1/11
@TheTuringPost
The freshest AI/ML researches of the week, part 1
New AI Model Gemini Experimental 1114 Debuts On Google AI Studio
CamemBERT 2.0
Qwen2.5-Coder Series
Llava-o1
LLMs Can Self-Improve In Long-Context Reasoning
Direct Preference Optimization Using Sparse Feature-Level Constraints
Cut Your Losses In Large-Vocabulary Language Models
SPARSING LAW

2/11
@TheTuringPost
1. New AI Model Gemini Experimental 1114 Debuts On Google AI Studio
Demonstrates strong reasoning skills with a 32k context window, outperforming competitors on benchmarks, despite slower problem-solving speed.
[Quoted tweet]
gemini-exp-1114…. available in Google AI Studio right now, enjoy : )
aistudio.google.com
3/11
@TheTuringPost
2. CamemBERT 2.0: A Smarter French Language Model Aged to Perfection
Tackles concept drift in French NLP with improved tokenization, excelling in QA and domain-specific tasks like biomedical NER.
[2411.08868] CamemBERT 2.0: A Smarter French Language Model Aged to Perfection
Open models: almanach (ALMAnaCH (Inria))
4/11
@TheTuringPost
3. Qwen2.5-Coder Series: Powerful, Diverse, Practical
Excels in coding and multi-language repair tasks, rivaling GPT-4o in 40+ programming languages with open innovation for developers.
Qwen2.5-Coder Series: Powerful, Diverse, Practical.
5/11
@TheTuringPost
4. Llava-o1: Let Vision Language Models Reason Step-By-Step
Enhances multimodal reasoning through structured, multi-stage processes, achieving superior benchmark performance.
[2411.10440] LLaVA-o1: Let Vision Language Models Reason Step-by-Step
[Quoted tweet]
LLaVA-o1 is a smarter Vision-Language Model (VLM) that thinks step-by-step.
Instead of jumping to answers, it divides reasoning into 4 clear stages and uses stage-level beam search to generate multiple answers and select the best one for each stage.
Here's how is works:
6/11
@TheTuringPost
5. Large Language Models Can Self-Improve In Long-Context Reasoning
Uses self-improvement via ranking model outputs (SeaLong approach), improving performance in long-context reasoning tasks without external datasets.
[2411.08147] Large Language Models Can Self-Improve in Long-context Reasoning
GitHub: GitHub - SihengLi99/SEALONG: Large Language Models Can Self-Improve in Long-context Reasoning
7/11
@TheTuringPost
6. Direct Preference Optimization Using Sparse Feature-Level Constraints
Introduces method that improves alignment efficiency in LLMs and reduces computational overhead, using sparse autoencoders and feature constraints.
[2411.07618] Direct Preference Optimization Using Sparse Feature-Level Constraints
8/11
@TheTuringPost
7. Cut Your Losses In Large-Vocabulary Language Models
Proposes Cut Cross-Entropy (CCE) method that reduces memory use for large-scale training, enabling up to 10x larger batch sizes without sacrificing performance.
[2411.09009] Cut Your Losses in Large-Vocabulary Language Models
GitHub: GitHub - apple/ml-cross-entropy
9/11
@TheTuringPost
8. SPARSING LAW: Towards Large Language Models With Greater Activation Sparsity
Explores neuron sparsity in LLMs to enhance efficiency while preserving interpretability.
[2411.02335] Sparsing Law: Towards Large Language Models with Greater Activation Sparsity
GitHub: GitHub - thunlp/SparsingLaw: The open-source materials for paper "Sparsing Law: Towards Large Language Models with Greater Activation Sparsity".
10/11
@TheTuringPost
9. Find a complete list of the latest research papers in our free weekly digest:
#76: Rethinking Scaling Laws (when plateau is actually a fork)
11/11
@TheTuringPost
10. Follow @TheTuringPost for more.
Like/repost the 1st post to support our work
Also, elevate your AI game with our free newsletter ↓
Turing Post
[Quoted tweet]
The freshest AI/ML researches of the week, part 1
New AI Model Gemini Experimental 1114 Debuts On Google AI Studio
CamemBERT 2.0
Qwen2.5-Coder Series
Llava-o1
LLMs Can Self-Improve In Long-Context Reasoning
Direct Preference Optimization Using Sparse Feature-Level Constraints
Cut Your Losses In Large-Vocabulary Language Models
SPARSING LAW

To post tweets in this format, more info here: https://www.thecoli.com/threads/tips-and-tricks-for-posting-the-coli-megathread.984734/post-52211196
@TheTuringPost
The freshest AI/ML researches of the week, part 1









