1/7
DeepSeek is the level of cracked when a quant finance firm from China is forced to work on AI cuz the government says they're not contributing to society
Imagine if all the perf guys at US quants worked on useful important stuff instead of making markets 0.1 second more efficient
2/7
Liang Wenfeng(deepseek/High flyer founder) was AGI pilled since 2008
Read this interview:
揭秘DeepSeek:一个更极致的中国技术理想主义故事
They also talk about you
3/7
Ye I read it. P cool. Llama 3 paper is better now though maybe
4/7
Renaissance makes too much. Probably higher ROI for them to keep doing quant math
5/7
do you believe that efficient markets are important for facilitating investment in the US? most of my CN friends just invest into real estate which seems like a massive inefficiency in their private sector.
6/7
Is the American financial industry wasting intelligence?
7/7
lol probably it’s because there’s not much money to make in China’s financial market. Derivatives are limited and the equity market never rises. The government always blames quant firms to cover their incompetence. Maybe they are just better off doing AI research …
To post tweets in this format, more info here: https://www.thecoli.com/threads/tips-and-tricks-for-posting-the-coli-megathread.984734/post-52211196
DeepSeek is the level of cracked when a quant finance firm from China is forced to work on AI cuz the government says they're not contributing to society
Imagine if all the perf guys at US quants worked on useful important stuff instead of making markets 0.1 second more efficient
2/7
Liang Wenfeng(deepseek/High flyer founder) was AGI pilled since 2008
Read this interview:
揭秘DeepSeek:一个更极致的中国技术理想主义故事
They also talk about you
3/7
Ye I read it. P cool. Llama 3 paper is better now though maybe
4/7
Renaissance makes too much. Probably higher ROI for them to keep doing quant math
5/7
do you believe that efficient markets are important for facilitating investment in the US? most of my CN friends just invest into real estate which seems like a massive inefficiency in their private sector.
6/7
Is the American financial industry wasting intelligence?
7/7
lol probably it’s because there’s not much money to make in China’s financial market. Derivatives are limited and the equity market never rises. The government always blames quant firms to cover their incompetence. Maybe they are just better off doing AI research …
To post tweets in this format, more info here: https://www.thecoli.com/threads/tips-and-tricks-for-posting-the-coli-megathread.984734/post-52211196
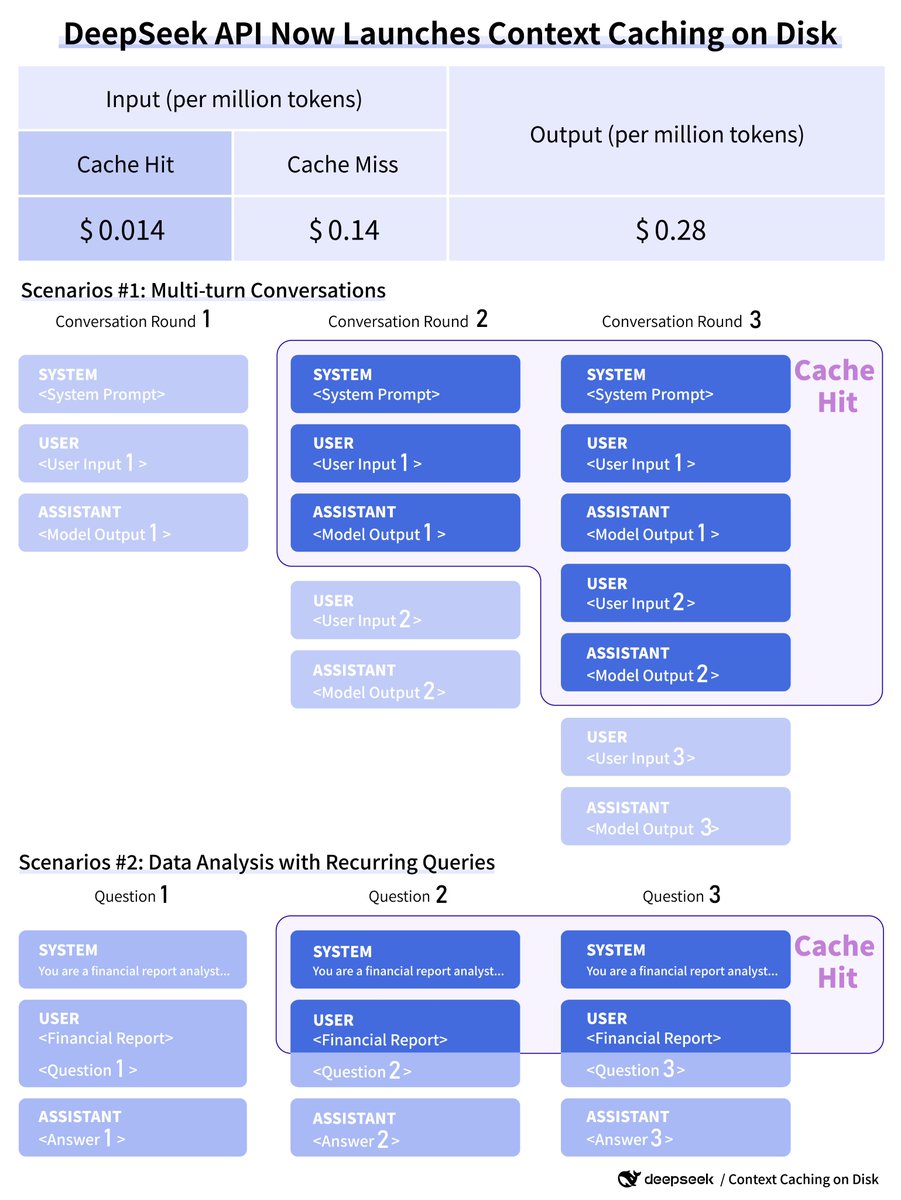
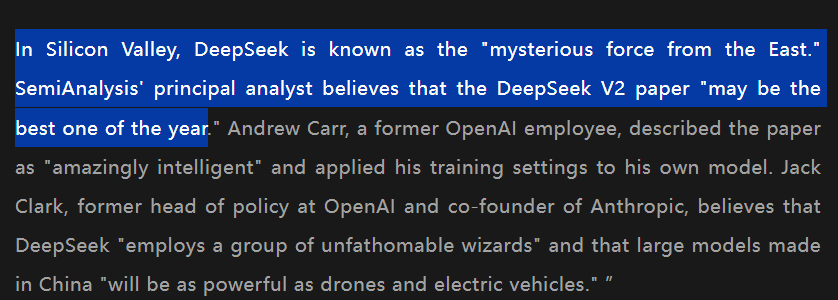
1/11
Exciting news! DeepSeek API now launches context caching on disk, with no code changes required! This new feature automatically caches frequently referenced contexts on distributed storage, slashing API costs by up to 90%. For a 128K prompt with high reference, the first token latency is cut from 13s to just 500ms.
Benefit Scenarios:
- Multi-turn conversations where subsequent rounds hit the previous context cache.
- Data analysis with recurring queries on the same documents/files.
- Code analysis/debugging with repeated repository references.
DeepSeek API disk caching is now live with unlimited concurrency. Billing is automatic based on actual cache hits. Learn more at DeepSeek API introduces Context Caching on Disk, cutting prices by an order of magnitude | DeepSeek API Docs
/search?q=#DeepSeek /search?q=#ContextCaching
2/11
It seems that a response issue has appeared after the update. 500s is crazy.
3/11
The issue has been fixed, and service is now restored.
4/11
interesting feature for cost savings
i've started using gpt4 mini to reduce costs but would love to stick with more expensive models on http://microlaunch.net
eventually w/ context caching
does deepseek also handle prompt compression?
5/11
This is awesome
6/11
I
you (I'm literally just a whale) 
7/11
The DeepSeek V2 and Coder V2 OpenRouter's API version are running the update 0628?
8/11
我没有中国手机号,我该怎么使用它呢?
9/11
wait so... this just automatically happens if you're using the deepseek models now? only through deepseek, or through openrouter/deekseek too? i'd love more clarification - amazing nonetheless!
10/11
国产之光!为你们感到骄傲!


