PanoHead: Geometry-Aware 3D Full-Head Synthesis in 360°
PanoHead: Geometry-Aware 3D Full-Head Synthesis in 360°
sizhean.github.io
PanoHead: Geometry-Aware 3D Full-Head Synthesis in 360°
Sizhe An, Hongyi Xu, Yichun Shi, Guoxian Song, Umit Y. Ogras, Linjie LuoUniversity of Wisconsin-Madison, ByteDance Inc.
Paper arXiv Video Code
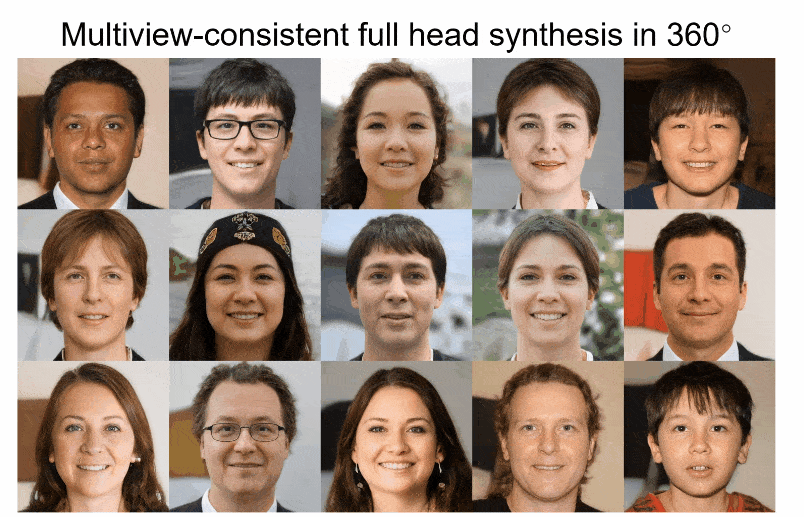
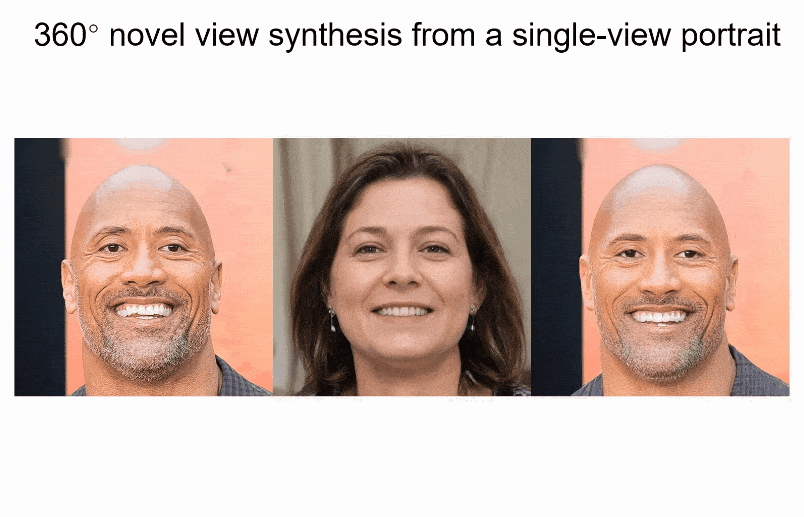
Abstract
Synthesis and reconstruction of 3D human head has gained increasing interests in computer vision and computer graphics recently. Existing state-of-the-art 3D generative adversarial networks (GANs) for 3D human head synthesis are either limited to near-frontal views or hard to preserve 3D consistency in large view angles. We propose PanoHead, the first 3D-aware generative model that enables high-quality view-consistent image synthesis of full heads in 360° with diverse appearance and detailed geometry using only in-the-wild unstructured images for training. At its core, we lift up the representation power of recent 3D GANs and bridge the data alignment gap when training from in-the-wild images with widely distributed views. Specifically, we propose a novel two-stage self-adaptive image alignment for robust 3D GAN training. We further introduce a tri-grid neural volume representation that effectively addresses front-face and back-head feature entanglement rooted in the widely-adopted tri-plane formulation. Our method instills prior knowledge of 2D image segmentation in adversarial learning of 3D neural scene structures, enabling compositable head synthesis in diverse backgrounds. Benefiting from these designs, our method significantly outperforms previous 3D GANs, generating high-quality 3D heads with accurate geometry and diverse appearances, even with long wavy and afro hairstyles, renderable from arbitrary poses. Furthermore, we show that our system can reconstruct full 3D heads from single input images for personalized realistic 3D avatars.
Last edited: