1/3
@rohanpaul_ai
LLMs gain human-like awareness of word positions through numbered tracking.
Adding position markers to LLM inputs enables exact length control and accurate text manipulation.
**Original Problem**
:
LLMs struggle with length control and precise copy-paste operations due to lack of positional awareness.
The authors identify a lack of positional awareness as the root cause of LLMs' inability to effectively control text length. This stems from token-level operations and insufficient training on data with strict length limitations.
-----
**Solution in this Paper**
:
• PositionID Prompting: Assigns sequential IDs to words/sentences/paragraphs during generation
• PositionID Fine-Tuning: Trains models on mixed normal and PositionID modes
• PositionID CP Prompting: Enables accurate copy-paste using a three-stage tool-use mechanism
-----
**Key Insights from this Paper**
:
• Explicit positional awareness enhances LLMs' length control and copy-paste abilities
• PositionID techniques work for both closed-source and open-source models
• Mixed-mode training transfers positional awareness to normal generation mode
-----
**Results**
:
• PositionID Prompting: Best Rouge-L (23.2) and MAE scores across all levels
• PositionID Fine-Tuning: Outperforms CFT and InstructCTG in MAE metrics
• PositionID CP Prompting: 80.8% CP Success Rate, 18.4 Rouge-L, 8.4 PPL
2/3
@rohanpaul_ai
LenCtrl-Bench Details
This component has three workflow variants:
Vanilla Prompting:
- Takes user query and length constraint
- Directly generates text without position tracking
- Less accurate length control
PositionID Prompting:
- Adds sequential position IDs to each word/token
- Helps model track length during generation
- More precise length control
- Example: "Three 1 -word 2 text 3"
PositionID Fine-Tuning:
- Trains model in two modes:
- Normal mode (without position IDs)
- PositionID mode (with position IDs)
- Infers in normal mode while retaining positional awareness
- Most effective for length control
3/3
@rohanpaul_ai
[2410.07035] PositionID: LLMs can Control Lengths, Copy and Paste with Explicit Positional Awareness
To post tweets in this format, more info here: https://www.thecoli.com/threads/tips-and-tricks-for-posting-the-coli-megathread.984734/post-52211196
@rohanpaul_ai
LLMs gain human-like awareness of word positions through numbered tracking.
Adding position markers to LLM inputs enables exact length control and accurate text manipulation.
**Original Problem**

LLMs struggle with length control and precise copy-paste operations due to lack of positional awareness.
The authors identify a lack of positional awareness as the root cause of LLMs' inability to effectively control text length. This stems from token-level operations and insufficient training on data with strict length limitations.
-----
**Solution in this Paper**

• PositionID Prompting: Assigns sequential IDs to words/sentences/paragraphs during generation
• PositionID Fine-Tuning: Trains models on mixed normal and PositionID modes
• PositionID CP Prompting: Enables accurate copy-paste using a three-stage tool-use mechanism
-----
**Key Insights from this Paper**

• Explicit positional awareness enhances LLMs' length control and copy-paste abilities
• PositionID techniques work for both closed-source and open-source models
• Mixed-mode training transfers positional awareness to normal generation mode
-----
**Results**

• PositionID Prompting: Best Rouge-L (23.2) and MAE scores across all levels
• PositionID Fine-Tuning: Outperforms CFT and InstructCTG in MAE metrics
• PositionID CP Prompting: 80.8% CP Success Rate, 18.4 Rouge-L, 8.4 PPL
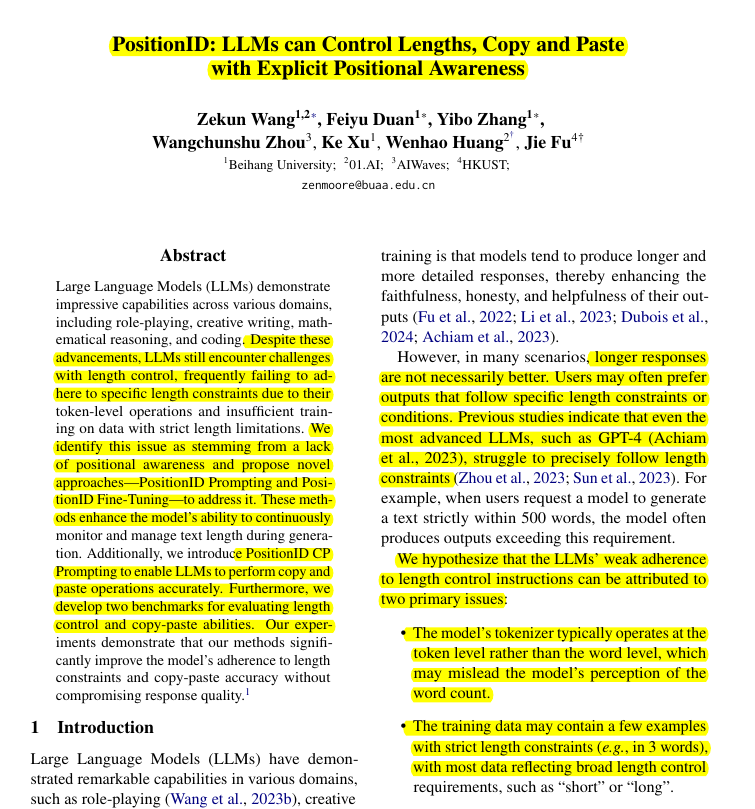
2/3
@rohanpaul_ai

This component has three workflow variants:

- Takes user query and length constraint
- Directly generates text without position tracking
- Less accurate length control

