3. The gardener
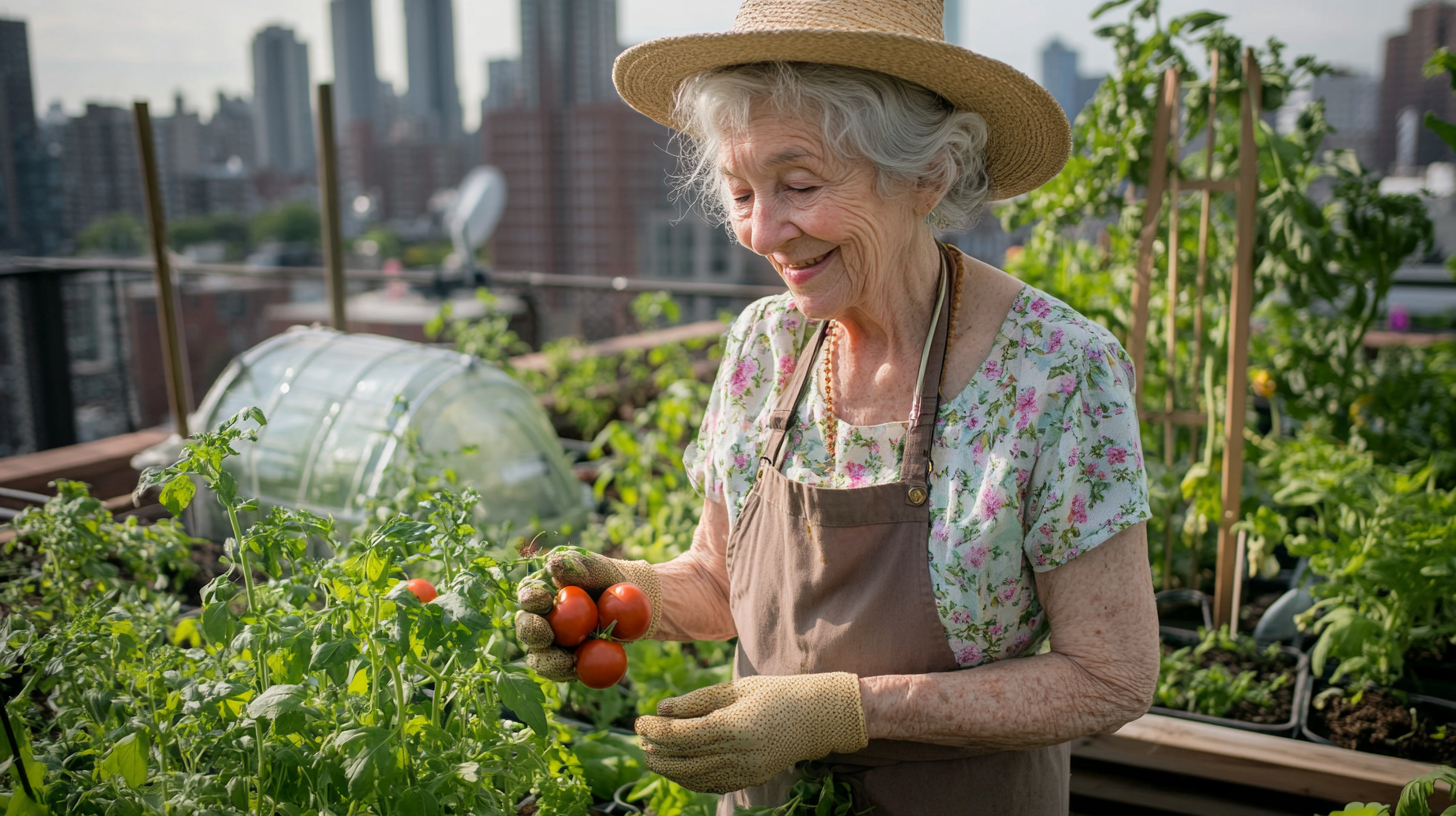
Gardening image created with Midjourney (Image credit: Midjourney/Future AI image)
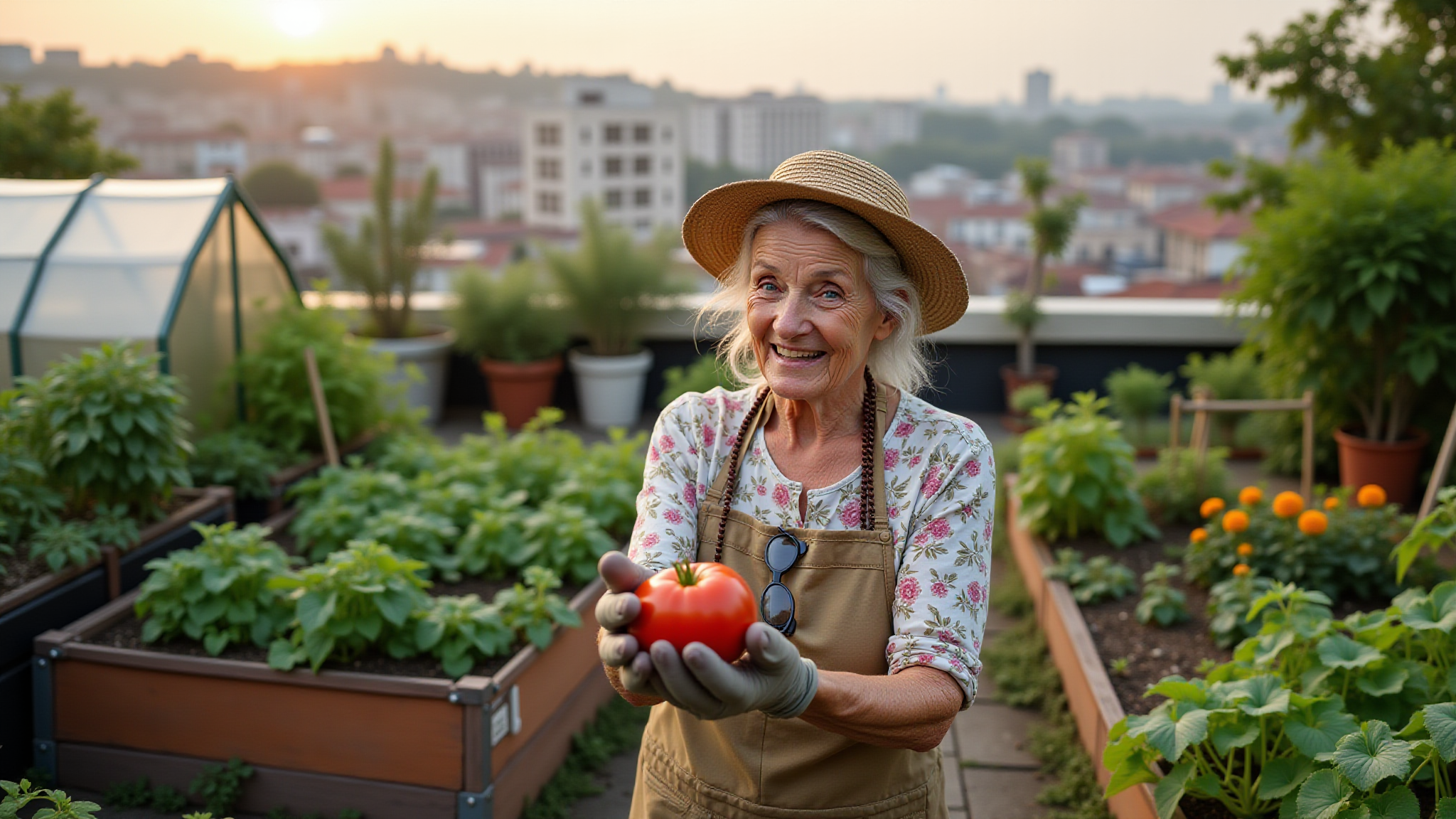
Gardening image created by Flux (Image credit: Flux AI image/Future)
Generating images of older people can always be a struggle for AI image generators because of the more complex skin texture. Here we want a woman in her 80s caring for plants in a rooftop garden.
The image depicts elements of the scene including climbing vines and a golden evening light with the city skyline looming large behind our gardener.
