1/7
New: my last PhD paper 
Introducing System-1.x, a controllable planning framework with LLMs. It draws inspiration from Dual-Process Theory, which argues for the co-existence of fast/intuitive System-1 and slow/deliberate System-2 planning.
System 1.x generates hybrid plans & balances between the two planning modes (efficient + inaccurate System-1 & inefficient + more accurate System-2) based on the difficulty of the decomposed (sub-)problem at hand.
Some exciting results+features of System-1.x:
-- performance: beats System-1, System-2 & a symbolic planner (A*) both ID and OOD (up to 39%), given an exploration budget.
-- training-time control/balance: user can train a System-1.25/1.5/1.75 to balance accuracy + efficiency.
-- test-time control/balance: user can bias the planner to solve more/less sub-goals using System-2.
-- flexibility to integrate symbolic solvers: allows building neuro-symbolic System-1.x with a symbolic System-2 (A*).
-- generalizability: can learn from different search algos (DFS/BFS/A*).


2/7
System-1.x consists of 3 components (trained using only search traces as supervision):
1⃣ a Controller, that decomposes a planning problem into easy+hard sub-goals
2⃣ a System-1 Planner, that solves easy sub-goals
3⃣ a System-2 Planner, that solves hard sub-goals w/ deliberate search
3/7
To train the controller, we automatically generate sub-goal decompositions, given 2 things:
– a hybridization factor x, that decides Sys-1 to Sys-2 balance in the planner.
– a hardness function, estimating difficulty of a sub-goal
Then we solve a constrained optimization problem that finds a contiguous x% of steps in the plan such that it corresponds to the hardest sub-goal.
4/7
On both Maze Navigation & Blocksworld domains, we get exciting results both ID & OOD:
System 1.x matches & generally outperforms System-1, System-2 & also a simpler hybrid w/o sub-goal decompositions at all state-exploration budgets by up to 33%.
Neuro-symbolic System 1.x also typically outperforms symbolic search, beating A* by up to 39% at a fixed budget & matching it at max budget.
5/7
Train-time controllability: We train a System-1.75 Planner that, compared to a System-1.5 Planner, trades off efficiency for greater accuracy. This trend can be continued to recover the full System-2 performance.
Generalizability: System-1.x also generalizes to different search algos (BFS, DFS, A*) & exhibits exploration behavior that closely resembles the corresponding algo it is trained on.
6/7
Here's a nice illustration of System 1.x for Maze:
System-1 ignores obstacles
fail
System-2 reaches a dead end + search does not terminate
fail
System-1.x decomposes into sub-goals & solves the middle hard sub-goal w/ obstacles using System-2
success
In Blocksworld, we also show an example of sub-goal decomposition benefitting System-1.x.
7/7
Paper link: [2407.14414] System-1.x: Learning to Balance Fast and Slow Planning with Language Models
Code link: GitHub - swarnaHub/System-1.x: PyTorch code for System-1.x: Learning to Balance Fast and Slow Planning with Language Models
work done w/ @ArchikiPrasad @cyjustinchen @peterbhase @EliasEskin @mohitban47
@uncnlp @UNC
To post tweets in this format, more info here: https://www.thecoli.com/threads/tips-and-tricks-for-posting-the-coli-megathread.984734/post-52211196


Introducing System-1.x, a controllable planning framework with LLMs. It draws inspiration from Dual-Process Theory, which argues for the co-existence of fast/intuitive System-1 and slow/deliberate System-2 planning.
System 1.x generates hybrid plans & balances between the two planning modes (efficient + inaccurate System-1 & inefficient + more accurate System-2) based on the difficulty of the decomposed (sub-)problem at hand.
Some exciting results+features of System-1.x:
-- performance: beats System-1, System-2 & a symbolic planner (A*) both ID and OOD (up to 39%), given an exploration budget.
-- training-time control/balance: user can train a System-1.25/1.5/1.75 to balance accuracy + efficiency.
-- test-time control/balance: user can bias the planner to solve more/less sub-goals using System-2.
-- flexibility to integrate symbolic solvers: allows building neuro-symbolic System-1.x with a symbolic System-2 (A*).
-- generalizability: can learn from different search algos (DFS/BFS/A*).


