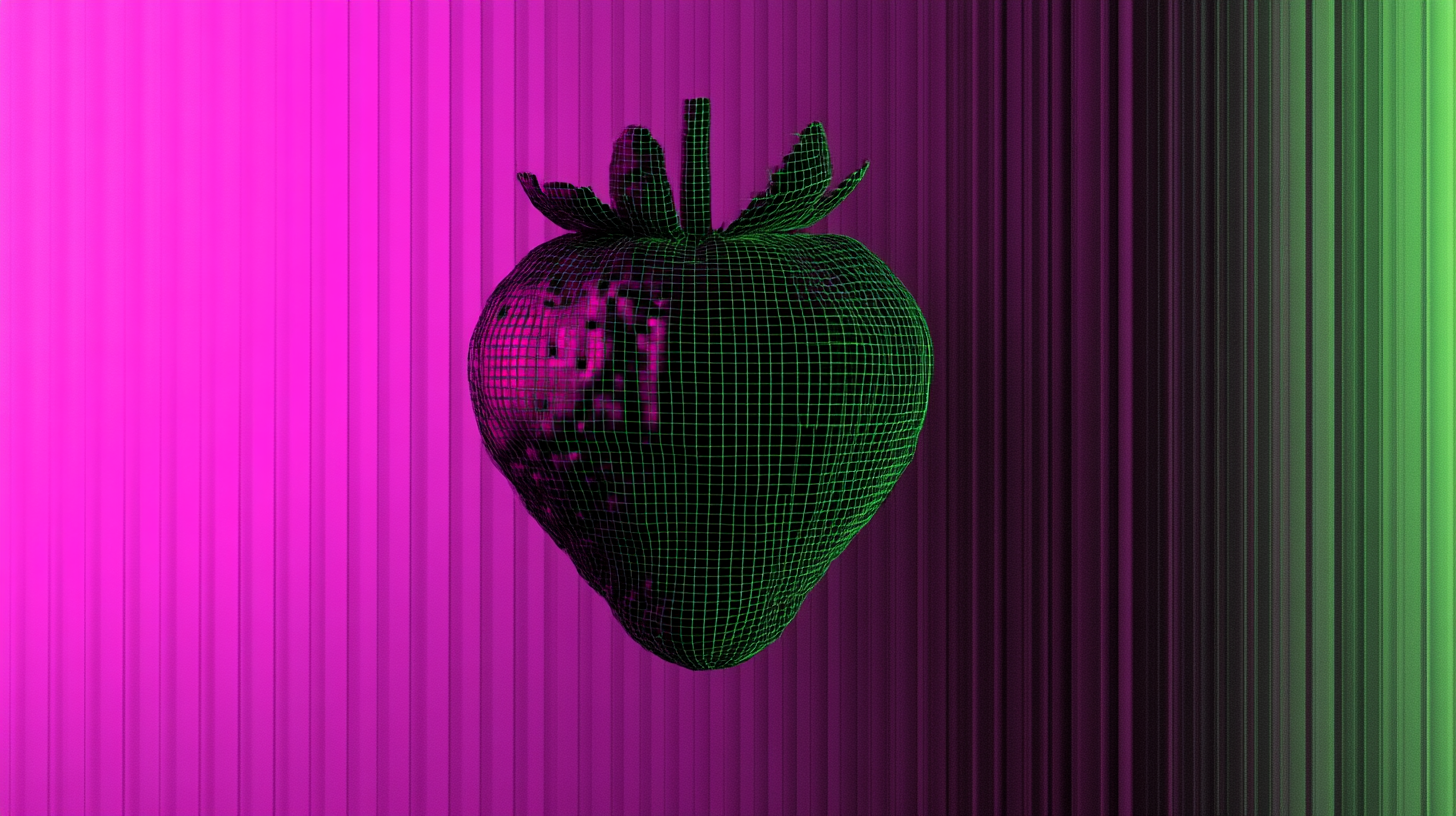
OpenAI's Strawberry AI is reportedly the secret sauce behind next-gen Orion language model
OpenAI is developing two new AI models that could significantly advance the field. "Strawberry" aims to solve complex math and programming problems better than current systems, while "Orion" aims to surpass GPT-4's capabilities with the help of Strawberry.

