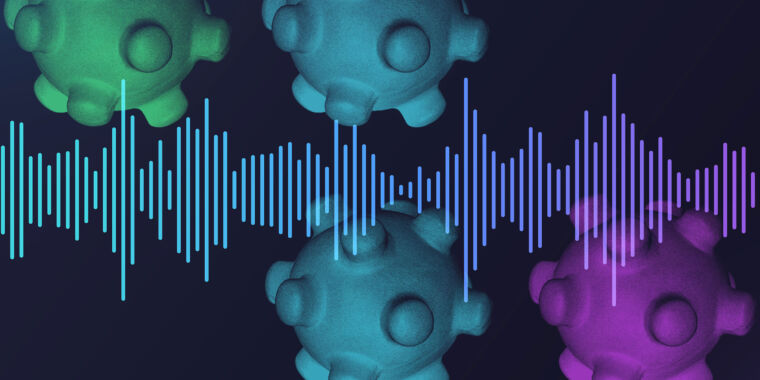
Fake AI “podcasters” are reviewing my book and it’s freaking me out
NotebookLM's "Audio Summaries" show a more personable future for AI-generated content.
Fake AI “podcasters” are reviewing my book and it’s freaking me out
NotebookLM's "Audio Summaries" show a more personable future for AI-generated content.
Kyle Orland - 9/23/2024, 11:40 AM
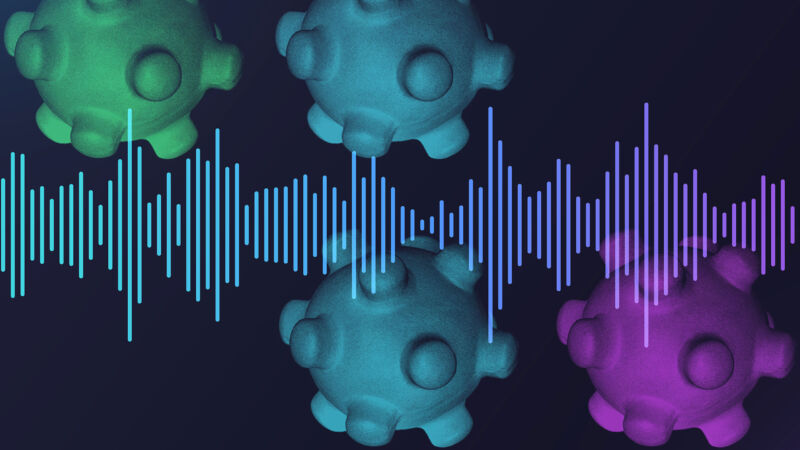
Enlarge / Hey, welcome back to "Talkin'Minesweeper," the podcast where AI hosts discuss a book about Minesweeper!
Aurich Lawson | Boss Fight Books
66
Further Reading
How Bill Gates’ Minesweeper addiction helped lead to the XboxAs someone who has been following the growth of generative AI for a while now, I know that the technology can be pretty good (if not quite human-level) at quickly summarizing complex documents into a more digestible form. But I still wasn't prepared for how disarmingly compelling it would be to listen to Google's NotebookLM condense my recent book about Minesweeper into a tight, 12.5-minute, podcast-style conversation between two people who don't exist.
There are still enough notable issues with NotebookLM's audio output to prevent it from fully replacing professional podcasters any time soon. Even so, the podcast-like format is an incredibly engaging and endearing way to take in complex information and points to a much more personable future for generative AI than the dry back-and-forth of a text-based chatbot.
Hey! Listen!
Listen to NotebookLM's 12.5-minute summary of my Minesweeper book using the player above.
Google's NotebookLM launched over a year ago as "a virtual research assistant that can summarize facts, explain complex ideas, and brainstorm new connections—all based on the sources you select." Just last week, though, Google added the new "Audio Overview" feature that it's selling as "a new way to turn your documents into engaging audio discussions."
Google doesn't use the word "podcast" anywhere in that announcement, instead talking up audio creations that "summarize your material, make connections between topics, and banter back and forth." But Wharton AI professor Ethan Mollick correctly referred to the style as a "podcast" in a recent social media post sharing a NotebookLM Audio Overview of his book. Mollick called these Audio Summaries "the current best 'wow this is amazing & useful' demo of AI" and "unnerving, too," and we agree on both counts.
Inspired by Mollick's post, I decided to feed my own book into NotebookLM to see what its virtual "podcasters" would make of 30,000 or so words about '90s Windows gaming classic Minesweeper (believe it or not, I could have written much more). Just a few minutes later, I was experiencing a reasonable facsimile of what it would be like if I was featured on NPR's Pop Culture Happy Hour or a similar banter-filled podcast.
Just the facts?
NotebookLM's summary hits on all the book's major sections: the pre-history of the games that inspired Minesweeper; the uphill battle for the Windows Entertainment Pack at a business-focused Microsoft of the '90s; the moral panic over the game's pre-installation on millions of business and government computers; and the surprising cheating controversies that surrounded the game's competitive scene.
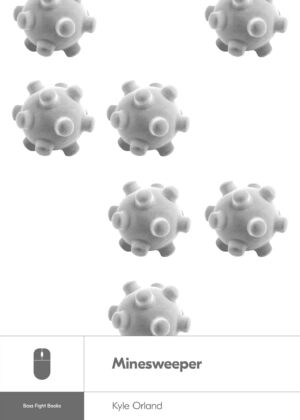
Enlarge / Why read ~30,000 words about Minesweeper when you can listen to two fake people banter for a few minutes instead?