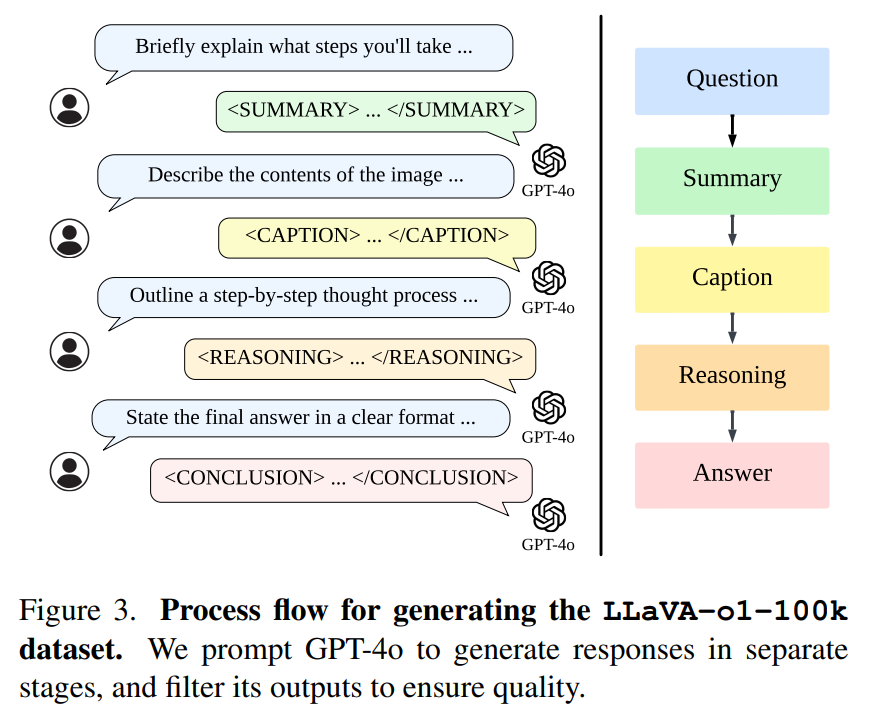
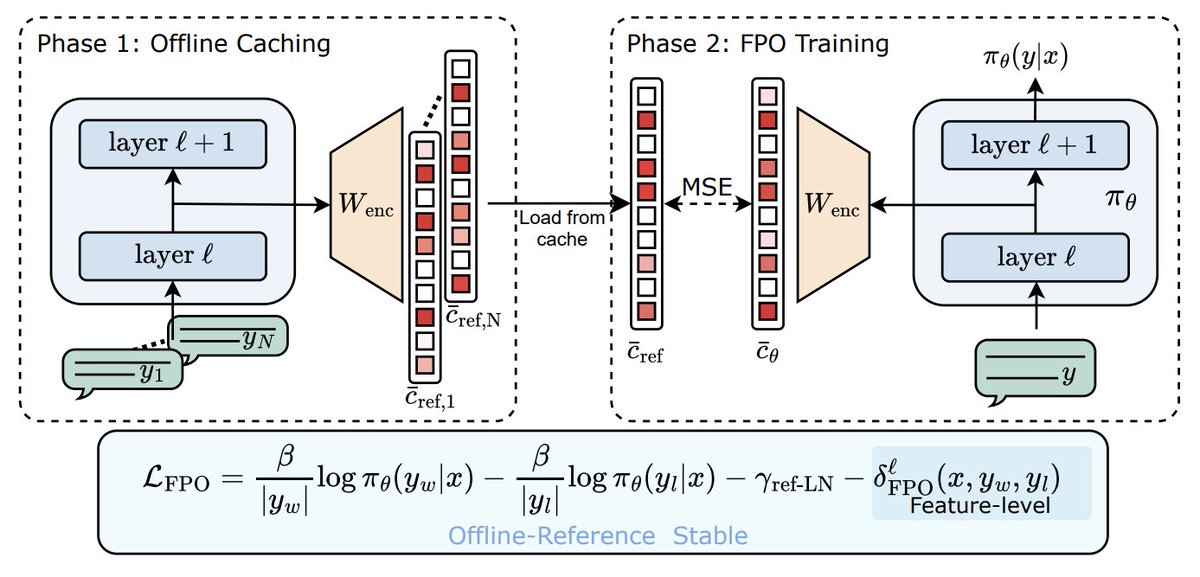
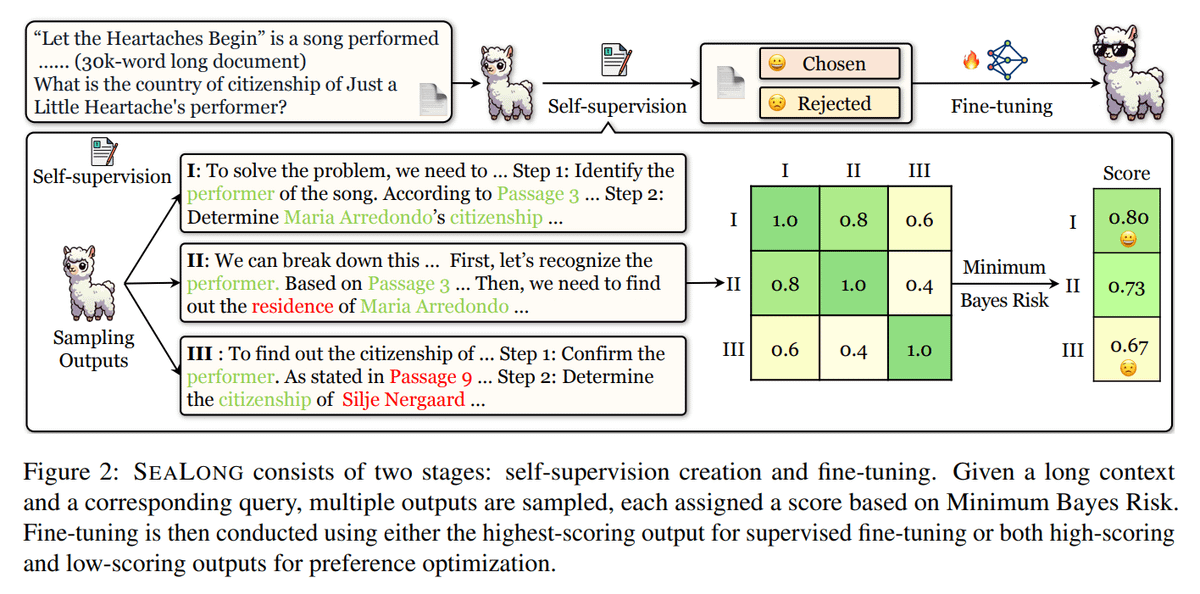
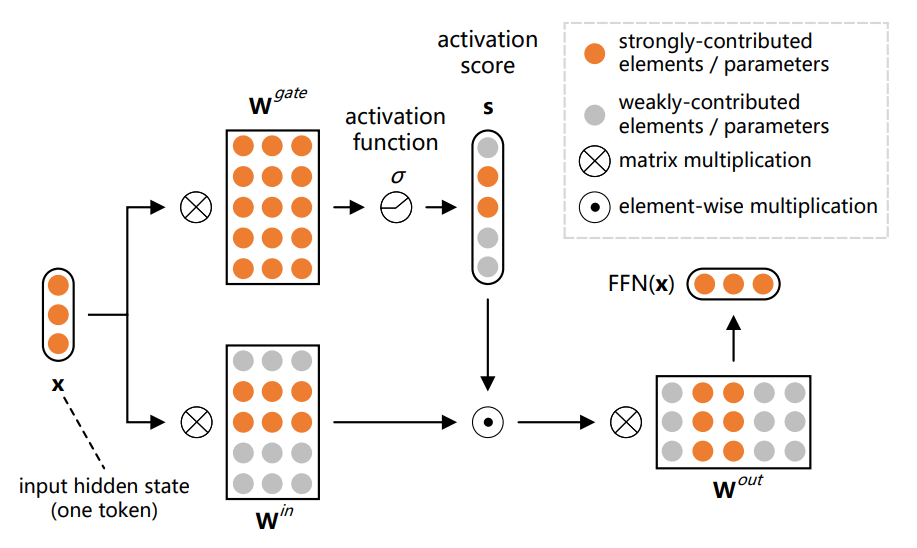
2/11
@TheTuringPost
1. New AI Model Gemini Experimental 1114 Debuts On Google AI Studio
Demonstrates strong reasoning skills with a 32k context window, outperforming competitors on benchmarks, despite slower problem-solving speed.
[Quoted tweet]
gemini-exp-1114…. available in Google AI Studio right now, enjoy : )
aistudio.google.com
3/11
@TheTuringPost
2. CamemBERT 2.0: A Smarter French Language Model Aged to Perfection
Tackles concept drift in French NLP with improved tokenization, excelling in QA and domain-specific tasks like biomedical NER.
[2411.08868] CamemBERT 2.0: A Smarter French Language Model Aged to Perfection
Open models: almanach (ALMAnaCH (Inria))
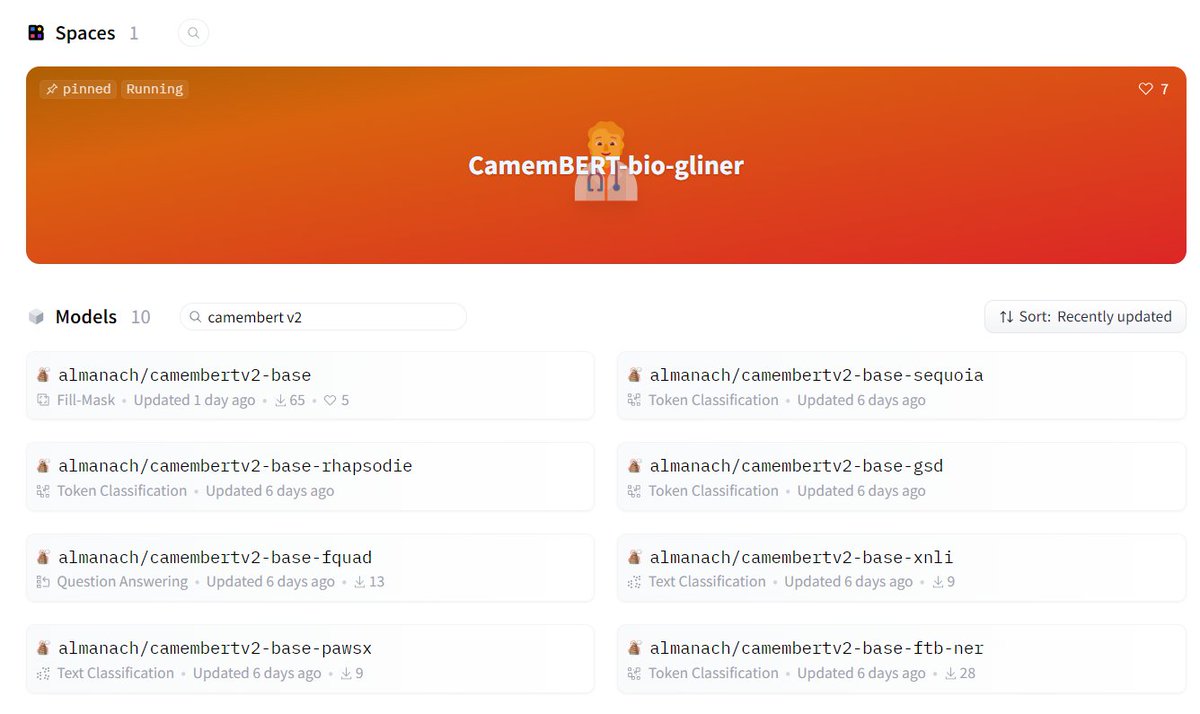
4/11
@TheTuringPost
