Meet Koala: Berkeley University’s LLaMA-Based Model Fine-Tuned with ChatGPT Dialogues
Created Using Midjourney
The accidental leak of the weights associated with Meta AI’s LLM LLaMA has sparked a tremendous level of innovation in the open-source LLM space. Since the furtious leak, we have seen models like Alpaca, Vicuna, ChatLlama and several others expand on the foundations of LLaMA to build innovative conversational agents that match the capabilities of ChatGPT. One of the latest addition to the list is Koala( yes I know, another animal-named model), a chatbot created by Berkeley AI Research(BAIR) that fine-tunes LLaMA on conversations gathered from the internet.
The core goal of Koala is to highlight the balance between mega-large closed-source LLMs and smaller, open-source LLMs. BAIR’s thesis is that smaller models can achieve performance that matches mega models like ChatGPT with a fraction of the cost while also improving in areas such as fine-tuning, transparency, and many others.
Image Credit: BAIR
The results suggest that using high-quality datasets can overcome some of the limitations of smaller models and may even match the capabilities of large closed-source models in the future. The research team recommends that the community should prioritize curating high-quality datasets, as this may enable the creation of safer, more factual, and more capable models than simply increasing the size of existing systems.
One of the interesting aspects of Koala was the data sources used for training. The fine-tuning datasets include data curated from ChatGPT dialogs. The fine-tuning strategy included the following datasets:
· ShareGPT: Around 60K dialogues shared by users on ShareGPT were collected through public APIs. To ensure data quality, the team deduplicated to the user-query level and removed non-English conversations. The resulting dataset comprises approximately 30K examples.
· HC3: The team used the human and ChatGPT responses from the HC3 English dataset, which includes roughly 60K human answers and 27K ChatGPT answers for approximately 24K questions. This results in a total of around 87K question-answer examples.
· OIG: A subset of components from the Open Instruction Generalist dataset curated by LAION was used, including the grade-school-math-instructions, the poetry-to-songs, and the plot-screenplay-books-dialogue datasets. The selected subset results in a total of around 30K examples.
· Stanford Alpaca: The team included the dataset used to train the Stanford Alpaca model, which contains approximately 52K examples generated by OpenAI’s text-davinci-003 through the self-instruct process. It is worth noting that HC3, OIG, and Alpaca datasets are single-turn question answering while ShareGPT dataset is dialogue conversations.
· Anthropic HH: The team utilized the Anthropic HH dataset, which includes around 160K human-rated examples. Each example consists of a pair of responses from a chatbot, one of which is preferred by humans. The dataset provides both capabilities and additional safety protections for the model.
· OpenAI WebGPT: The OpenAI WebGPT dataset includes approximately 20K comparisons where each example comprises a question, a pair of model answers, and metadata. The answers are rated by humans with a preference score.
· OpenAI Summarization: The OpenAI summarization dataset contains approximately 93K examples, each example consisting of feedback from humans regarding the summarizations generated by a model. Human evaluators chose the superior summary from two options.
A comparison between Koala, ChatGPT and open source models like Alpaca can be seen in the following matrix:
Image Credit: BAIR
EasyLM is built on top of Hugginface’s transformers and datasets, providing a user-friendly and customizable codebase for training large language models without the complexity of many other frameworks. By utilizing JAX’s pjit utility, EasyLM can train large models that do not fit on a single accelerator by sharding the model weights and training data across multiple accelerators. Currently, EasyLM supports multiple TPU/GPU training in a single host as well as multi-host training on Google Cloud TPU Pods.
Koala, on the other hand, was trained on a single Nvidia DGX server equipped with 8 A100 GPUs. The training process took approximately 6 hours to complete for 2 epochs. This type of training run typically costs less than $100 on public cloud computing platforms using preemptible instances.
The open-source release of Koala came accompanied by an online demo and the code for preprocessing the training data.
Koala represents an interesting iteration of the LlaMA models and one that sheds some light on the viability of smaller open-source alternatives to ChatGPT-like models.
Meet Koala: Berkeley University’s LLaMA-Based Model Fine-Tuned with ChatGPT Dialogues
The model provides a lighter, open-source alternative to ChatGPT and includes EasyLM, a framework for training and fine-tuning LLMs.
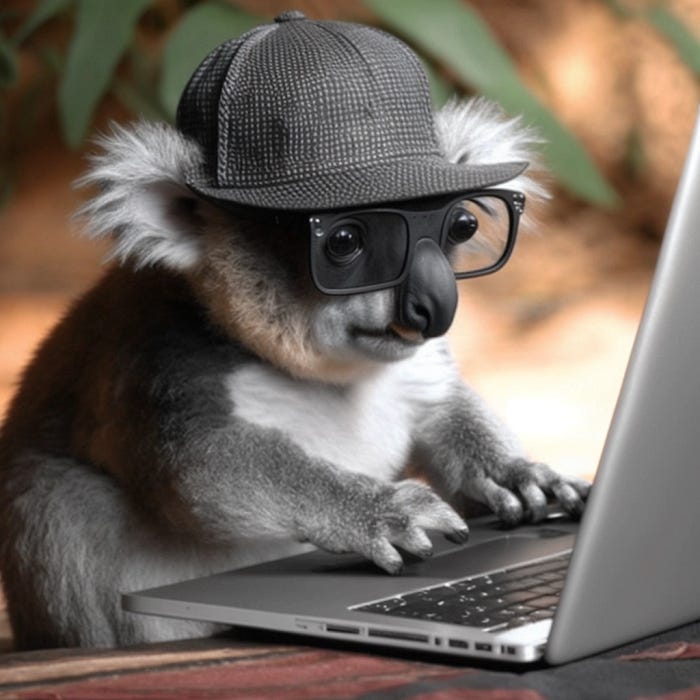
Created Using Midjourney
I recently started an AI-focused educational newsletter, that already has over 150,000 subscribers. TheSequence is a no-BS (meaning no hype, no news etc) ML-oriented newsletter that takes 5 minutes to read. The goal is to keep you up to date with machine learning projects, research papers and concepts. Please give it a try by subscribing below:
TheSequence | Jesus Rodriguez | Substack
The best source to stay up-to-date with the developments in the machine learning, artificial intelligence, and data…
thesequence.substack.comThe accidental leak of the weights associated with Meta AI’s LLM LLaMA has sparked a tremendous level of innovation in the open-source LLM space. Since the furtious leak, we have seen models like Alpaca, Vicuna, ChatLlama and several others expand on the foundations of LLaMA to build innovative conversational agents that match the capabilities of ChatGPT. One of the latest addition to the list is Koala( yes I know, another animal-named model), a chatbot created by Berkeley AI Research(BAIR) that fine-tunes LLaMA on conversations gathered from the internet.