2/7
System-1.x consists of 3 components (trained using only search traces as supervision):
1⃣ a Controller, that decomposes a planning problem into easy+hard sub-goals
2⃣ a System-1 Planner, that solves easy sub-goals
3⃣ a System-2 Planner, that solves hard sub-goals w/ deliberate search
3/7
To train the controller, we automatically generate sub-goal decompositions, given 2 things:
– a hybridization factor x, that decides Sys-1 to Sys-2 balance in the planner.
– a hardness function, estimating difficulty of a sub-goal
Then we solve a constrained optimization problem that finds a contiguous x% of steps in the plan such that it corresponds to the hardest sub-goal.
4/7
On both Maze Navigation & Blocksworld domains, we get exciting results both ID & OOD:


5/7


6/7
Here's a nice illustration of System 1.x for Maze:






In Blocksworld, we also show an example of sub-goal decomposition benefitting System-1.x.
7/7
Paper link: [2407.14414] System-1.x: Learning to Balance Fast and Slow Planning with Language Models
Code link: GitHub - swarnaHub/System-1.x: PyTorch code for System-1.x: Learning to Balance Fast and Slow Planning with Language Models
work done w/ @ArchikiPrasad @cyjustinchen @peterbhase @EliasEskin @mohitban47
@uncnlp @UNC