- Adds sequential position IDs to each word/token
- Helps model track length during generation
- More precise length control
- Example: "Three 1 -word 2 text 3"

- Trains model in two modes:
- Normal mode (without position IDs)
- PositionID mode (with position IDs)
- Infers in normal mode while retaining positional awareness
- Most effective for length control
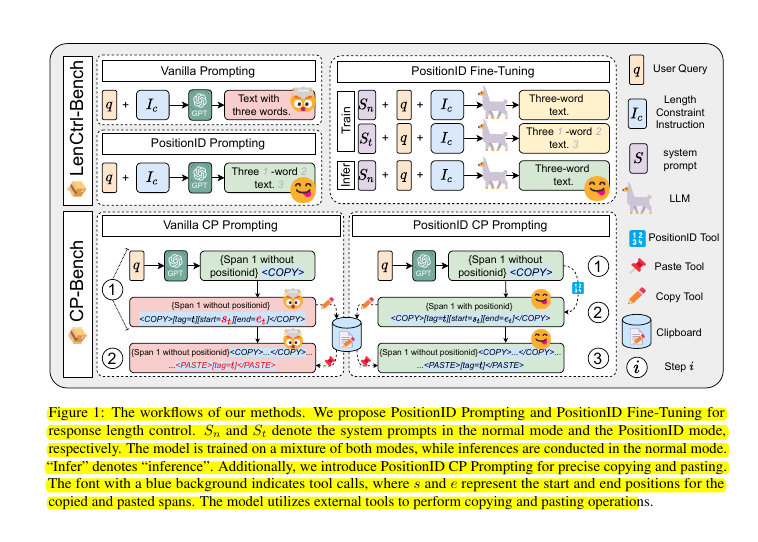
3/3
@rohanpaul_ai

To post tweets in this format, more info here: https://www.thecoli.com/threads/tips-and-tricks-for-posting-the-coli-megathread.984734/post-52211196
1/1
@arXivGPT
:PositionID: LLMs can Control Lengths, Copy and Paste with Explicit Positional Awareness
:https://arxiv.org/pdf/2410.07035.pdf
To post tweets in this format, more info here: https://www.thecoli.com/threads/tips-and-tricks-for-posting-the-coli-megathread.984734/post-52211196
@arXivGPT


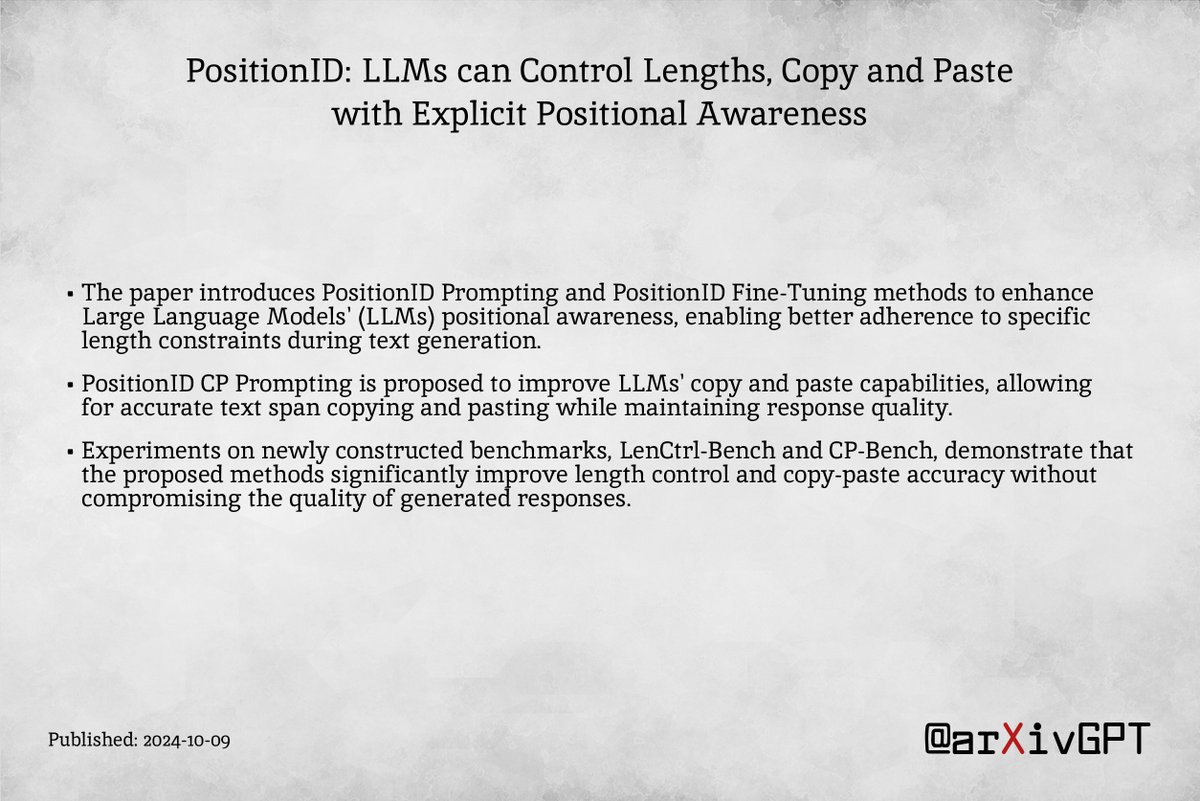
To post tweets in this format, more info here: https://www.thecoli.com/threads/tips-and-tricks-for-posting-the-coli-megathread.984734/post-52211196