AI in practice
Aug 27, 2024
OpenAI's Strawberry AI is reportedly the secret sauce behind next-gen Orion language model
Midjourney prompted by THE DECODER
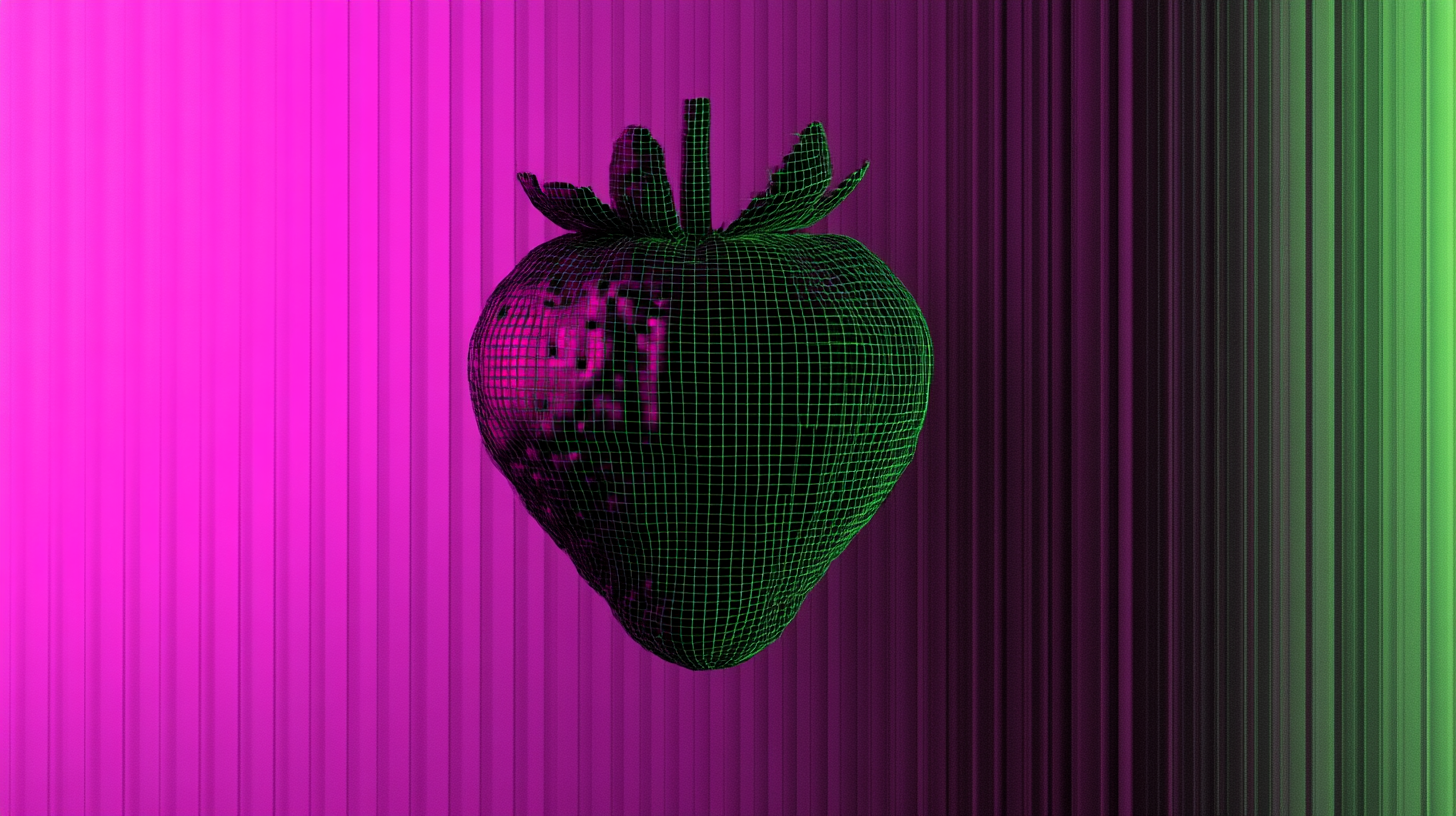
Matthias Bastian
Online journalist Matthias is the co-founder and publisher of THE DECODER. He believes that artificial intelligence will fundamentally change the relationship between humans and computers.
Profile
OpenAI is developing two new AI models that could significantly advance the field. "Strawberry" aims to solve complex math and programming problems better than current systems, while "Orion" aims to surpass GPT-4's capabilities with the help of Strawberry.
According to The Information, citing two people involved in the project, OpenAI might release a chatbot version of Strawberry as early as this fall, possibly as part of ChatGPT.
Strawberry is designed to tackle previously unseen math problems and optimize programming tasks. Its enhanced logic should allow it to solve language-related challenges more effectively when given sufficient time to "think."
Agent-based AI systems based on Strawberry
In internal demonstrations, Strawberry reportedly solved the New York Times word puzzle "Connections." The model could also serve as a foundation for more advanced AI systems capable of not just generating content, but taking action.
Reuters reported that OpenAI has already tested an AI internally that scored over 90 percent on the MATH benchmark, a collection of math mastery tasks. This is likely Strawberry, which has also been presented to national security officials, according to The Information.
Internal OpenAI documents describe plans to use Strawberry models for autonomous internet searches, enabling the AI to plan ahead and conduct in-depth research.
The Information notes that it's uncertain whether Strawberry will launch this year. If released, it would be a distilled version of the original model, delivering similar performance with less computational power – a technique OpenAI has also used for GPT-4 variants since the original model was released in March 2023.
OpenAI's approach reportedly resembles the "Self-Taught Reasoner" (STaR) method introduced by Stanford researchers, which aims to improve AI systems' reasoning abilities.
Former OpenAI chief researcher Ilya Sutskever, who has since founded his own startup focused on secure super AI, is said to have provided the idea and basis for Strawberry.