To post tweets in this format, more info here: https://www.thecoli.com/threads/tips-and-tricks-for-posting-the-coli-megathread.984734/post-52211196
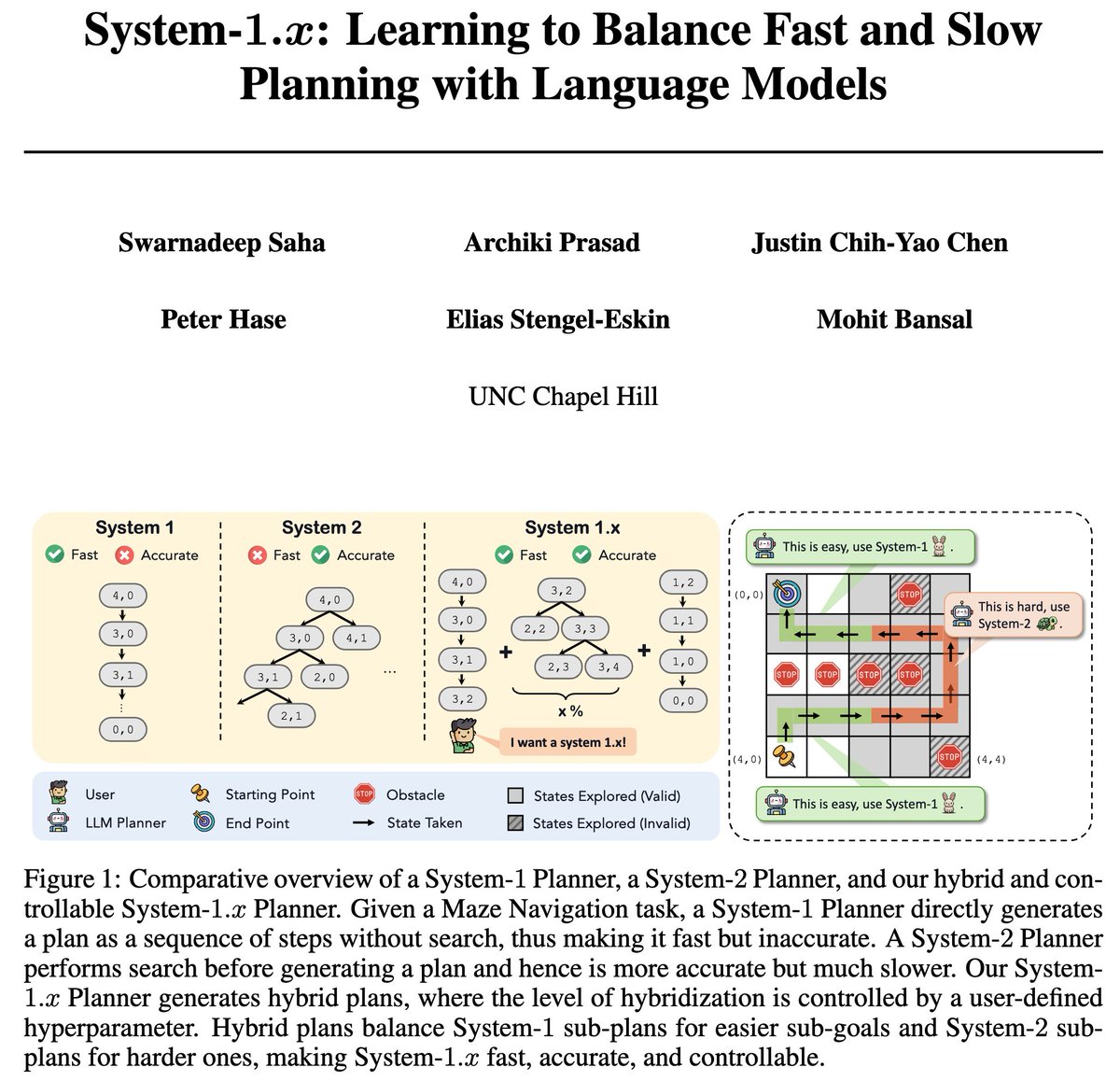
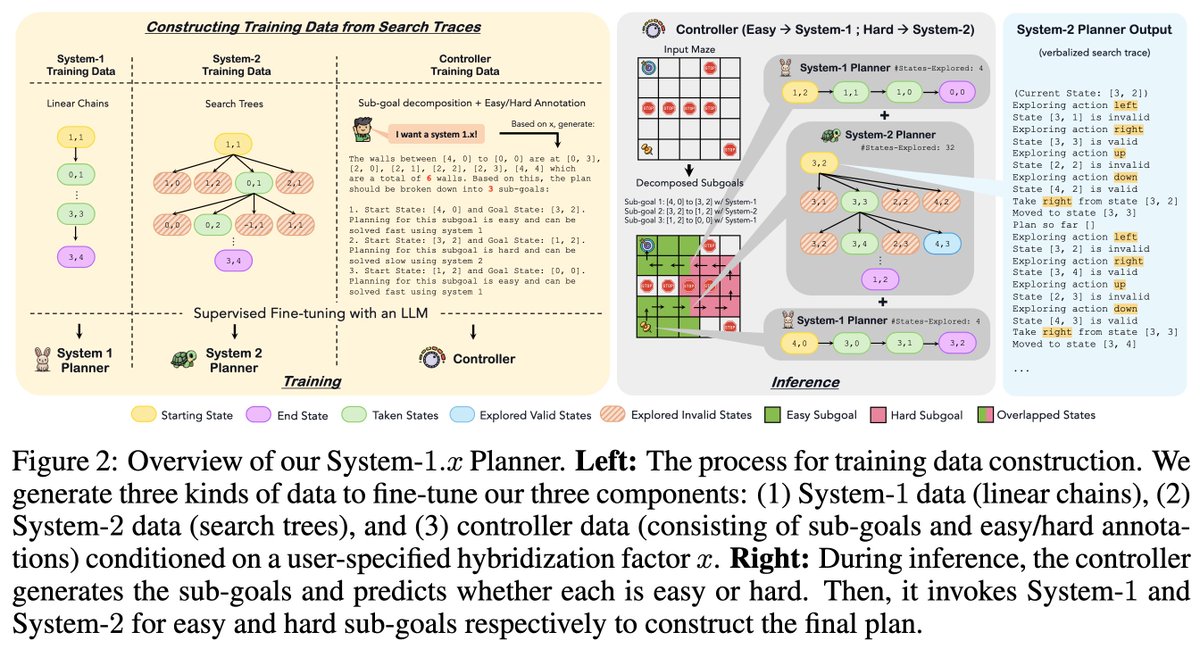
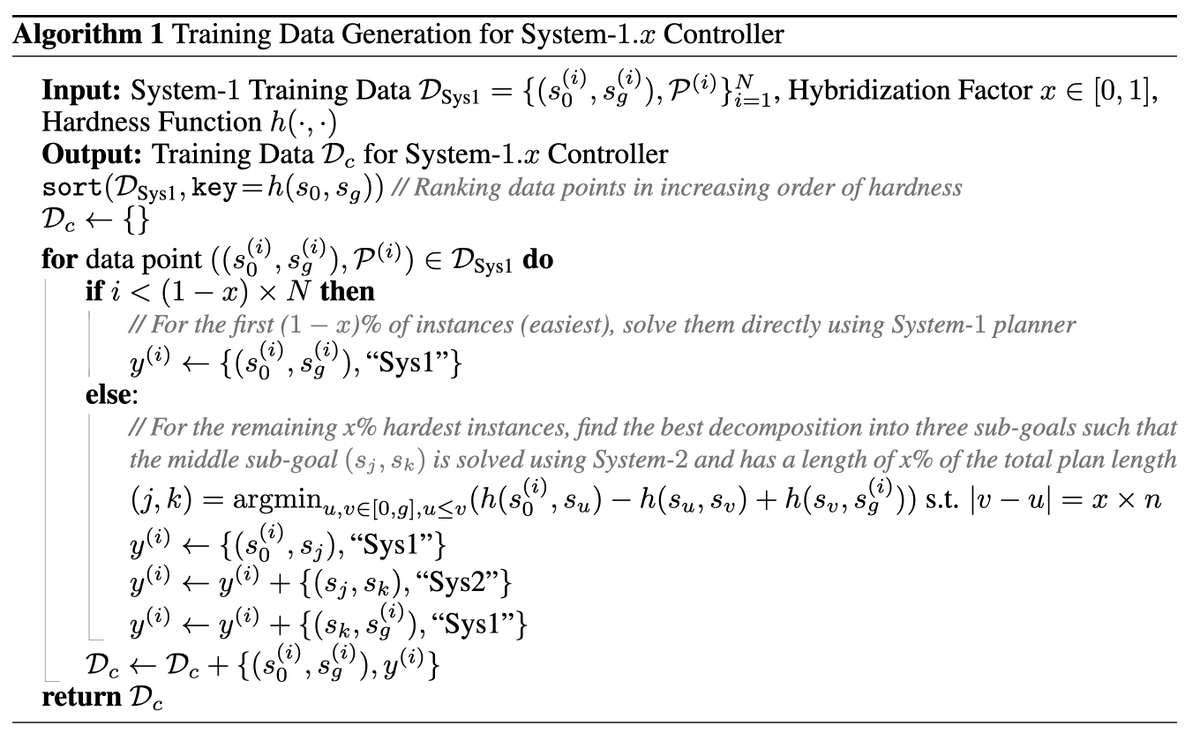
