1/9
mPLUG-DocOwl 1.5
Unified Structure Learning for OCR-free Document Understanding
Structure information is critical for understanding the semantics of text-rich images, such as documents, tables, and charts. Existing Multimodal Large Language Models (MLLMs) for
2/9
Visual Document Understanding are equipped with text recognition ability but lack general structure understanding abilities for text-rich document images. In this work, we emphasize the importance of structure information in Visual Document Understanding and propose the
3/9
Unified Structure Learning to boost the performance of MLLMs. Our Unified Structure Learning comprises structure-aware parsing tasks and multi-grained text localization tasks across 5 domains: document, webpage, table, chart, and natural image. To better encode
4/9
structure information, we design a simple and effective vision-to-text module H-Reducer, which can not only maintain the layout information but also reduce the length of visual features by merging horizontal adjacent patches through convolution, enabling the LLM to
5/9
understand high-resolution images more efficiently. Furthermore, by constructing structure-aware text sequences and multi-grained pairs of texts and bounding boxes for publicly available text-rich images, we build a comprehensive training set DocStruct4M to support
6/9
structure learning. Finally, we construct a small but high-quality reasoning tuning dataset DocReason25K to trigger the detailed explanation ability in the document domain. Our model DocOwl 1.5 achieves state-of-the-art performance on 10 visual document
7/9
understanding benchmarks, improving the SOTA performance of MLLMs with a 7B LLM by more than 10 points in 5/10 benchmarks.
8/9
paper page:
9/9
Google presents Chart-based Reasoning
Transferring Capabilities from LLMs to VLMs
Vision-language models (VLMs) are achieving increasingly strong performance on multimodal tasks. However, reasoning capabilities remain limited particularly for smaller VLMs, while those of
mPLUG-DocOwl 1.5