The core goal of Koala is to highlight the balance between mega-large closed-source LLMs and smaller, open-source LLMs. BAIR’s thesis is that smaller models can achieve performance that matches mega models like ChatGPT with a fraction of the cost while also improving in areas such as fine-tuning, transparency, and many others.
Koala
Koala is a version of LlaMA fine-tuned on dialogue data scraped from the web and public datasets, including high-quality responses to user queries from other large language models, as well as question-answering datasets and human feedback datasets. Koala has been specifically trained on interaction data scraped from the web, with a focus on data that includes interaction with highly capable closed-source models such as ChatGPT. The resulting model, Koala-13B, demonstrates competitive performance to existing models based on human evaluation of real-world user prompts.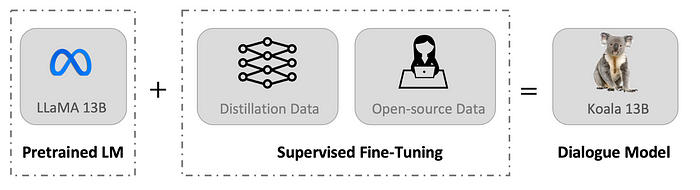
Image Credit: BAIR
The results suggest that using high-quality datasets can overcome some of the limitations of smaller models and may even match the capabilities of large closed-source models in the future. The research team recommends that the community should prioritize curating high-quality datasets, as this may enable the creation of safer, more factual, and more capable models than simply increasing the size of existing systems.
One of the interesting aspects of Koala was the data sources used for training. The fine-tuning datasets include data curated from ChatGPT dialogs. The fine-tuning strategy included the following datasets:
· ShareGPT: Around 60K dialogues shared by users on ShareGPT were collected through public APIs. To ensure data quality, the team deduplicated to the user-query level and removed non-English conversations. The resulting dataset comprises approximately 30K examples.
· HC3: The team used the human and ChatGPT responses from the HC3 English dataset, which includes roughly 60K human answers and 27K ChatGPT answers for approximately 24K questions. This results in a total of around 87K question-answer examples.
· OIG: A subset of components from the Open Instruction Generalist dataset curated by LAION was used, including the grade-school-math-instructions, the poetry-to-songs, and the plot-screenplay-books-dialogue datasets. The selected subset results in a total of around 30K examples.
· Stanford Alpaca: The team included the dataset used to train the Stanford Alpaca model, which contains approximately 52K examples generated by OpenAI’s text-davinci-003 through the self-instruct process. It is worth noting that HC3, OIG, and Alpaca datasets are single-turn question answering while ShareGPT dataset is dialogue conversations.
· Anthropic HH: The team utilized the Anthropic HH dataset, which includes around 160K human-rated examples. Each example consists of a pair of responses from a chatbot, one of which is preferred by humans. The dataset provides both capabilities and additional safety protections for the model.
· OpenAI WebGPT: The OpenAI WebGPT dataset includes approximately 20K comparisons where each example comprises a question, a pair of model answers, and metadata. The answers are rated by humans with a preference score.
· OpenAI Summarization: The OpenAI summarization dataset contains approximately 93K examples, each example consisting of feedback from humans regarding the summarizations generated by a model. Human evaluators chose the superior summary from two options.
A comparison between Koala, ChatGPT and open source models like Alpaca can be seen in the following matrix:

Image Credit: BAIR
EasyLM
One of the key contributions of the Koala research was the open-source release of EasyLM, the framework used for fine-tuning the model. Conceptually, EasyLM is a solution designed to pre-train, fine-tune, evaluate, and serve LLMs in JAX/Flax. Leveraging JAX’s pjit functionality, EasyLM can scale up LLM training to hundreds of TPU/GPU accelerators.EasyLM is built on top of Hugginface’s transformers and datasets, providing a user-friendly and customizable codebase for training large language models without the complexity of many other frameworks. By utilizing JAX’s pjit utility, EasyLM can train large models that do not fit on a single accelerator by sharding the model weights and training data across multiple accelerators. Currently, EasyLM supports multiple TPU/GPU training in a single host as well as multi-host training on Google Cloud TPU Pods.
Koala, on the other hand, was trained on a single Nvidia DGX server equipped with 8 A100 GPUs. The training process took approximately 6 hours to complete for 2 epochs. This type of training run typically costs less than $100 on public cloud computing platforms using preemptible instances.
The open-source release of Koala came accompanied by an online demo and the code for preprocessing the training data.
Koala represents an interesting iteration of the LlaMA models and one that sheds some light on the viability of smaller open-source alternatives to ChatGPT-like models.