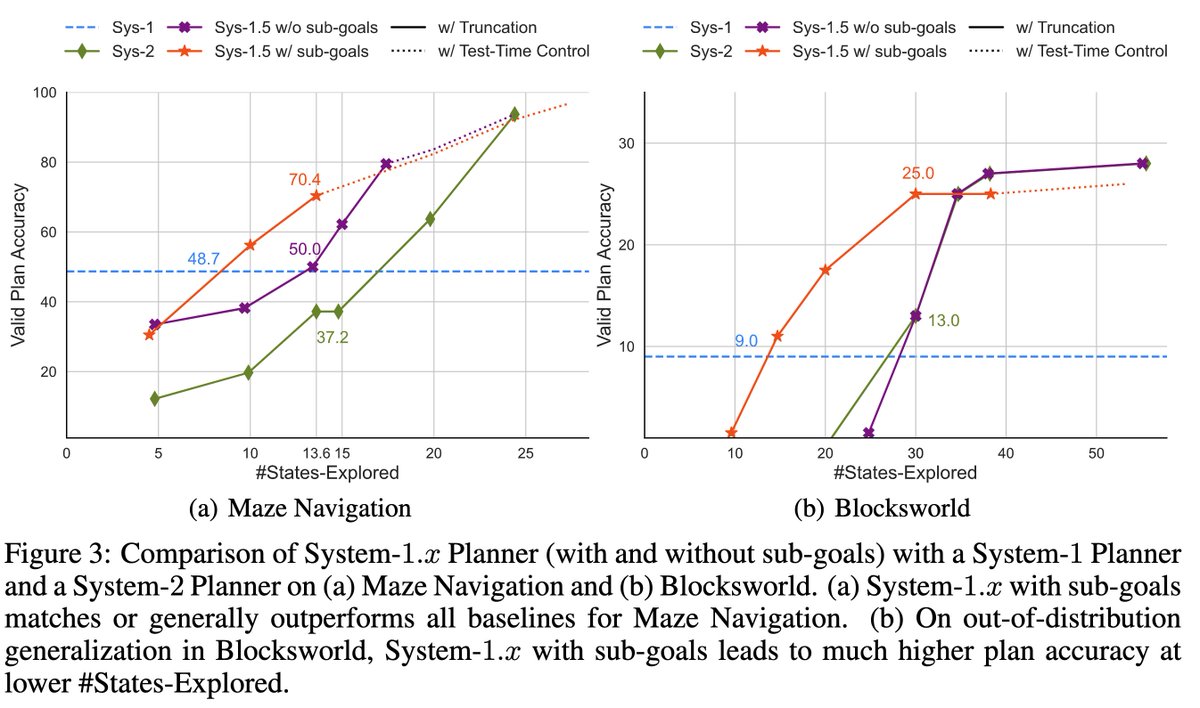
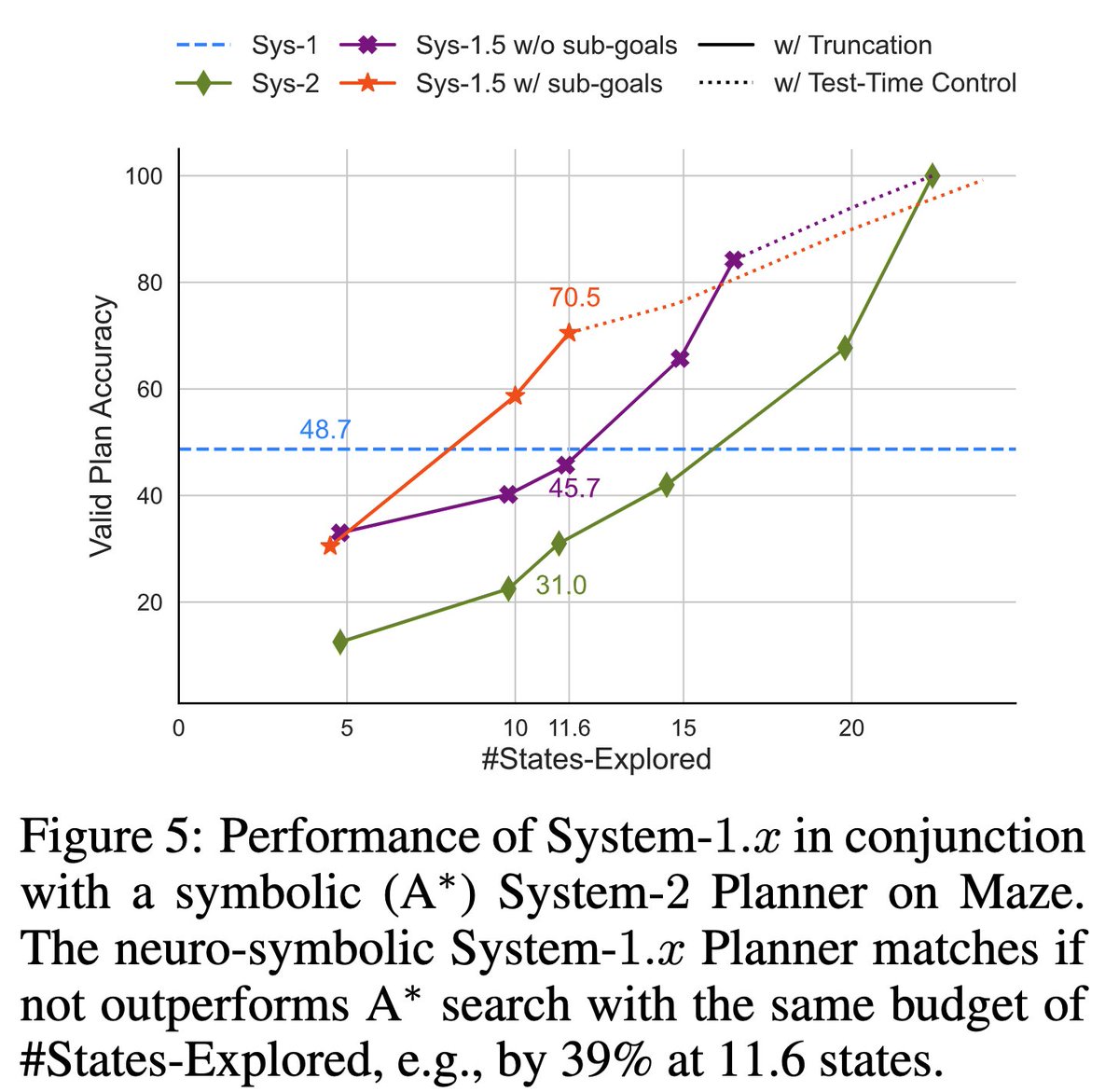
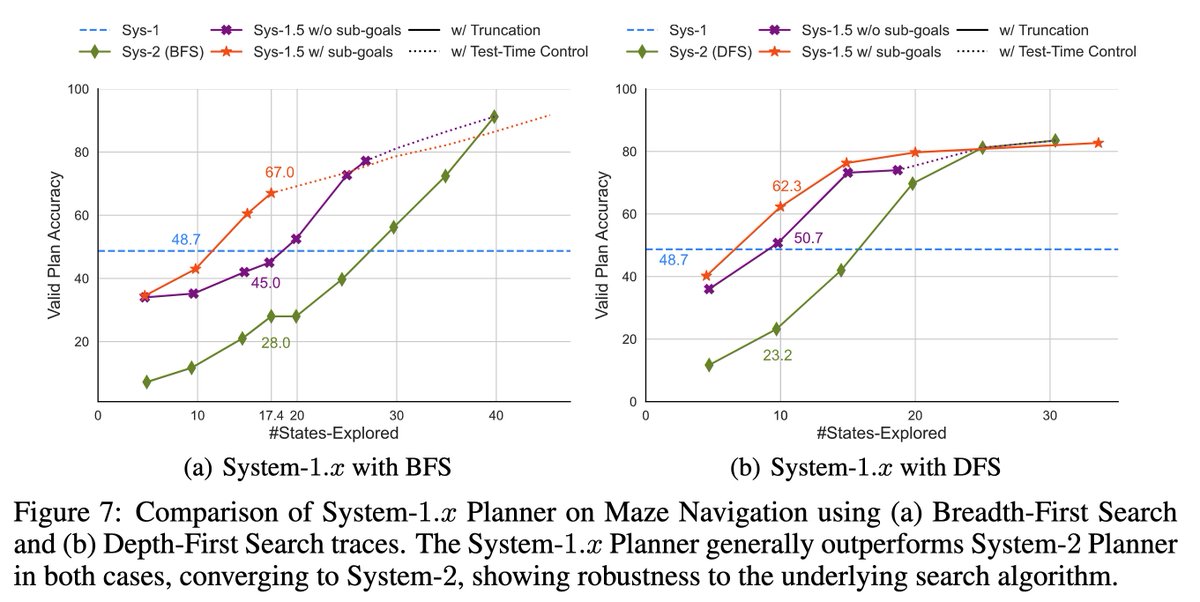
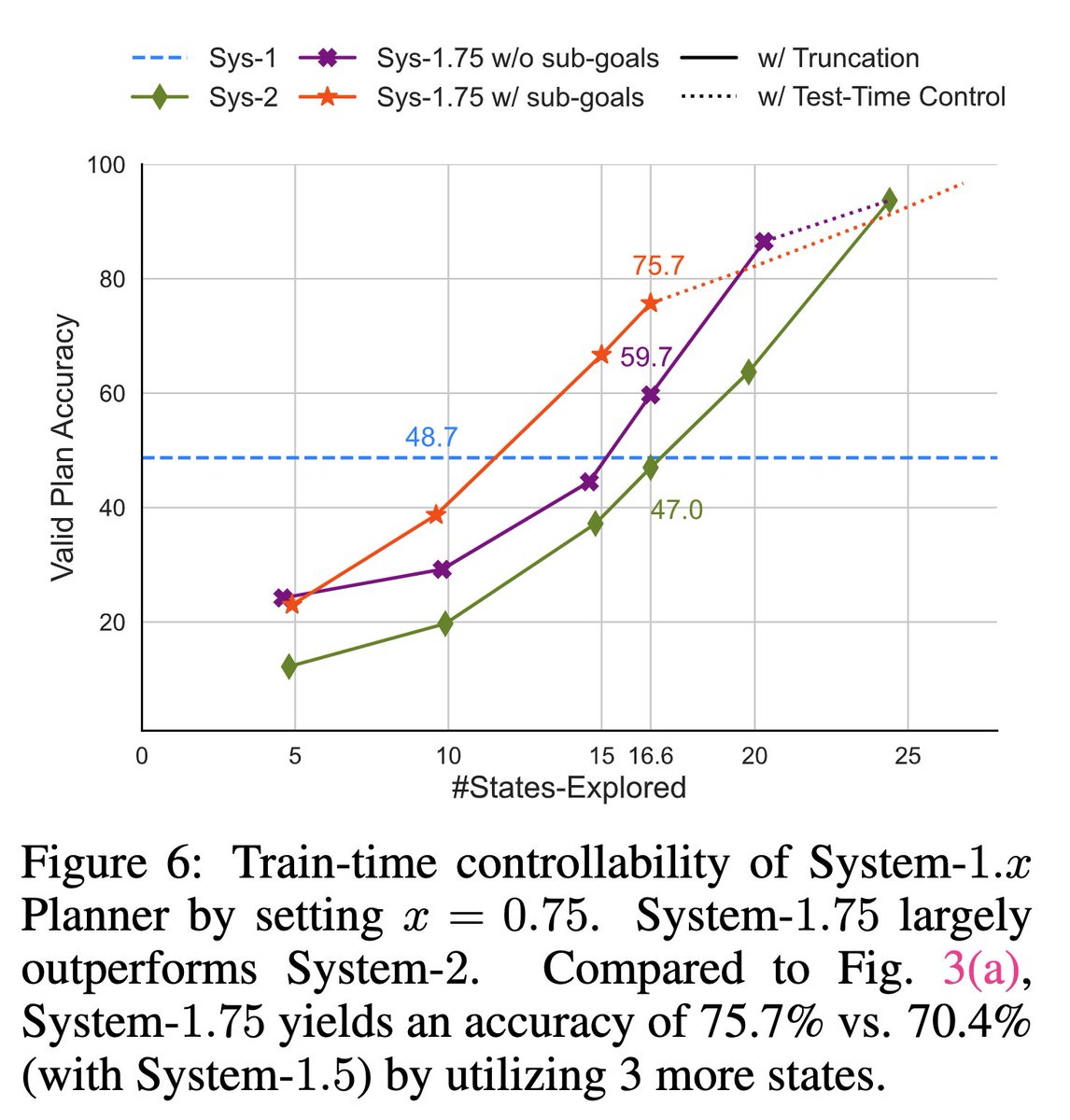
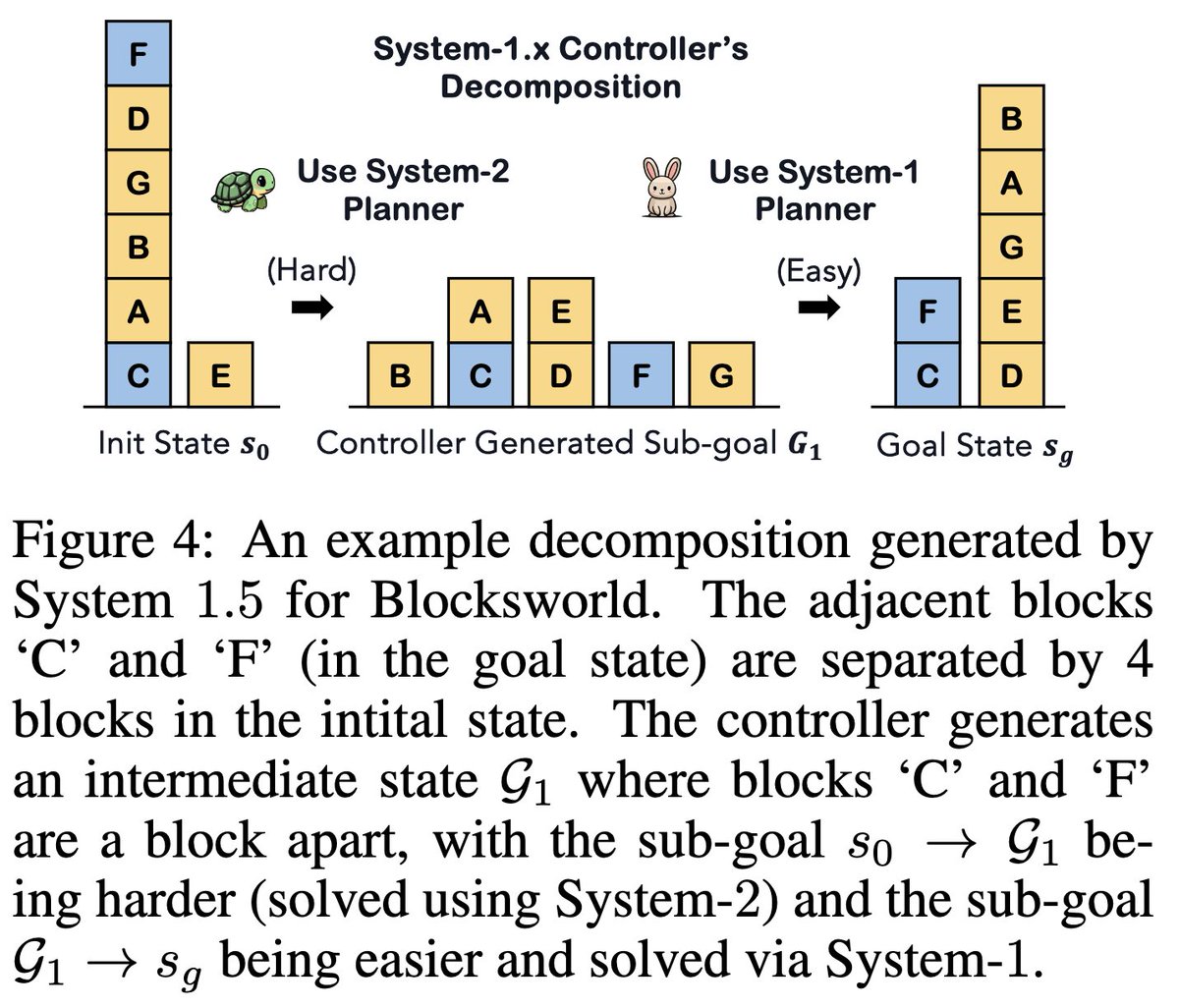
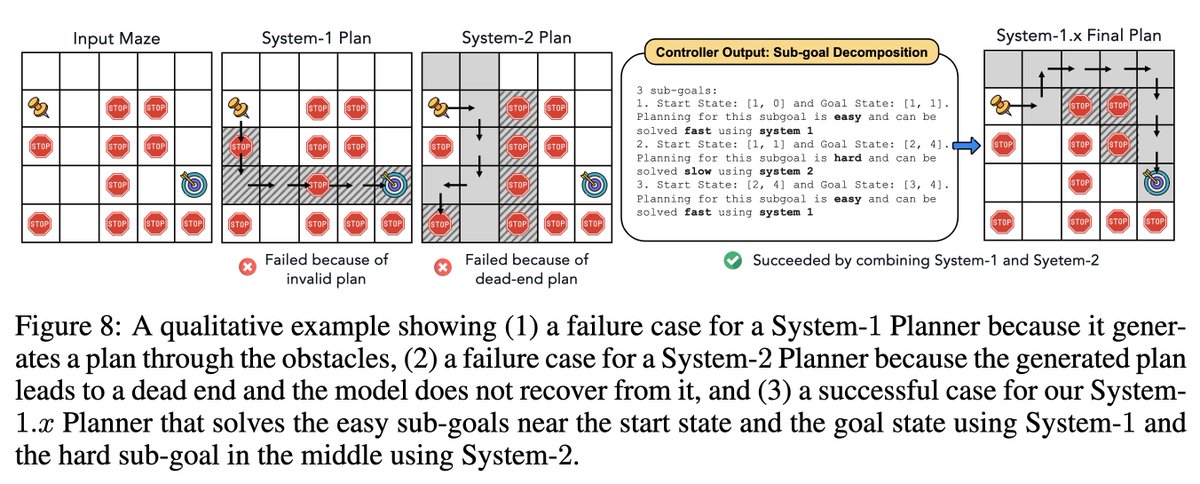