3. Qwen2.5-Coder Series: Powerful, Diverse, Practical
Excels in coding and multi-language repair tasks, rivaling GPT-4o in 40+ programming languages with open innovation for developers.
Qwen2.5-Coder Series: Powerful, Diverse, Practical.
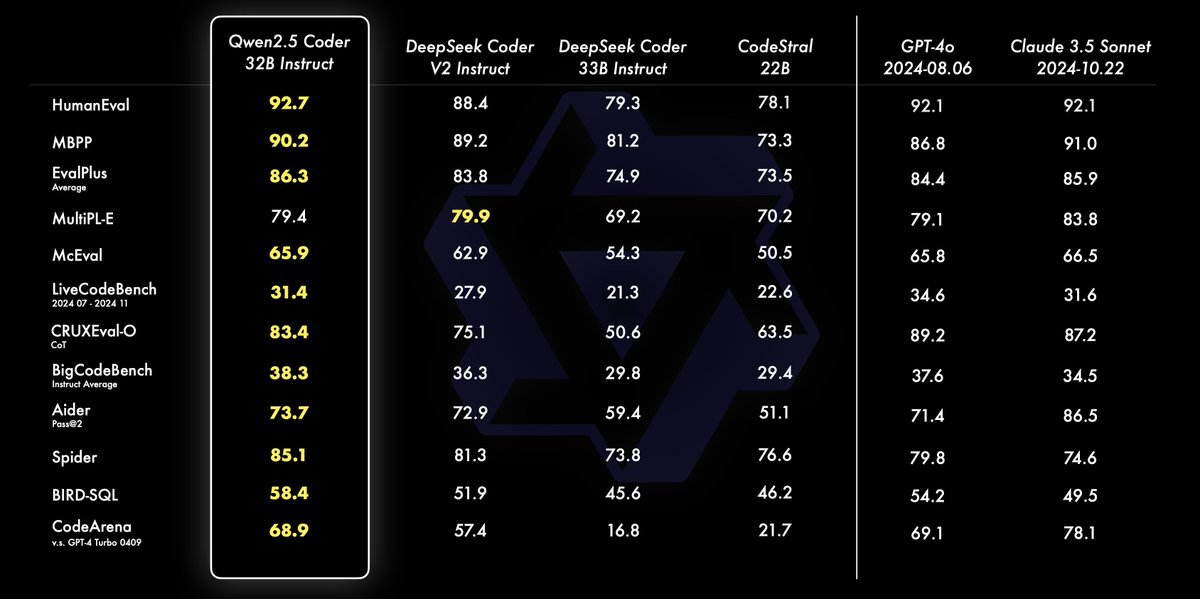
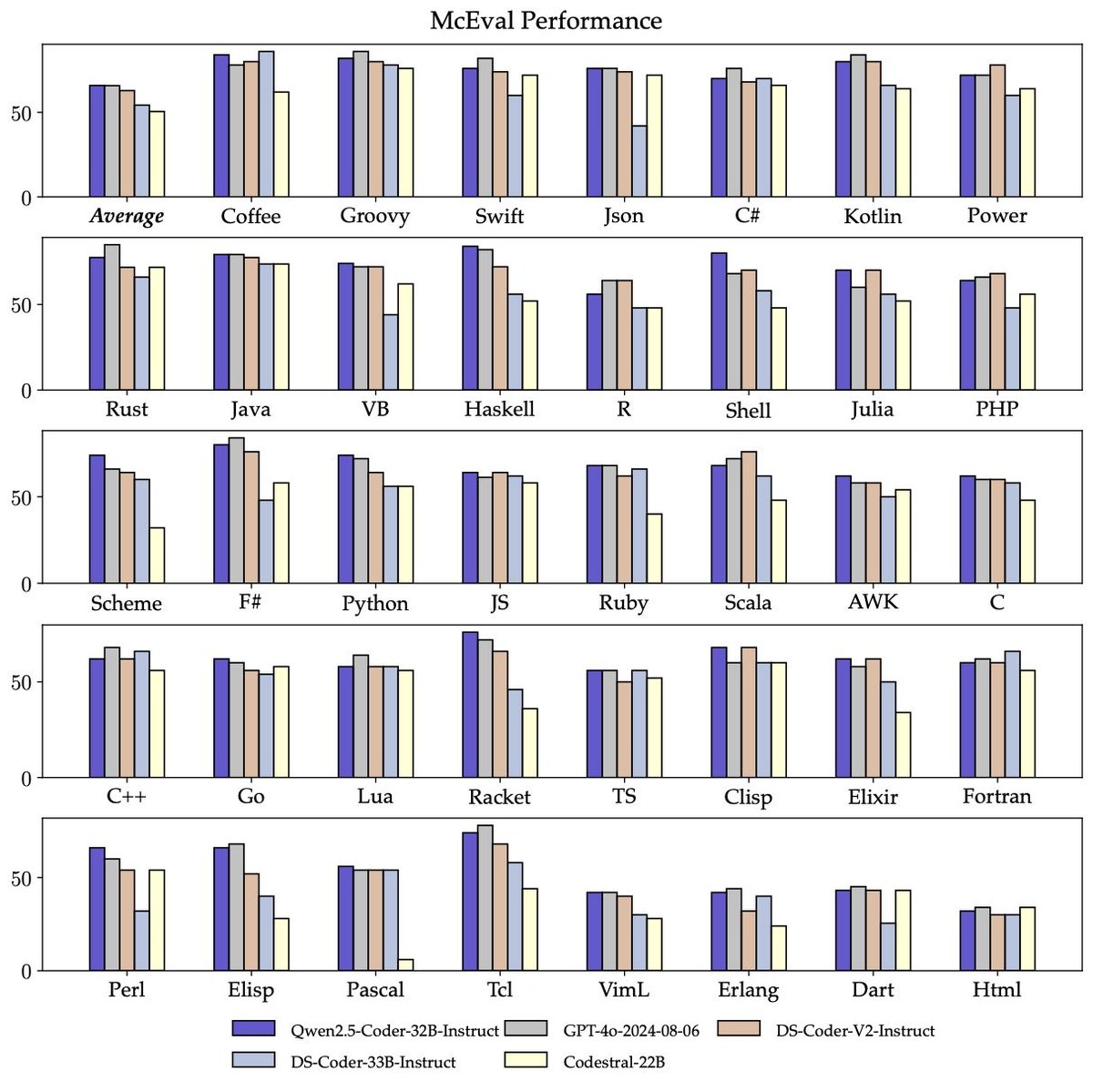
5/11
@TheTuringPost
4. Llava-o1: Let Vision Language Models Reason Step-By-Step
Enhances multimodal reasoning through structured, multi-stage processes, achieving superior benchmark performance.
[2411.10440] LLaVA-o1: Let Vision Language Models Reason Step-by-Step
[Quoted tweet]
LLaVA-o1 is a smarter Vision-Language Model (VLM) that thinks step-by-step.
Instead of jumping to answers, it divides reasoning into 4 clear stages and uses stage-level beam search to generate multiple answers and select the best one for each stage.
Here's how is works:
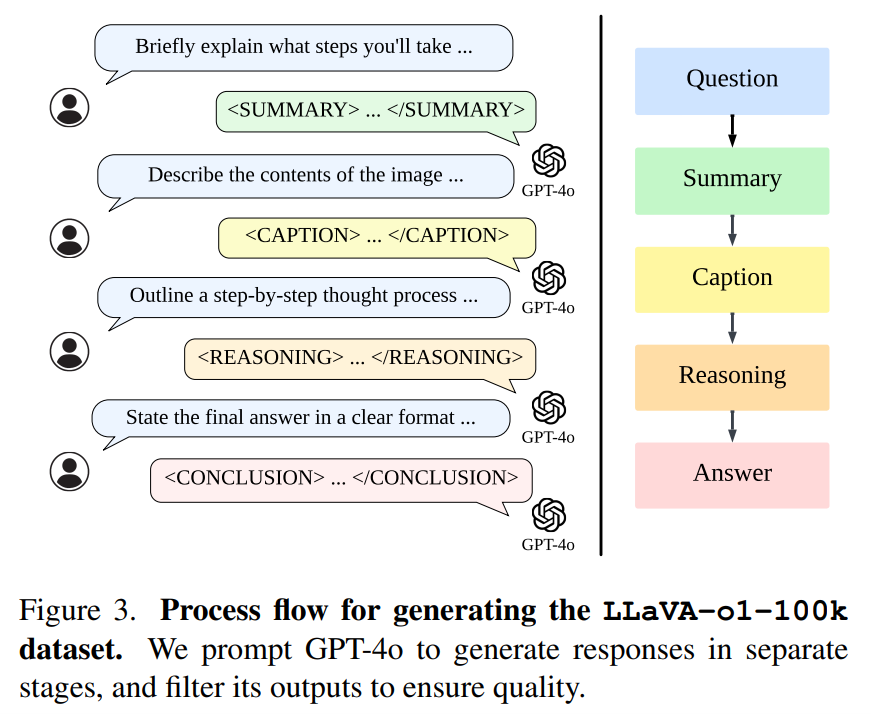