11/11
This is amazing. Will it work for openrouter also?
To post tweets in this format, more info here: https://www.thecoli.com/threads/tips-and-tricks-for-posting-the-coli-megathread.984734/post-52211196

Benefit Scenarios:
- Multi-turn conversations where subsequent rounds hit the previous context cache.
- Data analysis with recurring queries on the same documents/files.
- Code analysis/debugging with repeated repository references.
DeepSeek API disk caching is now live with unlimited concurrency. Billing is automatic based on actual cache hits. Learn more at DeepSeek API introduces Context Caching on Disk, cutting prices by an order of magnitude | DeepSeek API Docs
/search?q=#DeepSeek /search?q=#ContextCaching
2/11
It seems that a response issue has appeared after the update. 500s is crazy.
3/11
The issue has been fixed, and service is now restored.
4/11
interesting feature for cost savings
i've started using gpt4 mini to reduce costs but would love to stick with more expensive models on http://microlaunch.net
eventually w/ context caching
does deepseek also handle prompt compression?
5/11
This is awesome

6/11
I


7/11
The DeepSeek V2 and Coder V2 OpenRouter's API version are running the update 0628?
8/11
我没有中国手机号,我该怎么使用它呢?
9/11
wait so... this just automatically happens if you're using the deepseek models now? only through deepseek, or through openrouter/deekseek too? i'd love more clarification - amazing nonetheless!
10/11
国产之光!为你们感到骄傲!