Unified Structure Learning for OCR-free Document Understanding
Structure information is critical for understanding the semantics of text-rich images, such as documents, tables, and charts. Existing Multimodal Large Language Models (MLLMs) for
2/9
Visual Document Understanding are equipped with text recognition ability but lack general structure understanding abilities for text-rich document images. In this work, we emphasize the importance of structure information in Visual Document Understanding and propose the
3/9
Unified Structure Learning to boost the performance of MLLMs. Our Unified Structure Learning comprises structure-aware parsing tasks and multi-grained text localization tasks across 5 domains: document, webpage, table, chart, and natural image. To better encode
4/9
structure information, we design a simple and effective vision-to-text module H-Reducer, which can not only maintain the layout information but also reduce the length of visual features by merging horizontal adjacent patches through convolution, enabling the LLM to
5/9
understand high-resolution images more efficiently. Furthermore, by constructing structure-aware text sequences and multi-grained pairs of texts and bounding boxes for publicly available text-rich images, we build a comprehensive training set DocStruct4M to support
6/9
structure learning. Finally, we construct a small but high-quality reasoning tuning dataset DocReason25K to trigger the detailed explanation ability in the document domain. Our model DocOwl 1.5 achieves state-of-the-art performance on 10 visual document
7/9
understanding benchmarks, improving the SOTA performance of MLLMs with a 7B LLM by more than 10 points in 5/10 benchmarks.
8/9
paper page:
9/9
Google presents Chart-based Reasoning
Transferring Capabilities from LLMs to VLMs
Vision-language models (VLMs) are achieving increasingly strong performance on multimodal tasks. However, reasoning capabilities remain limited particularly for smaller VLMs, while those of
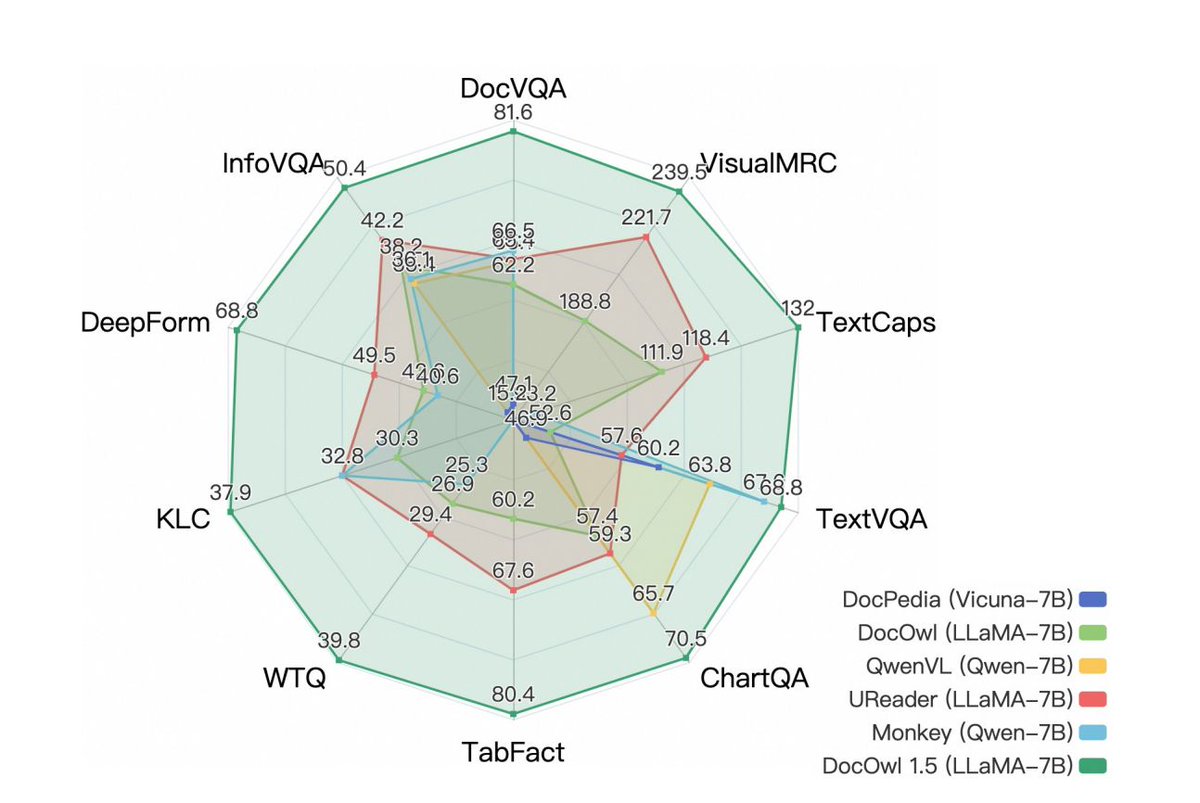
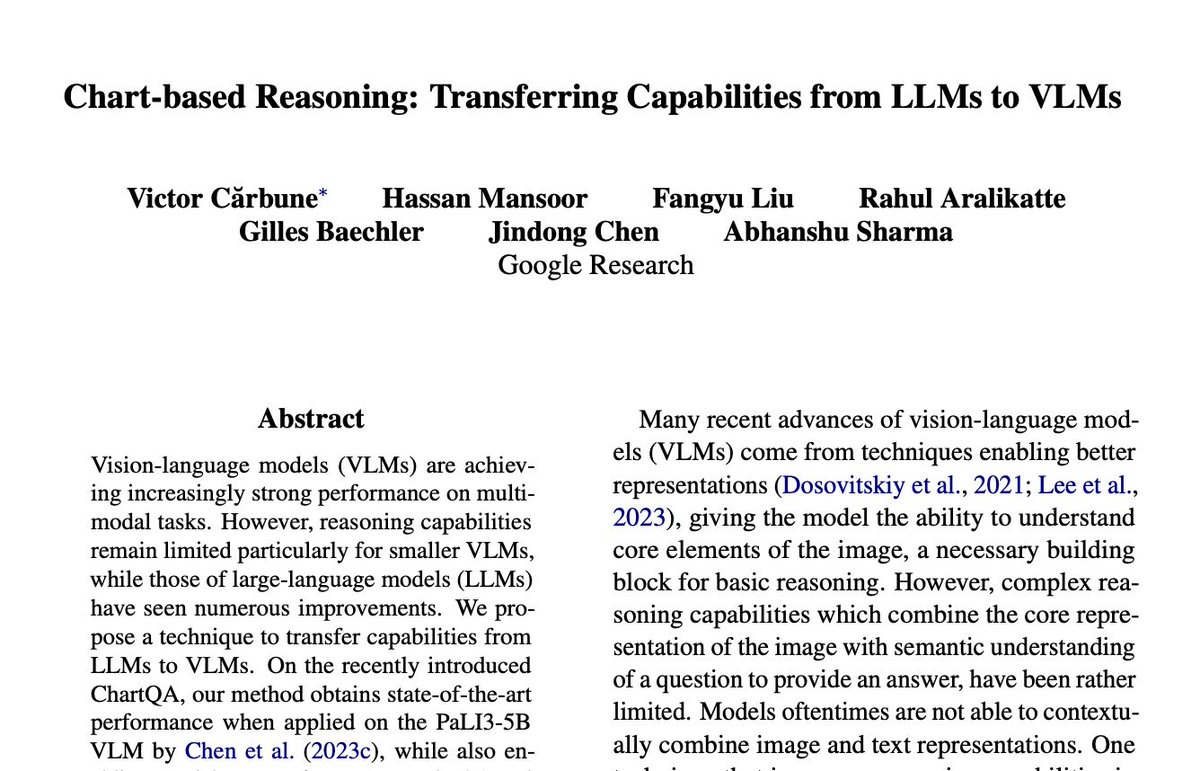