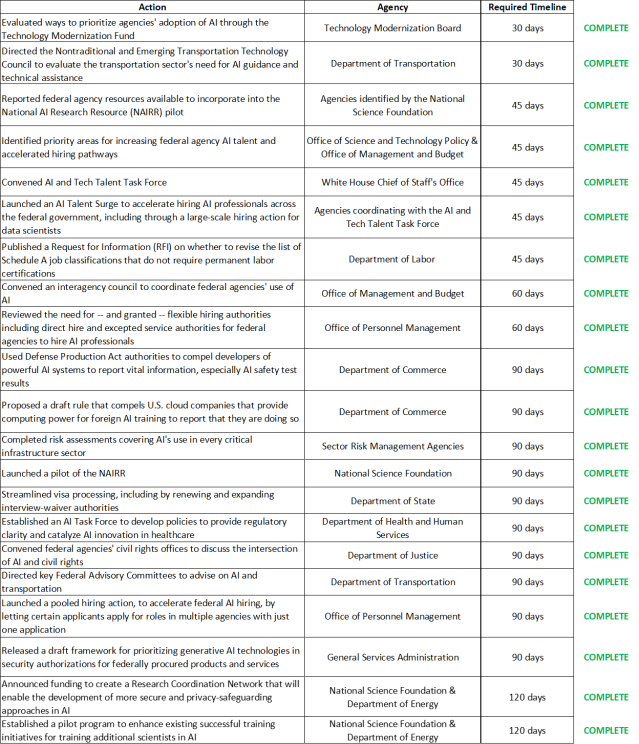
Biden-Harris Administration Announces Key AI Actions 180 Days Following President Biden’s Landmark Executive Order | The White House
Six months ago, President Biden issued a landmark Executive Order to ensure that America leads the way in seizing the promise and managing the risks of artificial intelligence (AI). Since then, agencies all across government have taken vital steps to manage AI’s safety and security risks...
Biden-
Six months ago, President Biden issued a landmark Executive Order to ensure that America leads the way in seizing the promise and managing the risks of artificial intelligence (AI). Since then, agencies all across government have taken vital steps to manage AI’s safety and security risks, protect Americans’ privacy, advance equity and civil rights, stand up for consumers and workers, promote innovation and competition, advance American leadership around the world, and more.
Today, federal agencies reported that they completed all of the 180-day actions in the E.O. on schedule, following their recent successes completing each 90-day, 120-day, and 150-day action on time. Agencies also progressed on other work tasked by the E.O. over longer timeframes.
Actions that agencies reported today as complete include the following:
Managing Risks to Safety and Security:
Over 180 days, the Executive Order directed agencies to address a broad range of AI’s safety and security risks, including risks related to dangerous biological materials, critical infrastructure, and software vulnerabilities. To mitigate these and other threats to safety, agencies have:
- Established a framework for nucleic acid synthesis screening to help prevent the misuse of AI for engineering dangerous biological materials. This work complements in-depth study by the Department of Homeland Security (DHS), Department of Energy (DOE) and Office of Science and Technology Policy on AI’s potential to be misused for this purpose, as well as a DHS report that recommended mitigations for the misuse of AI to exacerbate chemical and biological threats. In parallel, the Department of Commerce has worked to engage the private sector to develop technical guidance to facilitate implementation. Starting 180 days after the framework is announced, agencies will require that grantees obtain synthetic nucleic acids from vendors that screen.
- Released for public comment draft documents on managing generative AI risks, securely developing generative AI systems and dual-use foundation models, expanding international standards development in AI, and reducing the risks posed by AI-generated content. When finalized, these documents by the National Institute of Standards and Technology (NIST) will provide additional guidance that builds on NIST’s AI Risk Management Framework, which offered individuals, organizations, and society a framework to manage AI risks and has been widely adopted both in the U.S. and globally.
- Developed the first AI safety and security guidelines for critical infrastructure owners and operators. These guidelines are informed by the completed work of nine agencies to assess AI risks across all sixteen critical infrastructure sectors.
- Launched the AI Safety and Security Board to advise the Secretary of Homeland Security, the critical infrastructure community, other private sector stakeholders, and the broader public on the safe and secure development and deployment of AI technology in our nation’s critical infrastructure. The Board’s 22 inaugural members include representatives from a range of sectors, including software and hardware company executives, critical infrastructure operators, public officials, the civil rights community, and academia.
- Piloted new AI tools for identifying vulnerabilities in vital government software systems. The Department of Defense (DoD) made progress on a pilot for AI that can find and address vulnerabilities in software used for national security and military purposes. Complementary to DoD’s efforts, DHS piloted different tools to identify and close vulnerabilities in other critical government software systems that Americans rely on every hour of every day.
Standing up for Workers, Consumers, and Civil Rights
The Executive Order directed bold steps to mitigate other risks from AI—including risks to workers, to consumers, and to Americans’ civil rights—and ensure that AI’s development and deployment benefits all Americans. Today, agencies reported that they have:
- Developed bedrock principles and practices for employers and developers to build and deploy AI safely and in ways that empower workers. Agencies all across government are now starting work to establish these practices as requirements, where appropriate and authorized by law, for employers that receive federal funding.
- Released guidance to assist federal contractors and employers comply with worker protection laws as they deploy AI in the workplace. The Department of Labor (DOL) developed a guide for federal contractors and subcontractors to answer questions and share promising practices to clarify federal contractors’ legal obligations, promote equal employment opportunity, and mitigate the potentially harmful impacts of AI in employment decisions. DOL also provided guidance regarding the application of the Fair Labor Standards Act and other federal labor standards as employers increasingly use of AI and other automated technologies in the workplace.
