
Nvidia's Jensen Huang says AI hallucinations are solvable, artificial general intelligence is 5 years away | TechCrunch
Artificial general intelligence (AGI) -- often referred to as "strong AI," "full AI," "human-level AI" or "general intelligent action" -- represents a

Nvidia’s Jensen Huang says AI hallucinations are solvable, artificial general intelligence is 5 years away
Haje Jan Kamps @Haje / 5:13 PM EDT•March 19, 2024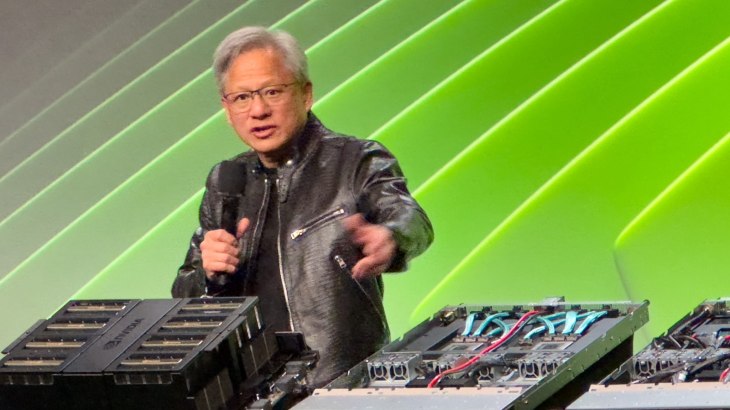
Image Credits: Haje Jan Kamps(opens in a new window)
Artificial general intelligence (AGI) — often referred to as “strong AI,” “full AI,” “human-level AI” or “general intelligent action” — represents a significant future leap in the field of artificial intelligence. Unlike narrow AI, which is tailored for specific tasks, such as detecting product flaws, summarizing the news, or building you a website, AGI will be able to perform a broad spectrum of cognitive tasks at or above human levels. Addressing the press this week at Nvidia’s annual GTC developer conference, CEO Jensen Huang appeared to be getting really bored of discussing the subject — not least because he finds himself misquoted a lot, he says.
The frequency of the question makes sense: The concept raises existential questions about humanity’s role in and control of a future where machines can outthink, outlearn and outperform humans in virtually every domain. The core of this concern lies in the unpredictability of AGI’s decision-making processes and objectives, which might not align with human values or priorities (a concept explored in-depth in science fiction since at least the 1940s). There’s concern that once AGI reaches a certain level of autonomy and capability, it might become impossible to contain or control, leading to scenarios where its actions cannot be predicted or reversed.
When sensationalist press asks for a timeframe, it is often baiting AI professionals into putting a timeline on the end of humanity — or at least the current status quo. Needless to say, AI CEOs aren’t always eager to tackle the subject.
Huang, however, spent some time telling the press what he does think about the topic. Predicting when we will see a passable AGI depends on how you define AGI, Huang argues, and draws a couple of parallels: Even with the complications of time zones, you know when New Year happens and 2025 rolls around. If you’re driving to the San Jose Convention Center (where this year’s GTC conference is being held), you generally know you’ve arrived when you can see the enormous GTC banners. The crucial point is that we can agree on how to measure that you’ve arrived, whether temporally or geospatially, where you were hoping to go.
“If we specified AGI to be something very specific, a set of tests where a software program can do very well — or maybe 8% better than most people — I believe we will get there within 5 years,” Huang explains. He suggests that the tests could be a legal bar exam, logic tests, economic tests or perhaps the ability to pass a pre-med exam. Unless the questioner is able to be very specific about what AGI means in the context of the question, he’s not willing to make a prediction. Fair enough.
AI hallucination is solvable
In Tuesday’s Q&A session, Huang was asked what to do about AI hallucinations — the tendency for some AIs to make up answers that sound plausible but aren’t based in fact. He appeared visibly frustrated by the question, and suggested that hallucinations are solvable easily — by making sure that answers are well-researched.“Add a rule: For every single answer, you have to look up the answer,” Huang says, referring to this practice as “retrieval-augmented generation,” describing an approach very similar to basic media literacy: Examine the source and the context. Compare the facts contained in the source to known truths, and if the answer is factually inaccurate — even partially — discard the whole source and move on to the next one. “The AI shouldn’t just answer; it should do research first to determine which of the answers are the best.”
For mission-critical answers, such as health advice or similar, Nvidia’s CEO suggests that perhaps checking multiple resources and known sources of truth is the way forward. Of course, this means that the generator that is creating an answer needs to have the option to say, “I don’t know the answer to your question,” or “I can’t get to a consensus on what the right answer to this question is,” or even something like “Hey, the Super Bowl hasn’t happened yet, so I don’t know who won.”