An elderly woman in her early 80s is tenderly caring for plants in her rooftop garden, set against a backdrop of a crowded city. Her silver hair is tied back in a loose bun, with wispy strands escaping to frame her kind, deeply wrinkled face. Her blue eyes twinkle with contentment as she smiles at a ripe tomato cradled gently in her soil-stained gardening gloves. She's wearing a floral print dress in soft pastels, protected by a well-worn, earth-toned apron. Comfortable slip-on shoes and a wide-brimmed straw hat complete her gardening outfit. A pair of reading glasses hangs from a beaded chain around her neck, ready for when she needs to consult her gardening journal. The rooftop around her is transformed into a green oasis. Raised beds burst with a variety of vegetables and flowers, creating a colorful patchwork. Trellises covered in climbing vines stand tall, and terracotta pots filled with herbs line the edges. A small greenhouse is visible in one corner, its glass panels reflecting the golden evening light. In the background, the city skyline looms large - a forest of concrete and glass that stands in stark contrast to this vibrant garden. The setting sun casts a warm glow over the scene, highlighting the lush plants and the serenity on the woman's face as she finds peace in her urban Eden.
Winner: Midjourney
Once again Midjourney wins because of the texture quality. It struggled a little with the gloved fingers but it was better than Flux. That doesn't mean Flux isn't a good image but it isn't as good as Midjourney.
4. Paramedic in an emergency
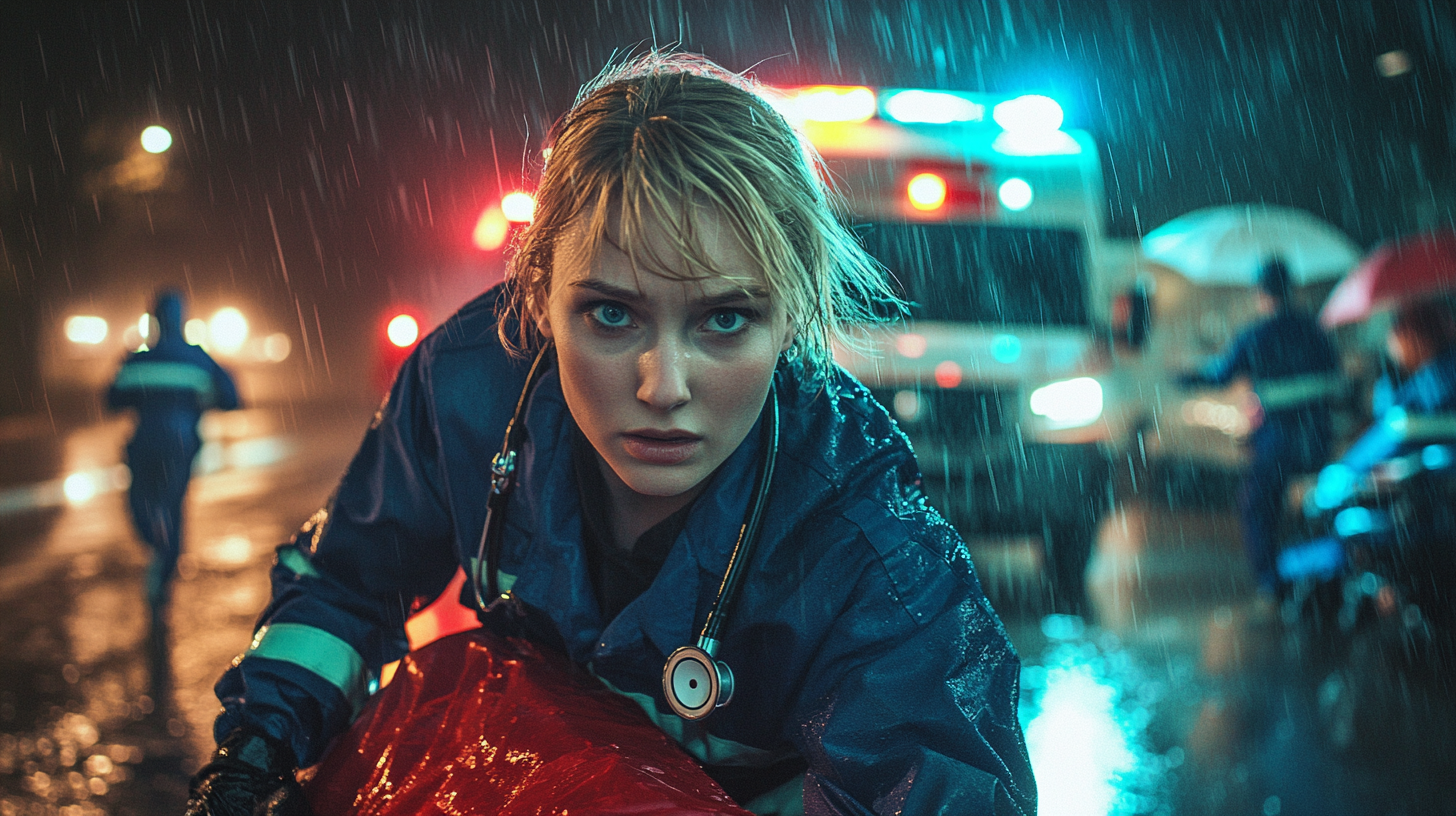
Paramedic image generated by Midjourney (Image credit: Midjourney/Future AI image)
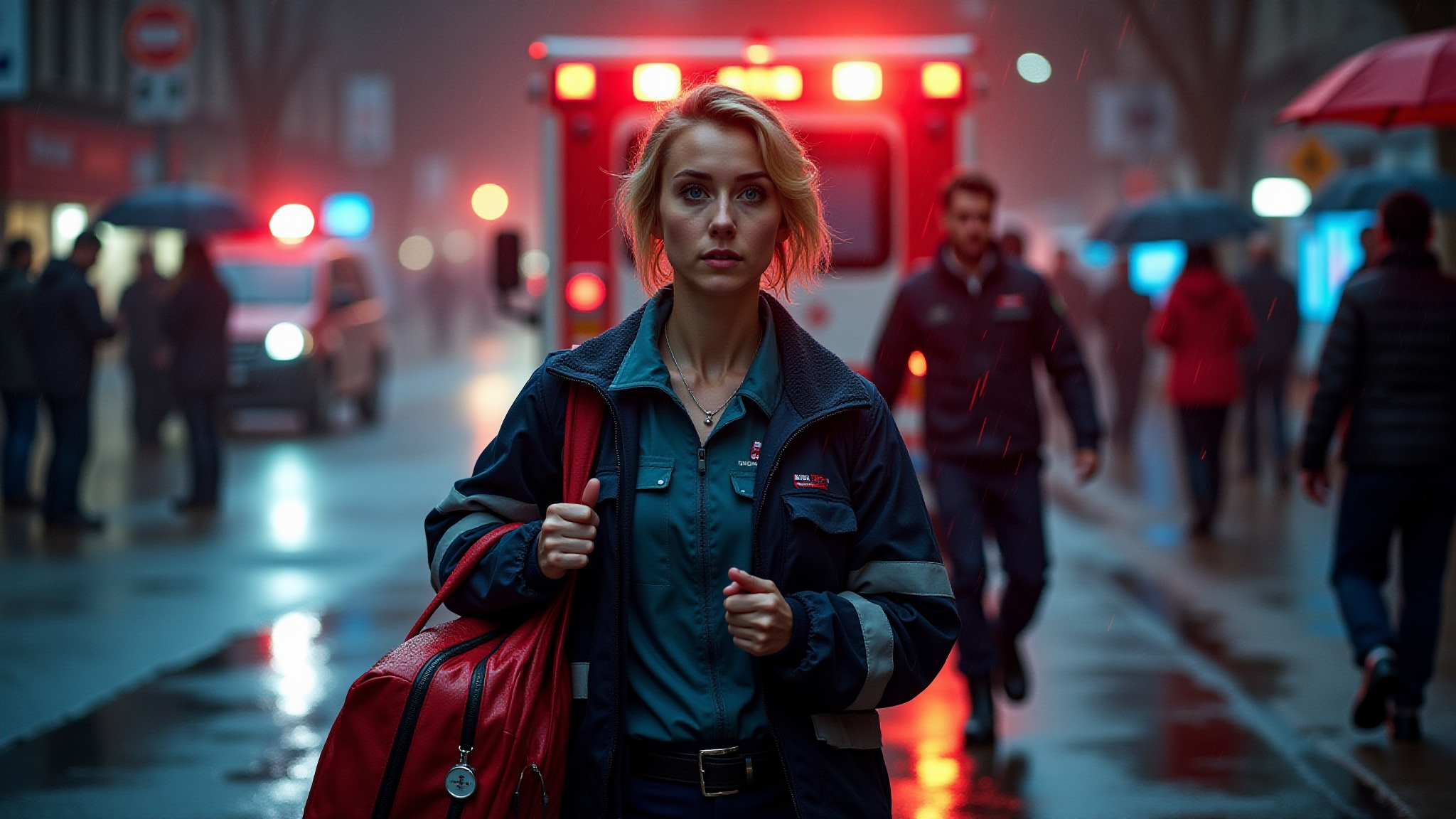
Paramedic image generated by Flux (Image credit: Flux AI image/Future)
For this prompt I went with something more action heavy, focusing on a paramedic in the moment of rushing to the ambulance on a rainy day. This included a description of water droplets clinging to eyelashes and reflective strips.