11/11
This is amazing. Will it work for openrouter also?
To post tweets in this format, more info here: https://www.thecoli.com/threads/tips-and-tricks-for-posting-the-coli-megathread.984734/post-52211196
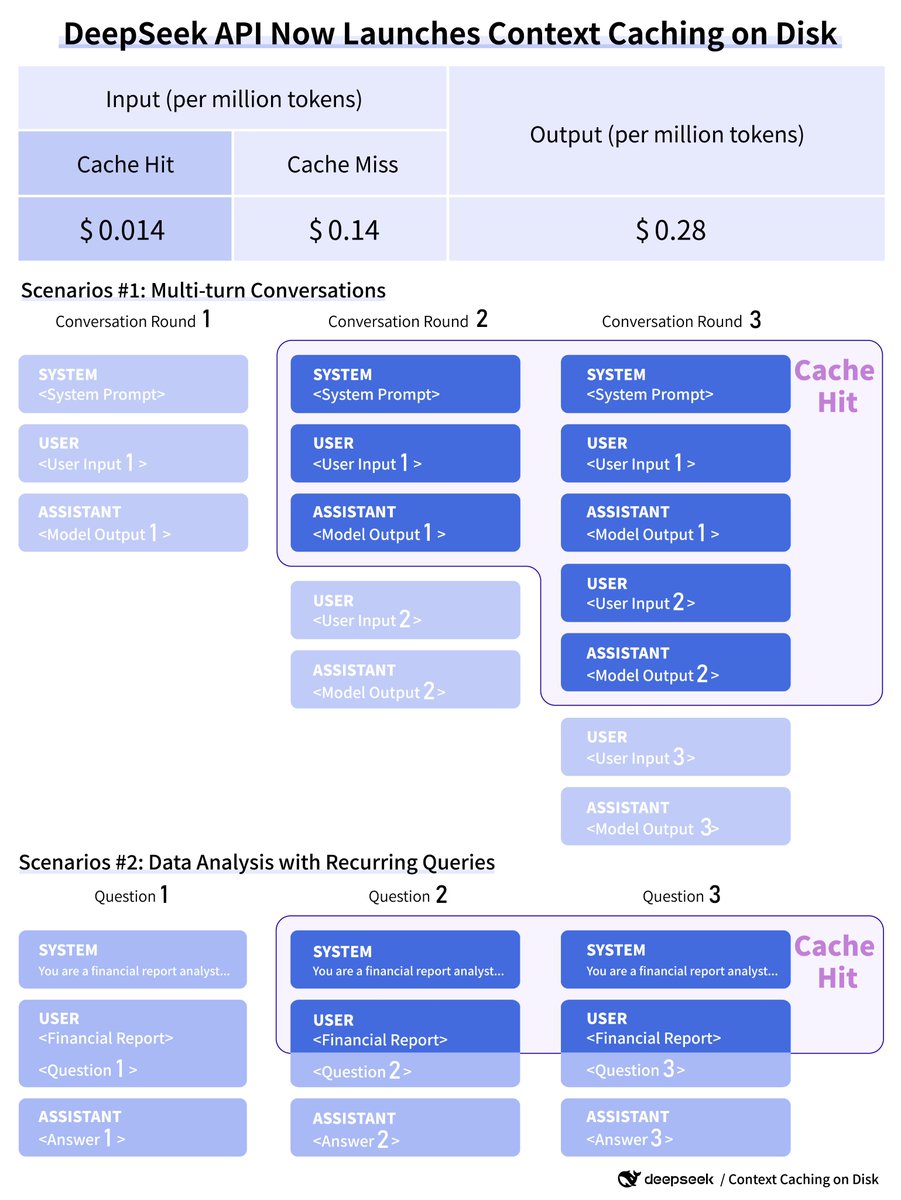
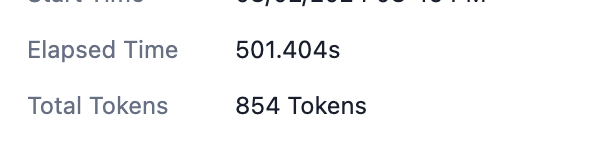