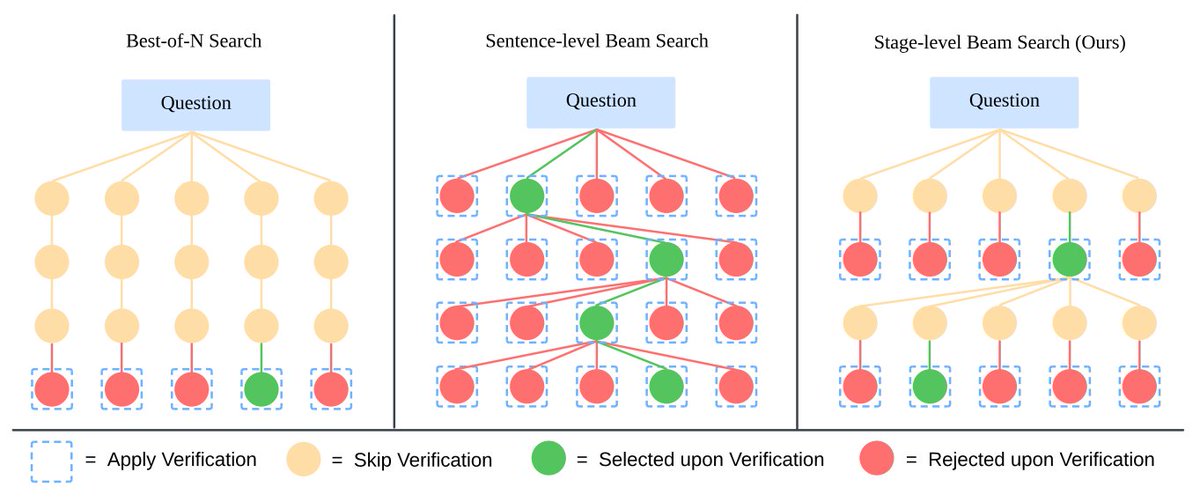
6/11
@TheTuringPost
5. Large Language Models Can Self-Improve In Long-Context Reasoning
Uses self-improvement via ranking model outputs (SeaLong approach), improving performance in long-context reasoning tasks without external datasets.
[2411.08147] Large Language Models Can Self-Improve in Long-context Reasoning
GitHub: GitHub - SihengLi99/SEALONG: Large Language Models Can Self-Improve in Long-context Reasoning
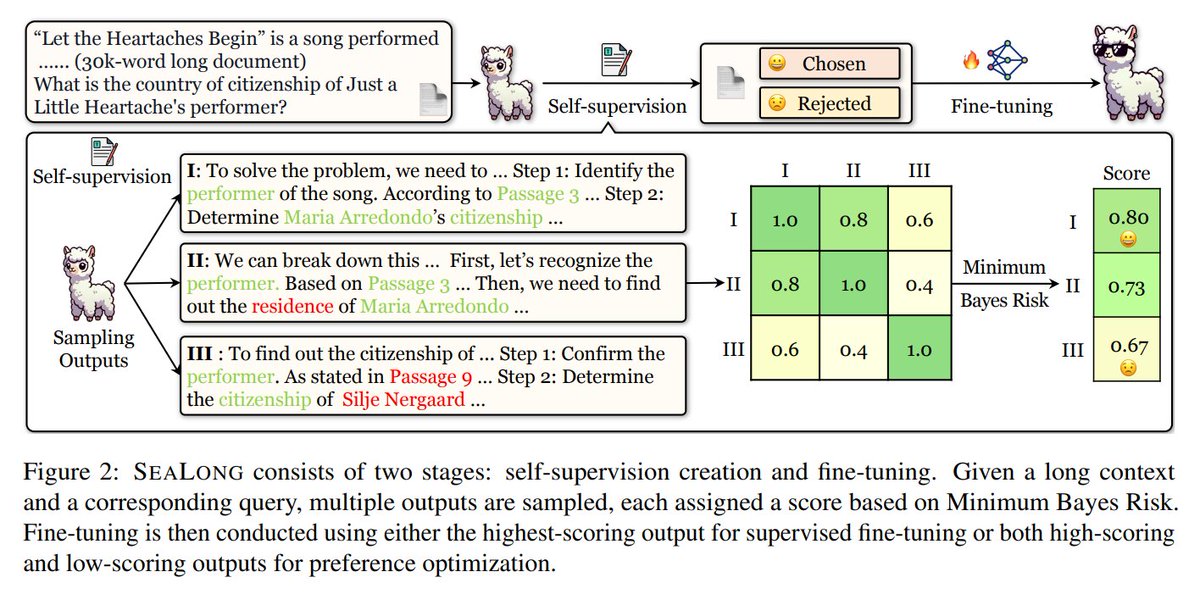
7/11
@TheTuringPost
6. Direct Preference Optimization Using Sparse Feature-Level Constraints
Introduces method that improves alignment efficiency in LLMs and reduces computational overhead, using sparse autoencoders and feature constraints.
[2411.07618] Direct Preference Optimization Using Sparse Feature-Level Constraints
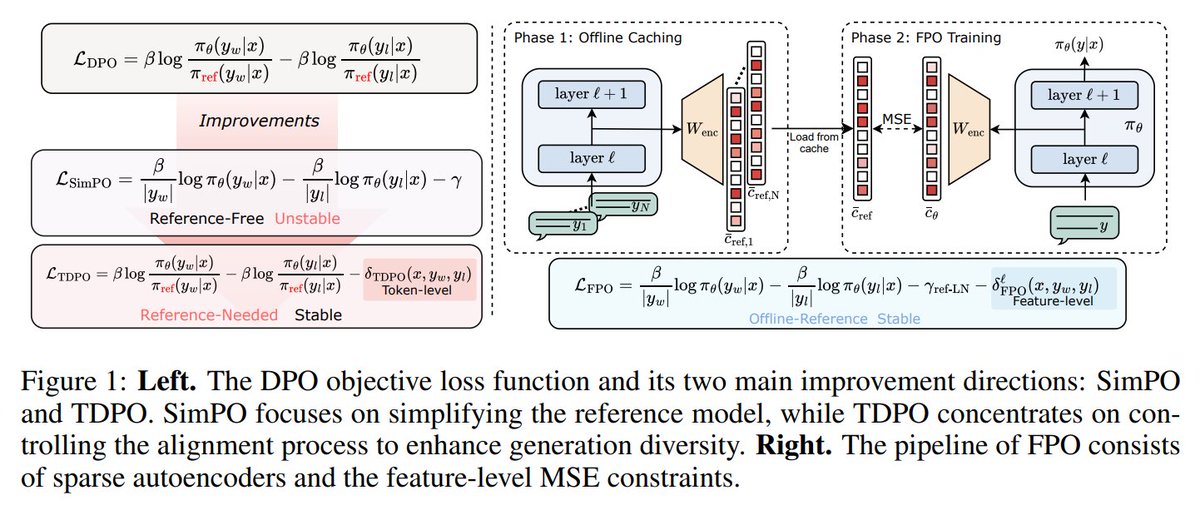
8/11
@TheTuringPost
7. Cut Your Losses In Large-Vocabulary Language Models
