Wifi can read through walls
UCSB researchers’ new method enables high-quality imaging of still objects with WiFi by using the Geometrical Theory of Diffraction and the corresponding Keller cones to trace edges of the objects.
Mostofi Lab’s latest research makes significant progress in imaging still objects with WiFi, by exploiting the interaction of the edges with the incoming waves using the Geometrical Theory of Diffraction (GTD). This further enables the first demonstration of WiFi reading through walls.
SCIENCE + TECHNOLOGY
September 11, 2023
Wifi can read through walls
Sonia FernandezResearchers in UC Santa Barbara professor Yasamin Mostofi’s lab have proposed a new foundation that can enable high-quality imaging of still objects with only WiFi signals. Their method uses the Geometrical Theory of Diffraction and the corresponding Keller cones to trace edges of the objects. The technique has also enabled, for the first time, imaging, or reading, the English alphabet through walls with WiFi, a task deemed too difficult for WiFi due to the complex details of the letters.
For more details on this technology, check their video at
“Imaging still scenery with WiFi is considerably challenging due to the lack of motion,” said Mostofi, a professor of electrical and computer engineering. “We have then taken a completely different approach to tackle this challenging problem by focusing on tracing the edges of the objects instead.” The proposed methodology and experimental results appeared in the Proceedings of the 2023 IEEE National Conference on Radar (RadarConf) on June 21, 2023.
Image
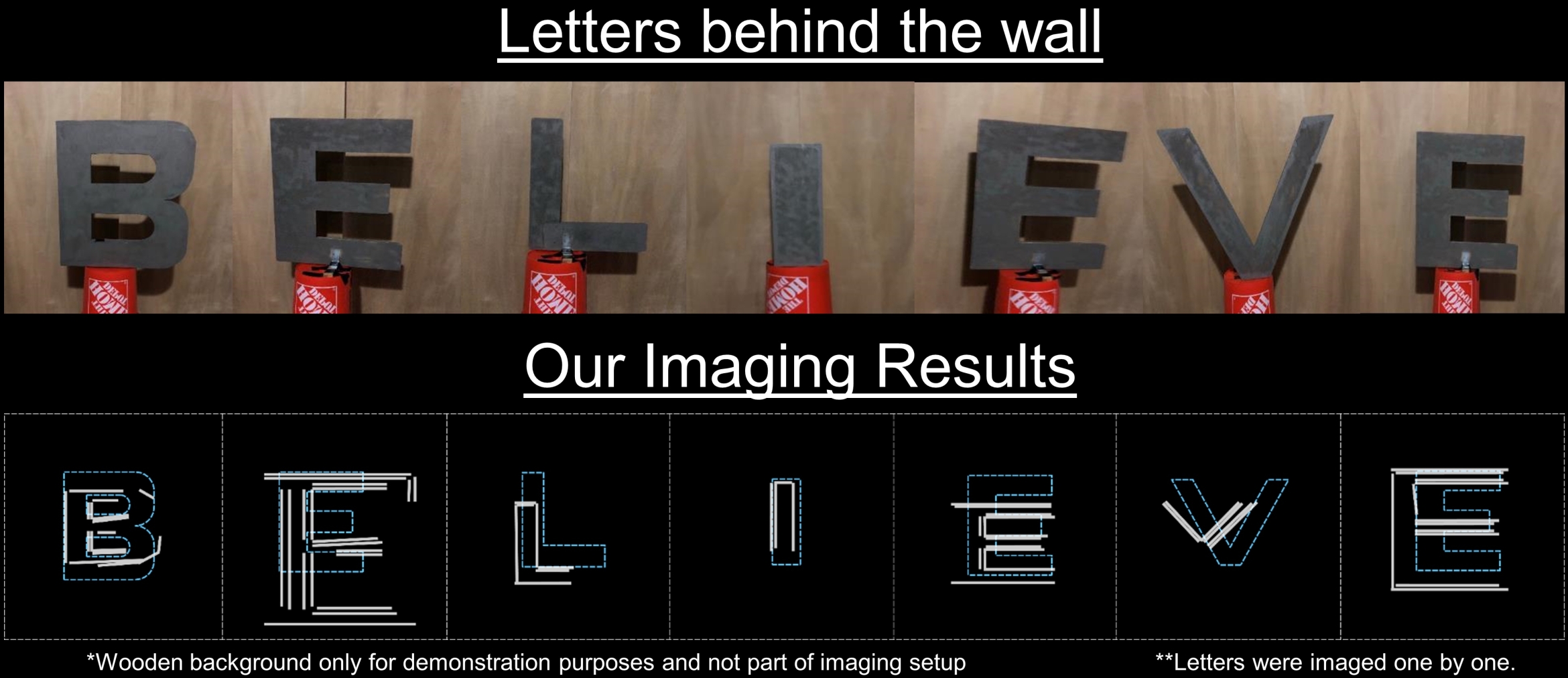
Photo Credit
Courtesy Mostofi Lab
To showcase the capabilities of the proposed pipeline in imaging complex details, the researchers have shown how WiFi can image the English alphabet, even through walls
This innovation builds on previous work in the Mostofi Lab, which since 2009 has pioneered sensing with everyday radio frequency signals such as WiFi for several different applications, including crowd analytics, person identification, smart health and smart spaces.