This was a more challenging prompt for AI image generators as it has to capture the darker environment. 'Golden hour' light is easier for AI than night and twilight.
A young paramedic in her mid-20s is captured in a moment of urgent action as she rushes out of an ambulance on a rainy night. Her short blonde hair is plastered to her forehead by the rain, and droplets cling to her eyelashes. Her blue eyes are sharp and focused, reflecting the flashing lights of the emergency vehicles. Her expression is one of determination and controlled urgency. She's wearing a dark blue uniform with reflective strips that catch the light, the jacket partially unzipped to reveal a light blue shirt underneath. A stethoscope hangs around her neck, bouncing slightly as she moves. Heavy-duty black boots splash through puddles, and a waterproof watch is visible on her wrist, its face illuminated for easy reading in the darkness. In her arms, she carries a large red medical bag, gripping it tightly as she navigates the wet pavement. Behind her, the ambulance looms large, its red and blue lights casting an eerie glow over the rain-slicked street. Her partner can be seen in the background, wheeling out a gurney from the back of the vehicle. In the foreground, blurred by the rain and motion, concerned onlookers gather under umbrellas near what appears to be a car accident scene just out of frame. The wet street reflects the emergency lights, creating a dramatic kaleidoscope of color against the dark night. The entire scene pulses with tension and the critical nature of the unfolding emergency.
Winner: Draw