Proposes Cut Cross-Entropy (CCE) method that reduces memory use for large-scale training, enabling up to 10x larger batch sizes without sacrificing performance.
[2411.09009] Cut Your Losses in Large-Vocabulary Language Models
GitHub: GitHub - apple/ml-cross-entropy
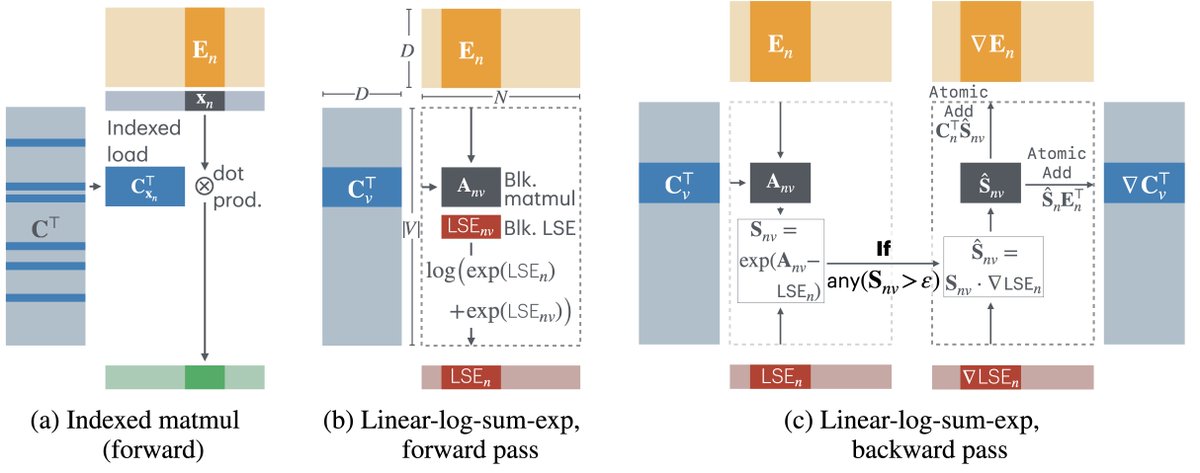
9/11
@TheTuringPost
8. SPARSING LAW: Towards Large Language Models With Greater Activation Sparsity
Explores neuron sparsity in LLMs to enhance efficiency while preserving interpretability.
[2411.02335] Sparsing Law: Towards Large Language Models with Greater Activation Sparsity
GitHub: GitHub - thunlp/SparsingLaw: The open-source materials for paper "Sparsing Law: Towards Large Language Models with Greater Activation Sparsity".
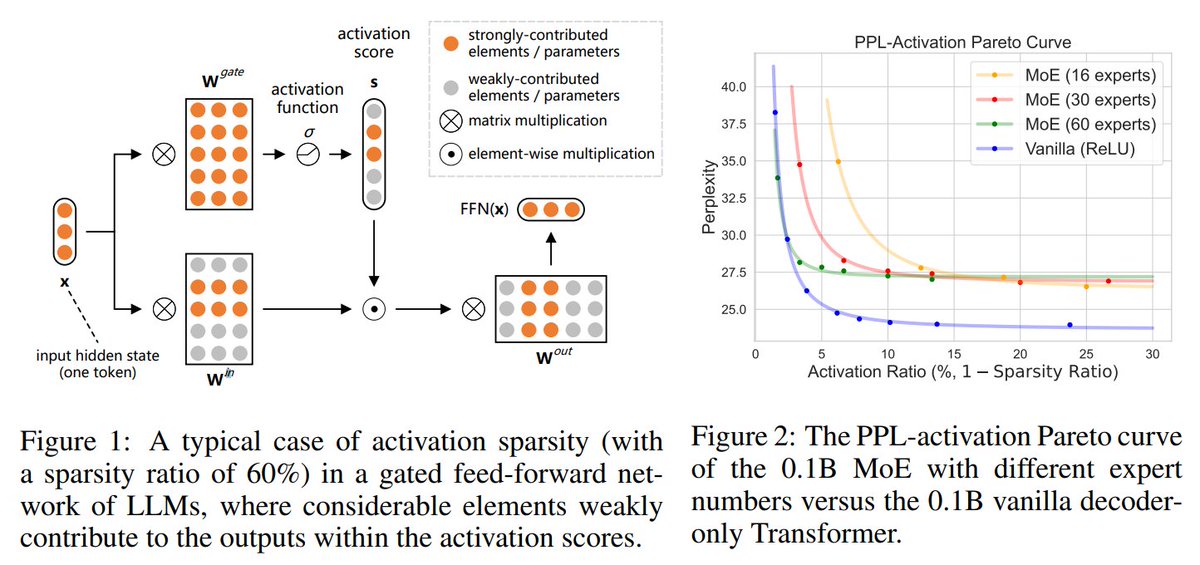
10/11
@TheTuringPost
9. Find a complete list of the latest research papers in our free weekly digest:

