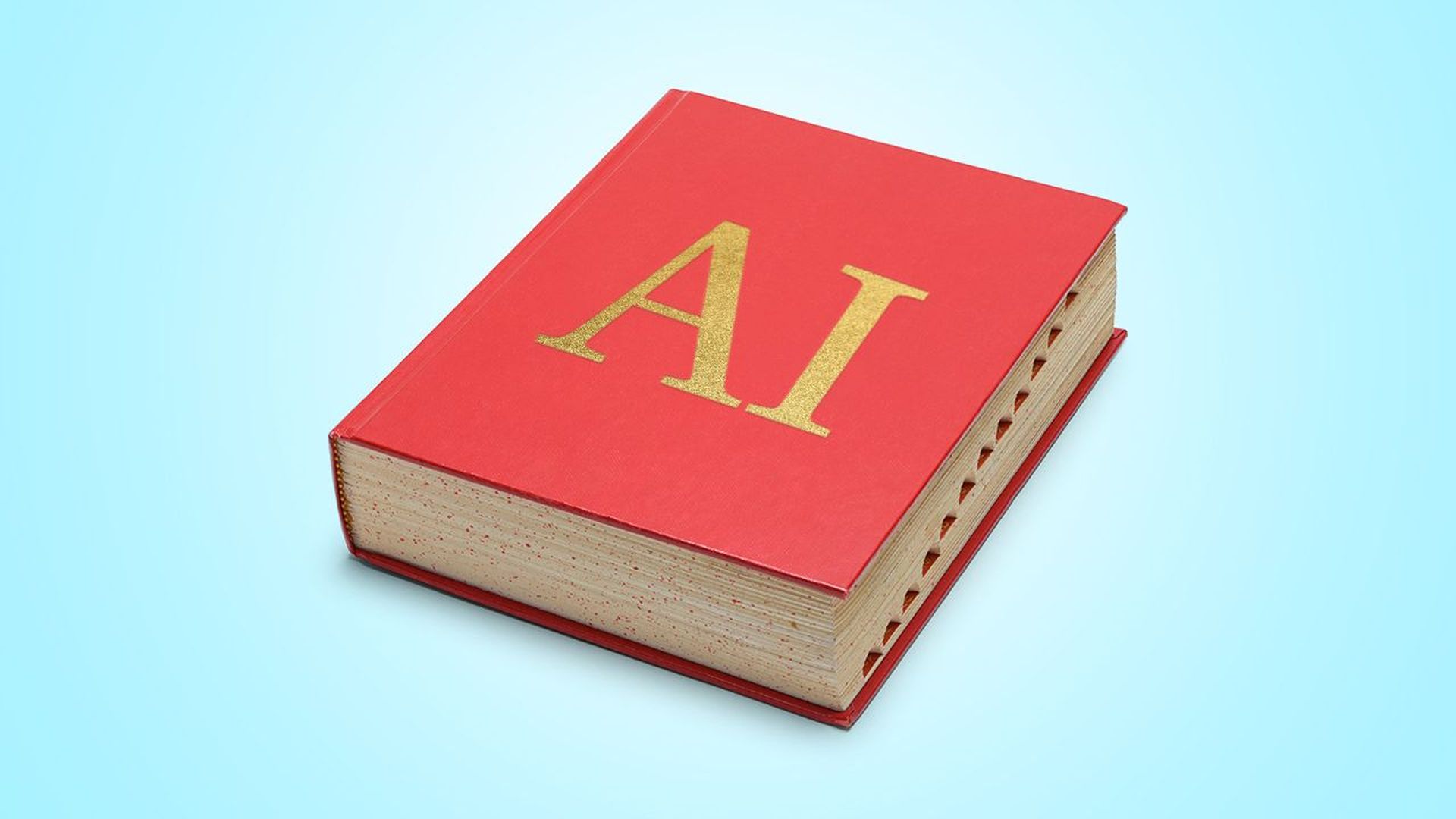
Illustration: Natalie Peeples/Axios
A new open-source generative AI model can follow instructions in more than 100 languages.
Why it matters: Most models that power today's generative AI tools are trained on data in English and Chinese, leaving a massive gap of thousands of languages — and potentially limiting access to the powerful technology for billions of people.
Details: Cohere for AI, the nonprofit AI research lab at Cohere, on Tuesday released its open-source multilingual large language model (LLM) called Aya.
- It covers more than twice as many languages as other existing open-source models and is the result of a year-long project involving 3,000 researchers in 119 countries.
- But they first had to create a high-quality dataset of prompt and completion pairs (the inputs and outputs of the model) in different languages, which is also being released.
- Their data sources include machine translations of several existing datasets into more than 100 languages, roughly half of which are considered underrepresented — or unrepresented — in existing text datasets, including Azerbaijani, Bemba, Welsh and Gujarati.
- They also created a dataset that tries to capture cultural nuances and meaningful information by having about 204,000 prompts and completions curated and annotated by fluent speakers in 67 languages.
- The team reports Aya outperforms other existing open-source multilingual models when evaluated by humans or using GPT-4.
The impact: "Aya is a massive leap forward — but the biggest goal is all these collaboration networks spur bottom up collaborations," says Sara Hooker, who leads Cohere for AI.
- The team envisions Aya being used for language research and to preserve and represent languages and cultures at risk of being left out of AI advances.
The big picture: Aya is one of a handful of open-source multilingual models, including BLOOM, which can generate text in 46 languages, a bilingual Arabic-English LLM called Jais, and a model in development by the Masakhane Foundation that covers African languages.
What to watch: "In some ways this is a bandaid for the wider issue with multilingual [LLMs]," Hooker says. "An important bandaid but the issues still persist."
- Those include figuring out why LLMs can't seem to be adopted to languages they didn't see in pre-training and exploring how best to evaluate these models.
- "We used to think of a model as something that fulfills a very specific, finite and controlled notion of a request," like a definitive answer about whether a response is correct, Hooker says. "And now we want models to do everything, and be universal and fluid."
- 'We can’t have everything so we will have to chose what we want it to be."