- Released resources for job seekers, workers, and tech vendors and creators on how AI use could violate employment discrimination laws. The Equal Employment Opportunity Commission’s resources clarify that existing laws apply the use of AI and other new technologies in employment just as they apply to other employment practices.
- Issued guidance on AI’s nondiscriminatory use in the housing sector. In two guidance documents, the Department of Housing and Urban Development affirmed that existing prohibitions against discrimination apply to AI’s use for tenant screening and advertisement of housing opportunities, and it explained how deployers of AI tools can comply with these obligations.
- Published guidance and principles that set guardrails for the responsible and equitable use of AI in administering public benefits programs. The Department of Agriculture’s guidance explains how State, local, Tribal, and territorial governments should manage risks for uses of AI and automated systems in benefits programs such as SNAP. The Department of Health and Human Services (HHS) released a plan with guidelines on similar topics for benefits programs it oversees. Both agencies’ documents prescribe actions that align with the Office of Management and Budget’s policies, published last month, for federal agencies to manage risks in their own use of AI and harness AI’s benefits.
- Announced a final rule clarifying that nondiscrimination requirements in health programs and activities continue to apply to the use of AI, clinical algorithms, predictive analytics, and other tools. Specifically, the rule applies the nondiscrimination principles under Section 1557 of the Affordable Care Act to the use of patient care decision support tools in clinical care, and it requires those covered by the rule to take steps to identify and mitigate discrimination when they use AI and other forms of decision support tools for care.
- Developed a strategy for ensuring the safety and effectiveness of AI deployed in the health care sector. The strategy outlines rigorous frameworks for AI testing and evaluation, and it outlines future actions for HHS to promote responsible AI development and deployment.
Harnessing AI for Good
President Biden’s Executive Order also directed work to seize AI’s enormous promise, including by advancing AI’s use for scientific research, deepening collaboration with the private sector, and piloting uses of AI. Over the past 180 days, agencies have done the following:
- Announced DOE funding opportunities to support the application of AI for science, including energy-efficient AI algorithms and hardware.
- Prepared convenings for the next several months with utilities, clean energy developers, data center owners and operators, and regulators in localities experiencing large load growth. Today, DOE announced new actions to assess the potential energy opportunities and challenges of AI, accelerate deployment of clean energy, and advance AI innovation to manage the growing energy demand of AI.
- Launched pilots, partnerships, and new AI tools to address energy challenges and advance clean energy. For example, DOE is piloting AI tools to streamline permitting processes and improving siting for clean energy infrastructure, and it has developed other powerful AI tools with applications at the intersection of energy, science, and security. Today, DOE also published a report outlining opportunities AI brings to advance the clean energy economy and modernize the electric grid.
- Initiated a sustained effort to analyze the potential risks that deployment of AI may pose to the grid. DOE has started the process of convening energy stakeholders and technical experts over the coming months to collaboratively assess potential risks to the grid, as well as ways in which AI could potentially strengthen grid resilience and our ability to respond to threats—building off a new public assessment.
- Authored a report on AI’s role in advancing scientific research to help tackle major societal challenges, written by the President’s Council of Advisors on Science and Technology.
Bringing AI Talent into Government
The AI and Tech Talent Task Force has made substantial progress on hiring through the AI Talent Surge. Since President Biden signed the E.O., federal agencies have hired over 150 AI and AI-enabling professionals and, along with the tech talent programs, are on track to hire hundreds by Summer 2024. Individuals hired thus far are already working on critical AI missions, such as informing efforts to use AI for permitting, advising on AI investments across the federal government, and writing policy for the use of AI in government.
- The General Services Administration has onboarded a new cohort of Presidential Innovation Fellows (PIF) and also announced their first-ever PIF AI cohort starting this summer.
- DHS has launched the DHS AI Corps, which will hire 50 AI professionals to build safe, responsible, and trustworthy AI to improve service delivery and homeland security.
- The Office of Personnel Management has issued guidance on skills-based hiring to increase access to federal AI roles for individuals with non-traditional academic backgrounds.
- For more on the AI Talent Surge’s progress, read its report to the President. To explore opportunities, visit Join the National AI Talent Surge
The table below summarizes many of the activities that federal agencies have completed in response to the Executive Order.
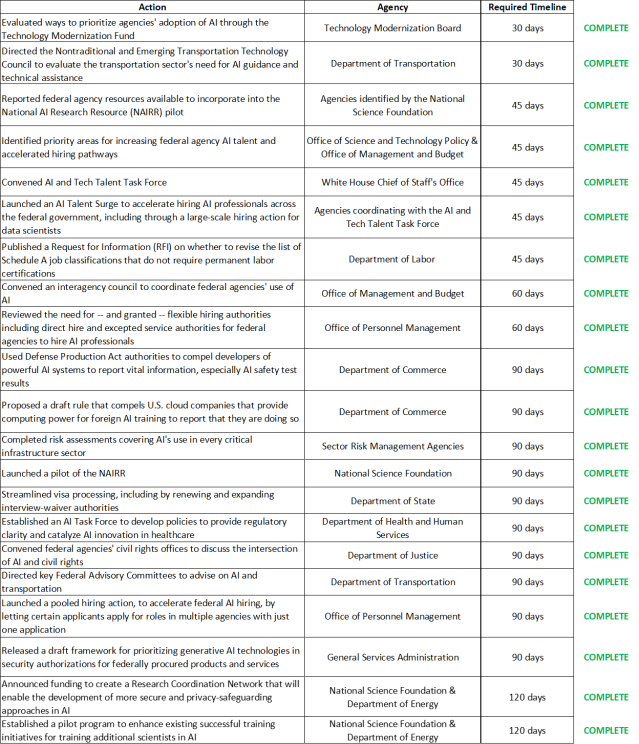