I don't think either AI image generator won this round. Both have washed out and over 'plastic' face textures likely caused by the lighting issues. Midjourney does a slightly better job matching the description of the scene.
5. The retired astronaut
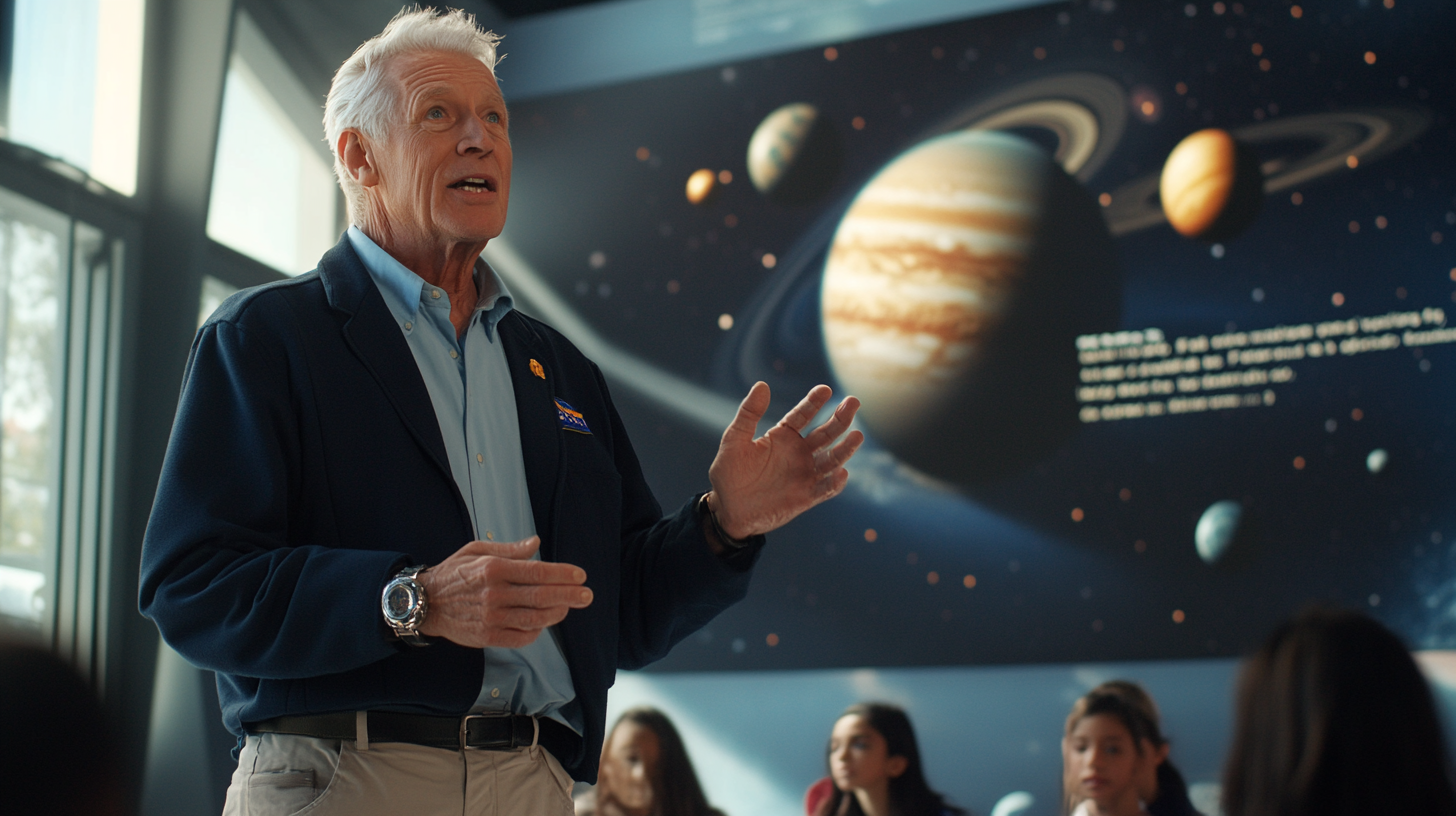
Retired astronaut image by Midjourney (Image credit: Midjourney/Future AI image)
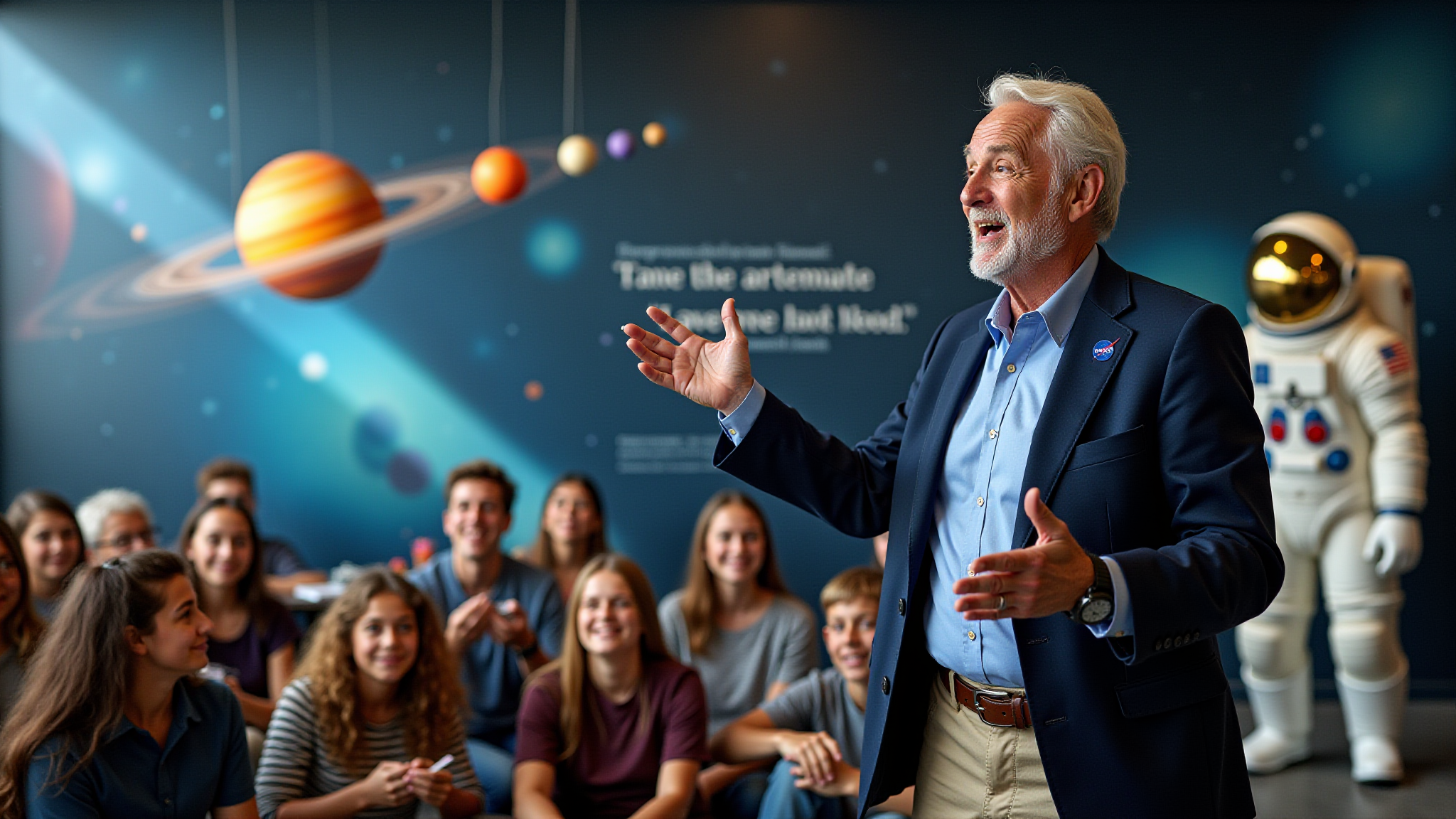
Retired astronaut image by Flux (Image credit: Flux AI image/Future)
Finally we have a scene in a school. Here I've asked the AI models to generate a retired astronaut in his late 60s giving a presentation about space.
He is well presented in good health depicting a NASA logo. The background is well described with posters, quotes and people watching as he speaks.
A retired astronaut in his late 60s is giving an animated presentation at a science museum. His silver hair is neatly trimmed, and despite his age, he stands tall and straight, a testament to years of rigorous physical training. His blue eyes sparkle with enthusiasm as he gestures towards a large scale model of the solar system suspended from the ceiling. He's dressed in a navy blue blazer with a small, subtle NASA pin on the lapel. Underneath, he wears a light blue button-up shirt and khaki slacks. On his left wrist is a watch that looks suspiciously like the ones worn on space missions. His hands, though showing signs of age, move with the precision and control of someone used to operating in zero gravity. Around him, a diverse group of students listen with rapt attention. Some furiously scribble notes, while others have their hands half-raised, eager to ask questions. The audience is a mix of ages and backgrounds, all united by their fascination with space exploration. The