11/11
@TheTuringPost
10. Follow @TheTuringPost for more.
Like/repost the 1st post to support our work

Also, elevate your AI game with our free newsletter ↓
Turing Post
[Quoted tweet]
The freshest AI/ML researches of the week, part 1









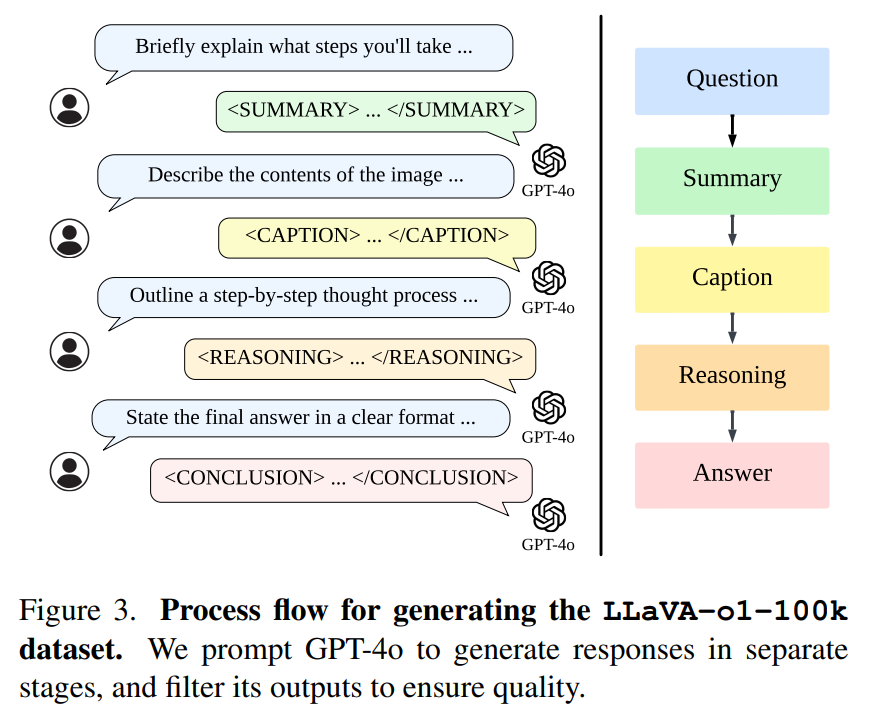
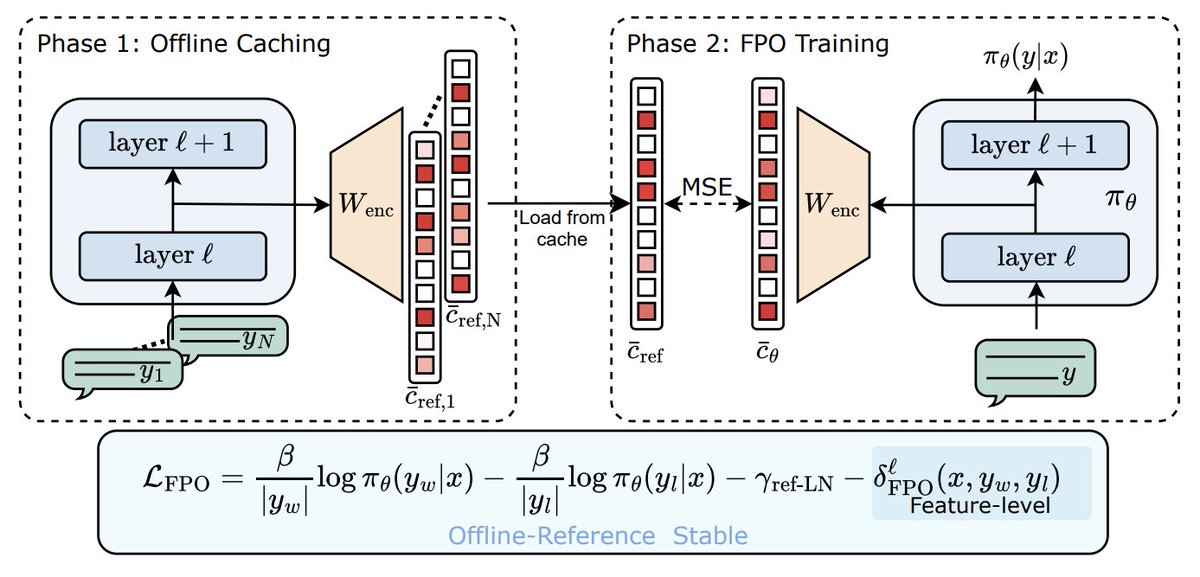
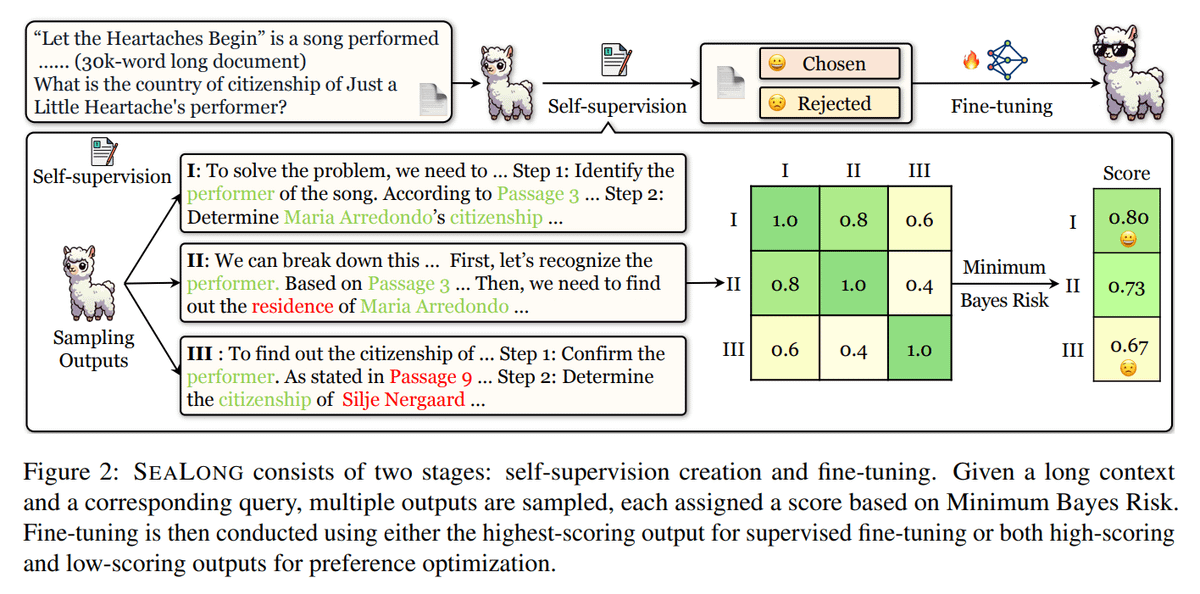
To post tweets in this format, more info here: https://www.thecoli.com/threads/tips-and-tricks-for-posting-the-coli-megathread.984734/post-52211196
1/3
@rohanpaul_ai
LLaVA-o1 teaches machines to think step-by-step like humans when analyzing images.
LLaVA-o1 introduces a novel approach to enhance Vision Language Models (VLMs) by implementing structured, multi-stage reasoning. This paper tackles the challenge of systematic reasoning in visual tasks by breaking down the process into distinct stages: summary, caption, reasoning, and conclusion.
-----
Original Problem:
Current VLMs struggle with systematic reasoning and often produce errors or hallucinated outputs during complex visual question-answering tasks. They lack structured thinking processes and tend to jump to conclusions without proper analysis.
-----
Solution in this Paper:
→ LLaVA-o1 implements a 4-stage reasoning process with dedicated tags for each stage: summary, caption, reasoning, and conclusion.
→ The model uses supervised fine-tuning on a new LLaVA-o1-100k dataset, created using GPT-4o for structured reasoning annotations.
→ A stage-level beam search method generates multiple candidates at each reasoning stage, selecting the best one to continue.
→ Training is performed on a single node with 8 H100 GPUs, combining samples from both general VQA and science-targeted datasets.
-----
Key Insights:
→ Structured reasoning stages help models organize thoughts before reaching conclusions
→ Special tags for each stage maintain clarity throughout the reasoning process
→ Stage-level beam search is more effective than sentence-level or best-of-N approaches
-----
Results:
→ Outperforms base model by 8.9% on multimodal reasoning benchmarks
→ Surpasses larger models including Gemini-1.5-pro and GPT-4o-mini
→ Stage-level beam search improves MMVet score from 60.3% to 62.9%
2/3
@rohanpaul_ai
Paper Title: "LLaVA-o1: Let Vision Language Models Reason Step-by-Step"
Generated below podcast on this paper with Google's Illuminate.
https://video.twimg.com/ext_tw_video/1860466160707469312/pu/vid/avc1/1080x1080/mAeNIFuBt10AwrXP.mp4
3/3
@rohanpaul_ai
[2411.10440] LLaVA-o1: Let Vision Language Models Reason Step-by-Step
To post tweets in this format, more info here: https://www.thecoli.com/threads/tips-and-tricks-for-posting-the-coli-megathread.984734/post-52211196
@rohanpaul_ai
LLaVA-o1 teaches machines to think step-by-step like humans when analyzing images.
LLaVA-o1 introduces a novel approach to enhance Vision Language Models (VLMs) by implementing structured, multi-stage reasoning. This paper tackles the challenge of systematic reasoning in visual tasks by breaking down the process into distinct stages: summary, caption, reasoning, and conclusion.
-----