“When a given wave is incident on an edge point, a cone of outgoing rays emerges according to the Keller’s Geometrical Theory of Diffraction (GTD), referred to as a Keller cone,” Mostofi explained. The researchers note that this interaction is not limited to visibly sharp edges but applies to a broader set of surfaces with a small enough curvature.
“Depending on the edge orientation, the cone then leaves different footprints (i.e., conic sections) on a given receiver grid. We then develop a mathematical framework that uses these conic footprints as signatures to infer the orientation of the edges, thus creating an edge map of the scene,” Mostofi continued.
Image
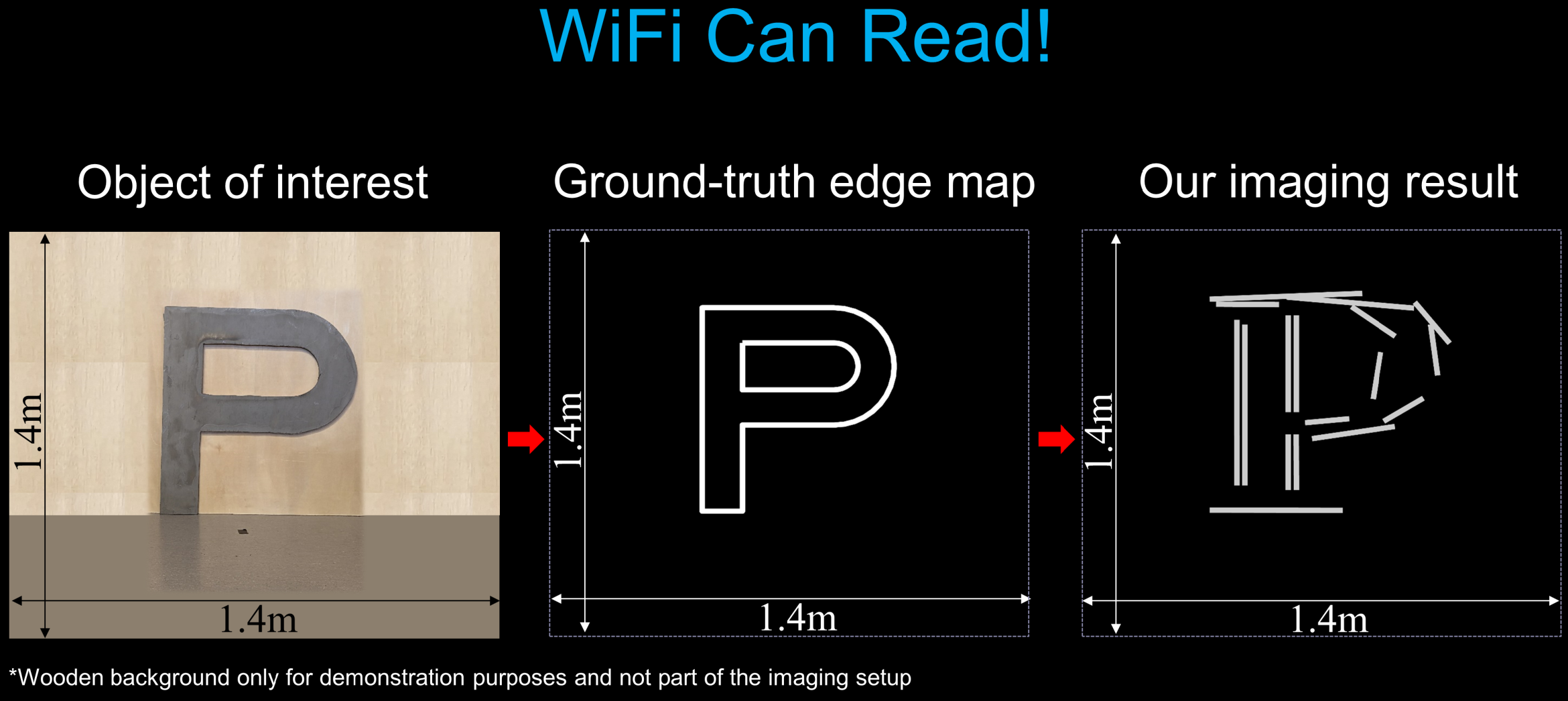
Photo Credit
Courtesy Mostofi Lab
Sample imaging in non-through-wall settings: Their method can image details of letter P in ways not possible before.