Boss Fight Books
Sure, I could quibble about which specific bits the summary decided to focus on and/or leave out (maybe feeding different chapters individually would have led to more detail in the collected summaries). But anyone listening to this "podcast" would get the same general overview of my book that they would listening to one of the many actual podcasts that I did after the book launched.
While there weren't any full-blown, whole-cloth hallucinations in NotebookLM's summary "podcast," there were a few points where it got small details wrong or made assumptions that weren't supported in the text. Discussing Minesweeper predecessor Mined-Out, for instance, NotebookLM's audio summary says, "So this is where those squares and flags start to come into play..." even though Mined-Out had neither feature.
Then there's the portion where the summary-cast mentions a senator who called Minesweeper "a menace to the republic," repeating the quote for emphasis. That definitely captures the spirit of Senator Lauch Faircloth's tirade against Minesweeper and other games being pre-installed on government computers. In the "podcast" context, though, it sounds like the voices are putting words in Faircloth's mouth by sharing a direct quote.
Small, overzealous errors like these—and a few key bits of the book left out of the podcast entirely—would give me pause if I were trying to use a NotebookLM summary as the basis for a scholarly article or piece of journalism. But I could see using a summary like this to get some quick Cliff's Notes-style grounding on a thick tome I didn't have the time or inclination to read fully. And, unlike poring through Cliff's Notes, the pithy, podcast-style format would actually make for enjoyable background noise while out on a walk or running errands.
It’s all in the delivery
It's that naturalistic, bantering presentation that makes NotebookLM's new feature stand out from other AI products that generate capable text summaries. I felt like I was eavesdropping on two people who just happened to be discussing my book in a cafe, except those people don't actually exist (and were probably algorithmically designed to praise the book).
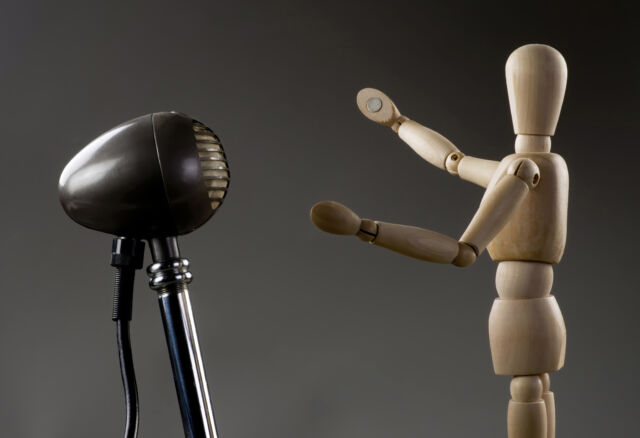
Enlarge / Is this thing on?
Getty Images
Right from the start, I was tickled by the way one "podcast host" described the book as a tale from "the land of floppy disks and dial-up modems" (a phrase I did not use in the book). That same voice goes on to tease "a bit of Bill Gates sneaking around the Microsoft office," up front, hinting at my absolute favorite anecdote from the book before fully exploring it later in the summary.
When they do get to that anecdote, the fake podcast hosts segue in with what feels like a natural conversational structure:
Voice 1: It's hard to deny the impact of something when your own CEO is secretly hooked.
Voice 2: Wait, are we talking about Bill Gates?
The back-and-forth style of the two-person "podcast" format allows for some entertaining digressions from the main point of the book, too. When discussing the wormy movie-star damsel-in-distress featured in Minesweeper predecessor Mined-Out, for instance, the AI summarizers seem to get a little distracted:
Voice 1: I have to ask, what kind of movies does a worm even star in?
Voice 2: I'm afraid that detail has been lost to the sands of gaming history.
Then there's the casual way the two "hosts" bring up the improved versions of Minesweeper that were crafted to fix problems with Microsoft's original:
Voice 1: So eventually the community came up with a more elegant solution.
Voice 2: Let me guess. They created a new version of Minesweeper.
Voice 1: Exactly.
Voice 2: Called it a day on the old one.
The two-person format helps foster a gentle, easy rhythm to the presentation of dense information, with natural-sounding pauses and repetition that help emphasize key points. When one ersatz podcaster talks about the phenomenon of "this incredibly addictive puzzle game [being] pre-installed on practically every computer," for instance, the other voice can answer back with the phrase "on every computer" with just the right amount of probing interest. Or when one AI voice intones that "it was discovered that the original Minesweeper had a flaw in how it generated random boards," the other voice jumps in and exclaims "A flaw!" with pitch-perfect timing and a sense of surprise.
Wait, are we talking about Bill Gates?
NotebookLM podcast voice
There are some problems with this back-and-forth style, though. For one, both voices seem to alternate between the "I read the book" role and the "I'm surprised at these book facts you're sharing" role, making it hard to feel like either one is genuine. For another, the sheer volume of surprised reactions (a partial sample: "What? No! Wooooow! You're kidding! No way! You're blowing my mind here!") can get a little grating. And then there are the sentences that pause at the wrong points or the bits of laughter that feel like an editor chopped them off prematurely.
Still, when one fake podcast voice cooed, "Oh, do tell!" in response to the idea of controversy in competitive Minesweeper, it set off the same parasocial relationship buttons that a good, authentic podcast can (while also effectively flattering my sense of authorial ego).
After listening to NotebookLM's summary of my own book, I can easily envision a near future where these "fake" podcasts become a part of my real podcast diet, especially for books or topics that are unlikely to get professional interest from human podcasters. By repackaging generative AI text into a "just two people chatting" format, Google has put a much more amiable face on what can sometimes seem like a dehumanizing technology.