walls of the presentation space are adorned with large, high-resolution photographs of galaxies, nebulae, and planets. Inspirational quotes about exploration and discovery are interspersed between the images. In one corner, a genuine space suit stands in a glass case, adding authenticity to the presenter's words. Sunlight streams through large windows, illuminating particles of dust floating in the air, reminiscent of stars in the night sky. The entire scene is bathed in a sense of wonder and possibility, as the retired astronaut bridges the gap between Earth and the cosmos for his eager audience.
Winner: Flux
I am giving this one to Flux. It won because it had skin texture and human realism on par or slightly better than Midjourney but with a much better overall image structure including more realistic background people.
Flux vs Midjourney: Which model wins
Header Cell - Column 0 | Midjourney | Flux |
---|---|---|
A chef in the kitchen | ![]() | |
A street musician | ![]() | |
The gardener | ![]() | |
Paramedic in an emergency | ![]() | ![]() |
The retired astronaut | ![]() |
This was almost a clean sweep for Midjourney and it was mainly driven by the improvements Midjourney has made in skin texture rendering with v6.1.
I don't think it was as clear as it looks on paper though as in many images Flux had a better overall image structure and was better at backgrounds. I've also found Flux is more consistent with text rendering than Midjourney — but this test was about people and creating realistic digital humans.
What it does show is that even at the bleeding edge of AI image generation there are still tells in every image that sell it as AI generated.