More specifically, the team proposed a Keller cone-based imaging projection kernel. This kernel is implicitly a function of the edge orientations, a relationship that is then exploited to infer the existence/orientation of the edges via hypothesis testing over a small set of possible edge orientations. In other words, if existence of an edge is determined, the edge orientation that best matches the resulting Keller cone-based signature is chosen for a given point that they are interested in imaging.
“Edges of real-life objects have local dependencies,” said Anurag Pallaprolu, the lead Ph.D. student on the project. “Thus, once we find the high-confidence edge points via the proposed imaging kernel, we then propagate their information to the rest of the points using Bayesian information propagation. This step can further help improve the image, since some of the edges may be in a blind region, or can be overpowered by other edges that are closer to the transmitters.” Finally, once an image is formed, the researchers can further improve the image by using image completion tools from the area of vision.
“It is worth noting that traditional imaging techniques result in poor imaging quality when deployed with commodity WiFi transceivers,” added Pallaprolu, “as the surfaces can appear near-specular at lower frequencies, thus not leaving enough signature on the receiver grid.”
The researchers have also extensively studied the impact of several different parameters, such as curvature of a surface, edge orientation, distance to the receiver grid, and transmitter location on the Keller cones and their proposed edge-based imaging system, thereby developing a foundation for a methodical imaging system design.
"We have then taken a completely different approach to tackle this challenging problem by focusing on tracing the edges of the objects instead."
In the team’s experiments, three off-the-shelf WiFi transmitters send wireless waves in the area. WiFi receivers are then mounted on an unmanned vehicle that emulates a WiFi receiver grid as it moves. The receiver measures the received signal power which it then uses for imaging, based on the proposed methodology.
The researchers have extensively tested this technology with several experiments in three different areas, including through-wall scenarios. In one example application, they developed a WiFi Reader to showcase the capabilities of the proposed pipeline.
This application is particularly informative as the English alphabet presents complex details which can be used to test the performance of the imaging system. Along this line, the group has shown how they can successfully image several alphabet-shaped objects. In addition to imaging, they can further classify the letters. Finally, they have shown how their approach enables WiFi to image and read through walls by imaging the details and further reading the letters of the word “BELIEVE” through walls. They have furthermore imaged a number of other objects as well, showing that they can capture details previously not possible with WiFi.
Overall, the proposed approach can open up new directions for RF imaging.
Image
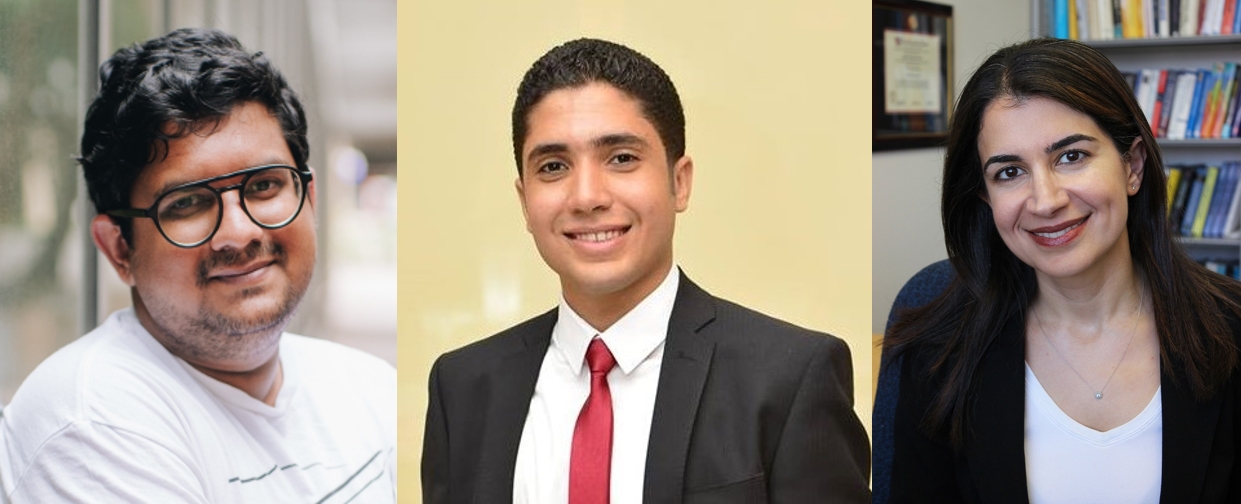
Photo Credit
Courtesy Mostofi Lab
From left to right: Ph.D. student Anurag Pallaprolu; former Ph.D. student Belal Korany and Professor Yasamin Mostofi
More information about the project can be found at Reading Through Walls With WiFi
Additional information about Mostofi’s research is available at http://www.ece.ucsb.edu/~ymostofi/.
Mostofi can be reached at ymostofi@ece.ucsb.edu.