
Meta AI Introduces Priority Sampling: Elevating Machine Learning with Deterministic Code Generation
Large language models (LLMs) have emerged as powerful tools capable of performing tasks with remarkable efficiency and accuracy. These models have demonstrated their prowess in generating code, translating programming languages, writing unit tests, and detecting and fixing bugs. Innovations like...
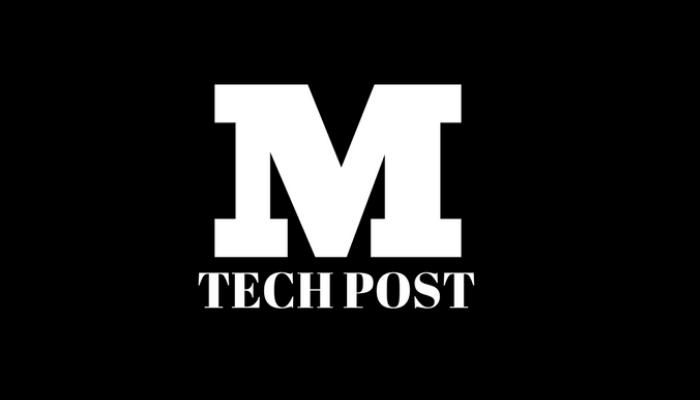
Meta AI Introduces Priority Sampling: Elevating Machine Learning with Deterministic Code Generation
By Sana Hassan
March 5, 2024
Large language models (LLMs) have emerged as powerful tools capable of performing tasks with remarkable efficiency and accuracy. These models have demonstrated their prowess in generating code, translating programming languages, writing unit tests, and detecting and fixing bugs. Innovations like CodeLlama, ChatGPT, and Codex have significantly improved the coding experience by excelling in various code manipulation tasks. Some models, such as AlphaCode, are even pretrained on competitive programming tasks, enabling them to optimize code at the source level across several languages.
The challenge at the heart of utilizing LLMs for tasks such as code generation lies in their ability to produce diverse and high-quality outputs. Traditional sampling methods, while useful, often need to catch up in generating a wide range of viable solutions. This limitation becomes particularly evident in code generation, where the ability to explore different implementation ideas can significantly enhance the development process. The problem intensifies with methods like temperature-based sampling, which, despite increasing output diversity, require extensive computation to find the optimal setting.
Current approaches to enhancing the diversity and quality of outputs from LLMs include stochastic methods and beam search techniques. Stochastic methods introduce randomness in the selection process to increase output variety, with strategies like Top-k Sampling and Nucleus Sampling focusing on the most probable tokens to maintain diversity. Meanwhile, beam search methods, such as Diverse Beam Search and Determinantal Beam Search, manipulate expansion mechanisms to explore different paths and ensure a broader range of generated outputs. These methods aim to tackle the limitations of traditional sampling by providing mechanisms that can produce more diverse and high-quality results, albeit with varying degrees of success and inherent challenges.
The research introduces Priority Sampling, a novel method developed by a team from Rice University and Meta AI. This technique is designed to enhance the performance of LLMs in generating diverse and high-quality outputs, particularly in code generation and optimization. Priority Sampling offers a deterministic approach that guarantees the production of unique samples, systematically expands the search tree based on model confidence, and incorporates regular expression support for controlled and structured exploration.
Priority Sampling operates by expanding the unexpanded token with the highest probability in an augmented search tree, ensuring that each new sample is unique and ordered by the model’s confidence. This approach addresses the common issue of duplicate or irrelevant outputs found in traditional sampling methods, providing a more efficient and effective means of generating diverse solutions. Regular expression support allows for more controlled exploration, enabling the generation of outputs that adhere to specific patterns or constraints.
The performance of Priority Sampling has been rigorously evaluated, particularly in the context of LLVM pass-ordering tasks. The method demonstrated a remarkable ability to boost the performance of the original model, achieving significant improvements over default optimization techniques. This success underscores the potential of Priority Sampling to access and leverage the vast knowledge stored within LLMs through strategic expansion of the search tree. The results highlight the method’s effectiveness in generating diverse and high-quality outputs and its potential to outperform existing autotuners for training label generation.
In conclusion, priority Sampling represents a significant leap forward in utilizing large language models for code generation and optimization tasks. By addressing the limitations of traditional sampling methods, this research offers a more efficient and effective approach to generating diverse and high-quality outputs. The method’s deterministic nature, coupled with its support for regular expression-based generation, provides a controlled and structured exploration process that can significantly enhance the capabilities of LLMs.
Computer Science > Machine Learning
[Submitted on 28 Feb 2024]Priority Sampling of Large Language Models for Compilers
Dejan Grubisic, Chris Cummins, Volker Seeker, Hugh LeatherLarge language models show great potential in generating and optimizing code. Widely used sampling methods such as Nucleus Sampling increase the diversity of generation but often produce repeated samples for low temperatures and incoherent samples for high temperatures. Furthermore, the temperature coefficient has to be tuned for each task, limiting its usability. We present Priority Sampling, a simple and deterministic sampling technique that produces unique samples ordered by the model's confidence. Each new sample expands the unexpanded token with the highest probability in the augmented search tree. Additionally, Priority Sampling supports generation based on regular expression that provides a controllable and structured exploration process. Priority Sampling outperforms Nucleus Sampling for any number of samples, boosting the performance of the original model from 2.87% to 5% improvement over -Oz. Moreover, it outperforms the autotuner used for the generation of labels for the training of the original model in just 30 samples.
Subjects: | Machine Learning (cs.LG); Computation and Language (cs.CL); Performance (cs.PF) |
Cite as: | arXiv:2402.18734 [cs.LG] |
(or arXiv:2402.18734v1 [cs.LG] for this version) |
Submission history
From: Dejan Grubisic [view email][v1] Wed, 28 Feb 2024 22:27:49 UTC (1,116 KB)
aI generated explanation:
Imagine you have a magic helper called a "large language model" (LLM) that's really good at understanding and creating code. It can do things like translate code, write tests, and fix mistakes. Some special helpers like CodeLlama, ChatGPT, and Codex make coding even easier.
But there's a problem: when these helpers create code, they don't always come up with lots of different, good ideas. They might get stuck on just one way of doing things. To fix this, people have tried different ways to help the helpers think more creatively. One way is called "temperature-based sampling," but it takes a lot of time to work well.
Other ways, like "stochastic methods" (random choices) and "beam search techniques," try to give more varied and better code. But they still have some problems and don't always work perfectly.
Now, some smart people from Rice University and Meta AI created a new method called "Priority Sampling." It's like a treasure hunt where the helper always chooses the next most likely and unique idea to explore. It also has a rule-following feature called "regular expressions" that helps the helper create code that fits certain patterns.
Priority Sampling was tested on a task related to organizing code, and it showed great improvement over other methods. It's more efficient and creates more diverse, high-quality code. This means that Priority Sampling could be a big step forward in making these language models even better at generating and improving code.