Cohere For AI Launches Aya, an LLM Covering More Than 100 Languages
More than double the number of languages covered by previous open-source AI models to increase coverage for underrepresented communities Today, the research team at Cohere For AI (C4AI), Cohere’s non-profit research lab, are excited to announce a new state-of-the-art, open-source, massively...

FEB 13, 2024
Cohere For AI Launches Aya, an LLM Covering More Than 100 Languages
More than double the number of languages covered by previous open-source AI models to increase coverage for underrepresented communitiesToday, the research team at Cohere For AI (C4AI), Cohere’s non-profit research lab, are excited to announce a new state-of-the-art, open-source, massively multilingual, generative large language research model (LLM) covering 101 different languages — more than double the number of languages covered by existing open-source models. Aya helps researchers unlock the powerful potential of LLMs for dozens of languages and cultures largely ignored by most advanced models on the market today.
We are open-sourcing both the Aya model, as well as the largest multilingual instruction fine-tuned dataset to-date with a size of 513 million covering 114 languages. This data collection includes rare annotations from native and fluent speakers all around the world, ensuring that AI technology can effectively serve a broad global audience that have had limited access to-date.
Closes the Gap in Languages and Cultural Relevance
Aya is part of a paradigm shift in how the ML community approaches massively multilingual AI research, representing not just technical progress, but also a change in how, where, and by whom research is done.As LLMs, and AI generally, have changed the global technological landscape, many communities across the world have been left unsupported due to the language limitations of existing models. This gap hinders the applicability and usefulness of generative AI for a global audience, and it has the potential to further widen existing disparities that already exist from previous waves of technological development. By focusing primarily on English and one or two dozen other languages as training resources, most models tend to reflect inherent cultural bias.
We started the Aya project to address this gap, bringing together over 3,000 independent researchers from 119 countries.
Figure: Geographical distribution of Aya collaborators
Significantly Outperforms Existing Open-Source Multilingual Models
The research team behind Aya was able to substantially improve performance for underserved languages, demonstrating superior capabilities in complex tasks, such as natural language understanding, summarization, and translation, across a wide linguistic spectrum.We benchmark Aya model performance against available, open-source, massively multilingual models. It surpasses the best open-source models, such as mT0 and Bloomz, on benchmark tests by a wide margin. Aya consistently scored 75% in human evaluations against other leading open-source models, and 80-90% across the board in simulated win rates.
Aya also expands coverage to more than 50 previously unserved languages, including Somali, Uzbek, and more. While proprietary models do an excellent job serving a range of the most commonly spoken languages in the world, Aya helps to provide researchers with an unprecedented open-source model for dozens of underrepresented languages.

Figure: Head-to-head comparison of preferred model responses
Trained on the Most Extensive Multilingual Dataset to Date
We are releasing the Aya Collection consisting of 513 million prompts and completions covering 114 languages. This massive collection was created by fluent speakers around the world creating templates for selected datasets and augmenting a carefully curated list of datasets. It also includes the Aya Dataset which is the most extensive human-annotated, multilingual, instruction fine-tuning dataset to date. It contains approximately 204,000 rare human curated annotations by fluent speakers in 67 languages, ensuring robust and diverse linguistic coverage. This offers a large-scale repository of high-quality language data for developers and researchers.Many languages in this collection had no representation in instruction-style datasets before. The fully permissive and open-sourced dataset includes a wide spectrum of language examples, encompassing a variety of dialects and original contributions that authentically reflect organic, natural, and informal language use. This makes it an invaluable resource for multifaceted language research and linguistic preservation efforts.