Current VLMs struggle with systematic reasoning and often produce errors or hallucinated outputs during complex visual question-answering tasks. They lack structured thinking processes and tend to jump to conclusions without proper analysis.
-----

→ LLaVA-o1 implements a 4-stage reasoning process with dedicated tags for each stage: summary, caption, reasoning, and conclusion.
→ The model uses supervised fine-tuning on a new LLaVA-o1-100k dataset, created using GPT-4o for structured reasoning annotations.
→ A stage-level beam search method generates multiple candidates at each reasoning stage, selecting the best one to continue.
→ Training is performed on a single node with 8 H100 GPUs, combining samples from both general VQA and science-targeted datasets.
-----

→ Structured reasoning stages help models organize thoughts before reaching conclusions
→ Special tags for each stage maintain clarity throughout the reasoning process
→ Stage-level beam search is more effective than sentence-level or best-of-N approaches
-----

→ Outperforms base model by 8.9% on multimodal reasoning benchmarks
→ Surpasses larger models including Gemini-1.5-pro and GPT-4o-mini
→ Stage-level beam search improves MMVet score from 60.3% to 62.9%
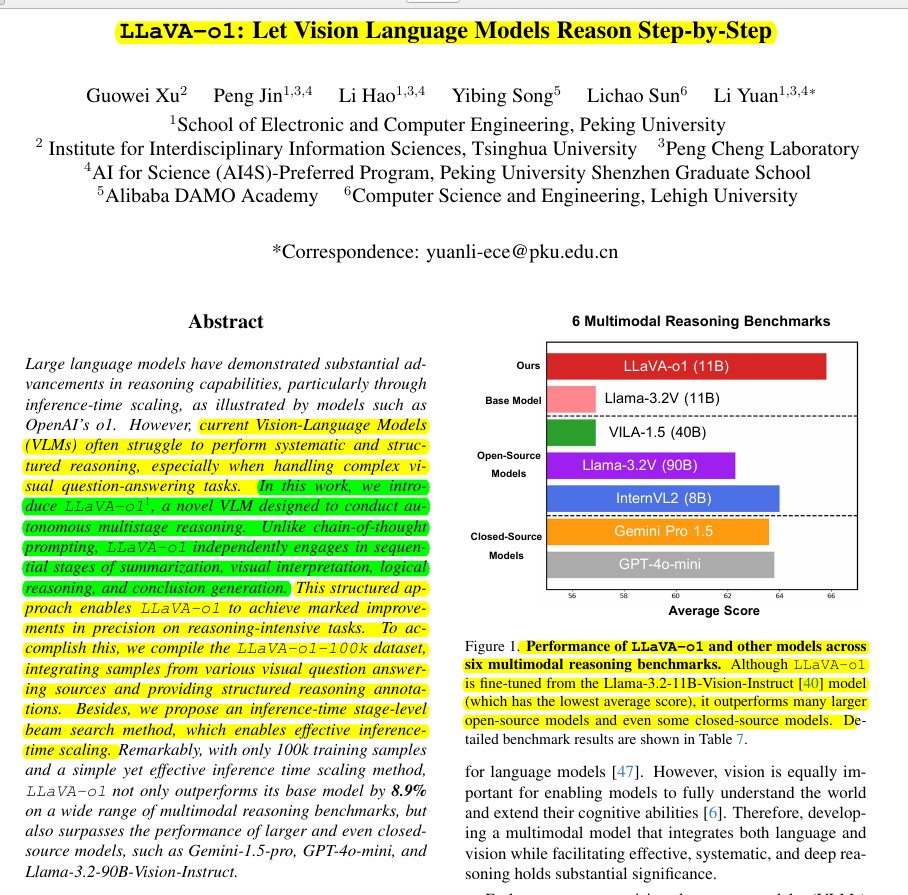
2/3
@rohanpaul_ai
Paper Title: "LLaVA-o1: Let Vision Language Models Reason Step-by-Step"
Generated below podcast on this paper with Google's Illuminate.
https://video.twimg.com/ext_tw_video/1860466160707469312/pu/vid/avc1/1080x1080/mAeNIFuBt10AwrXP.mp4
3/3
@rohanpaul_ai
[2411.10440] LLaVA-o1: Let Vision Language Models Reason Step-by-Step
To post tweets in this format, more info here: https://www.thecoli.com/threads/tips-and-tricks-for-posting-the-coli-megathread.984734/post-52211196
1/1
@jreuben1
LLaVA-o1: Let Vision Language Models Reason Step-by-Step LLaVA-o1: Let Vision Language Models Reason Step-by-Step inference-time stage-level beam search method, which enables effective inference-time scaling.
To post tweets in this format, more info here: https://www.thecoli.com/threads/tips-and-tricks-for-posting-the-coli-megathread.984734/post-52211196
@jreuben1
LLaVA-o1: Let Vision Language Models Reason Step-by-Step LLaVA-o1: Let Vision Language Models Reason Step-by-Step inference-time stage-level beam search method, which enables effective inference-time scaling.
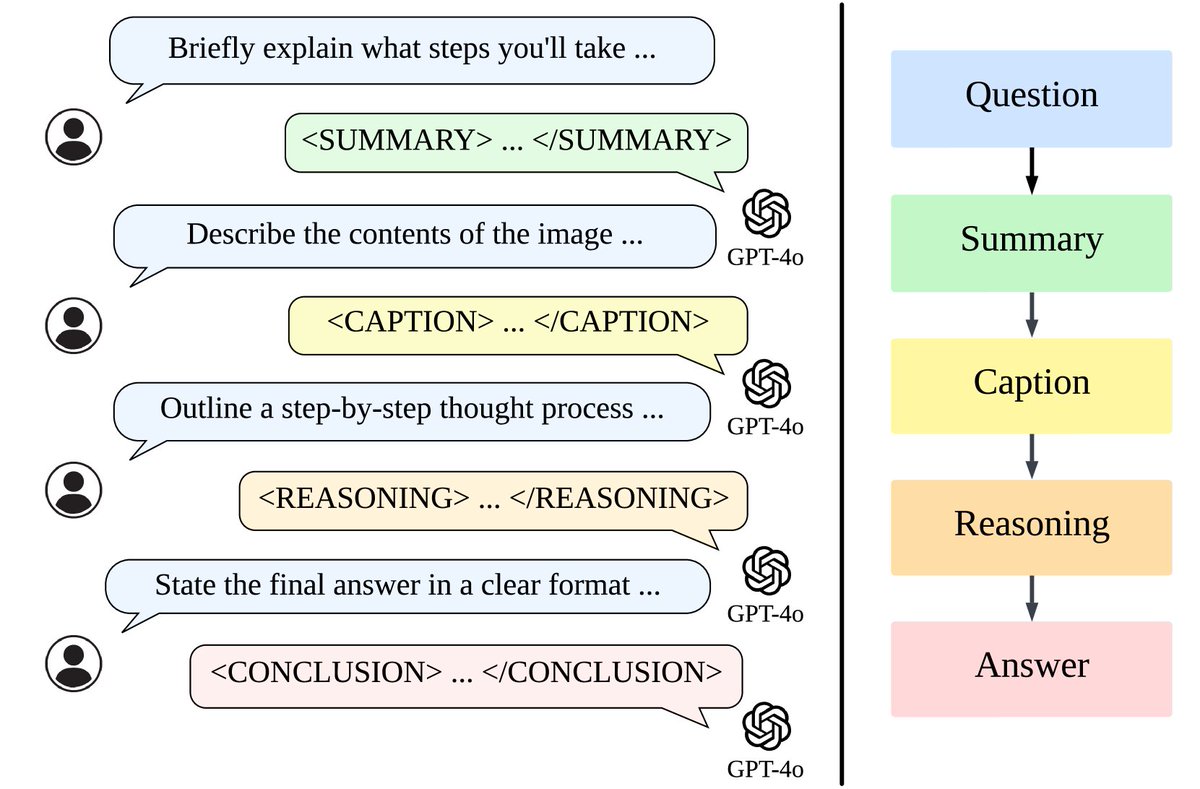
To post tweets in this format, more info here: https://www.thecoli.com/threads/tips-and-tricks-for-posting-the-coli-megathread.984734/post-52211196
1/10
@Gradio
LLaVA-o1 is the first visual language model capable of spontaneous, systematic reasoning, similar to GPT-o1!
11B model outperforms Gemini-1.5-pro,GPT-4o-mini, and Llama-3.2-90B-Vision-Instruct on six multimodal benchmarks.
2/10
@Gradio
LlaVA-o1
Stay tuned for the code and gradio app release.
GitHub - PKU-YuanGroup/LLaVA-o1
3/10
@NNaumovsky
@threadreaderapp unroll
4/10
@threadreaderapp
@NNaumovsky Namaste, please find the unroll here: Thread by @Gradio on Thread Reader App Enjoy

5/10
@CohorteAI
"LLaVA-o1’s success on multimodal benchmarks suggests it’s mastering the integration of vision and language. Could this pave the way for models capable of deeper real-world contextual understanding, like AR-enhanced assistants?
6/10
@hanul93
WOW
7/10
@arya_mukhlis354
amazing
8/10
@txhno
which image decoder does it use?
9/10
@matthaeus_win
I thought every model based on Llama 3 has to have 'Llama' in the name..
10/10
@wuwenjie1992
[Quoted tweet]
由北大信工袁粒课题组发布的 LLaVA-o1 是第一个能够进行自发、系统推理的视觉语言模型,类似于 GPT-o1!
⚙ 模型首先概述问题,解释图像中的相关信息,逐步进行推理,最终得出有充分依据的结论。
11B 的模型在六个多模态基准测试中优于 Gemini1.5pro、GPT4o-mini 和 Llama3.2-90B-Vision-Instruct。
To post tweets in this format, more info here: https://www.thecoli.com/threads/tips-and-tricks-for-posting-the-coli-megathread.984734/post-52211196
@Gradio
LLaVA-o1 is the first visual language model capable of spontaneous, systematic reasoning, similar to GPT-o1!

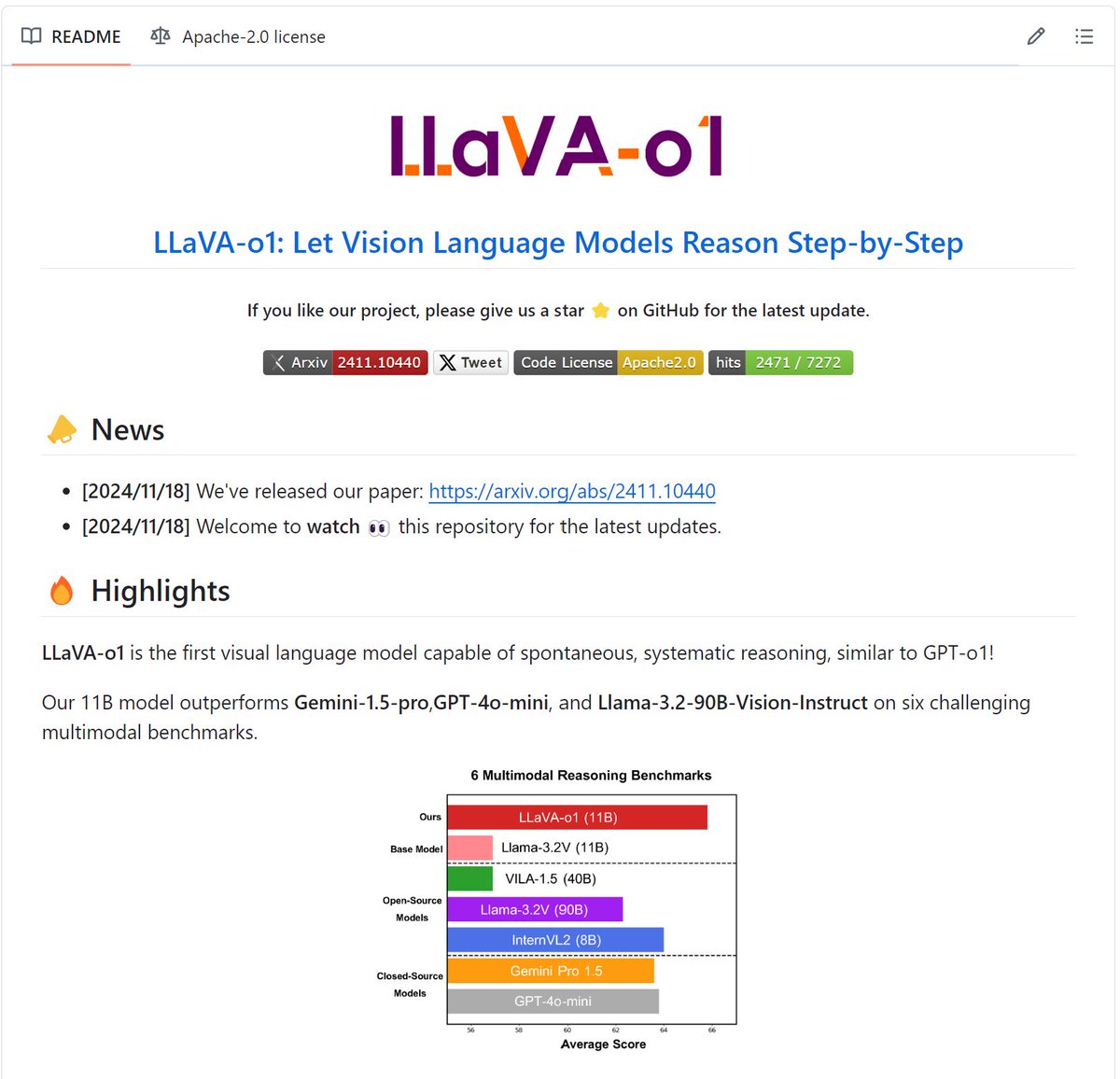
2/10
@Gradio
LlaVA-o1
Stay tuned for the code and gradio app release.
GitHub - PKU-YuanGroup/LLaVA-o1
3/10
@NNaumovsky
@threadreaderapp unroll
4/10
@threadreaderapp
@NNaumovsky Namaste, please find the unroll here: Thread by @Gradio on Thread Reader App Enjoy


5/10
@CohorteAI
"LLaVA-o1’s success on multimodal benchmarks suggests it’s mastering the integration of vision and language. Could this pave the way for models capable of deeper real-world contextual understanding, like AR-enhanced assistants?
6/10
@hanul93
WOW
7/10
@arya_mukhlis354
amazing
8/10
@txhno
which image decoder does it use?
9/10
@matthaeus_win
I thought every model based on Llama 3 has to have 'Llama' in the name..

10/10
@wuwenjie1992
[Quoted tweet]
由北大信工袁粒课题组发布的 LLaVA-o1 是第一个能够进行自发、系统推理的视觉语言模型,类似于 GPT-o1!
⚙ 模型首先概述问题,解释图像中的相关信息,逐步进行推理,最终得出有充分依据的结论。

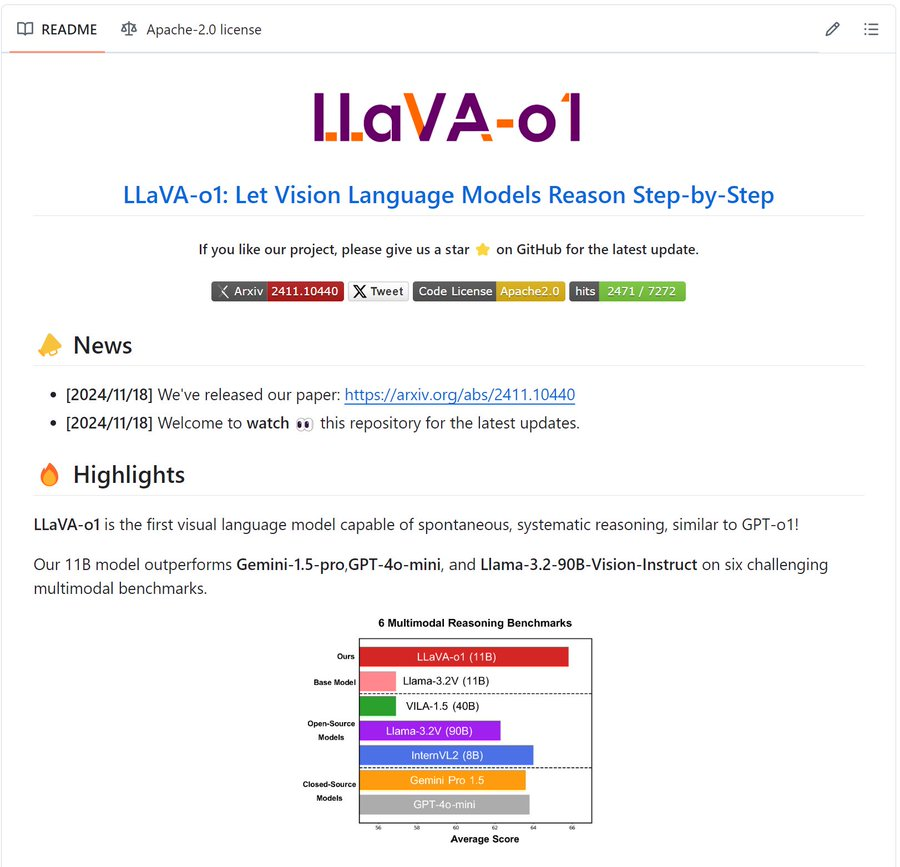
To post tweets in this format, more info here: https://www.thecoli.com/threads/tips-and-tricks-for-posting-the-coli-megathread.984734/post-52211196