1/3
StructLM
Towards Building Generalist Models for Structured Knowledge Grounding
Structured data sources, such as tables, graphs, and databases, are ubiquitous knowledge sources. Despite the demonstrated capabilities of large language models (LLMs) on plain text, their proficiency in interpreting and utilizing structured data remains limited. Our investigation reveals a notable deficiency in LLMs' ability to process structured data, e.g., ChatGPT lags behind state-of-the-art (SoTA) model by an average of 35%. To augment the Structured Knowledge Grounding (SKG) capabilities in LLMs, we have developed a comprehensive instruction tuning dataset comprising 1.1 million examples. Utilizing this dataset, we train a series of models, referred to as StructLM, based on the Code-LLaMA architecture, ranging from 7B to 34B parameters. Our StructLM series surpasses task-specific models on 14 out of 18 evaluated datasets and establishes new SoTA achievements on 7 SKG tasks. Furthermore, StructLM demonstrates exceptional generalization across 6 novel SKG tasks. Contrary to expectations, we observe that scaling model size offers marginal benefits, with StructLM-34B showing only slight improvements over StructLM-7B. This suggests that structured knowledge grounding is still a challenging task and requires more innovative design to push to a new level.
2/3
paper page:
3/3
Beyond Language Models
Byte Models are Digital World Simulators
Traditional deep learning often overlooks bytes, the basic units of the digital world, where all forms of information and operations are encoded and manipulated in binary format. Inspired by the success of next
StructLM
Towards Building Generalist Models for Structured Knowledge Grounding
Structured data sources, such as tables, graphs, and databases, are ubiquitous knowledge sources. Despite the demonstrated capabilities of large language models (LLMs) on plain text, their proficiency in interpreting and utilizing structured data remains limited. Our investigation reveals a notable deficiency in LLMs' ability to process structured data, e.g., ChatGPT lags behind state-of-the-art (SoTA) model by an average of 35%. To augment the Structured Knowledge Grounding (SKG) capabilities in LLMs, we have developed a comprehensive instruction tuning dataset comprising 1.1 million examples. Utilizing this dataset, we train a series of models, referred to as StructLM, based on the Code-LLaMA architecture, ranging from 7B to 34B parameters. Our StructLM series surpasses task-specific models on 14 out of 18 evaluated datasets and establishes new SoTA achievements on 7 SKG tasks. Furthermore, StructLM demonstrates exceptional generalization across 6 novel SKG tasks. Contrary to expectations, we observe that scaling model size offers marginal benefits, with StructLM-34B showing only slight improvements over StructLM-7B. This suggests that structured knowledge grounding is still a challenging task and requires more innovative design to push to a new level.
2/3
paper page:
3/3
Beyond Language Models
Byte Models are Digital World Simulators
Traditional deep learning often overlooks bytes, the basic units of the digital world, where all forms of information and operations are encoded and manipulated in binary format. Inspired by the success of next
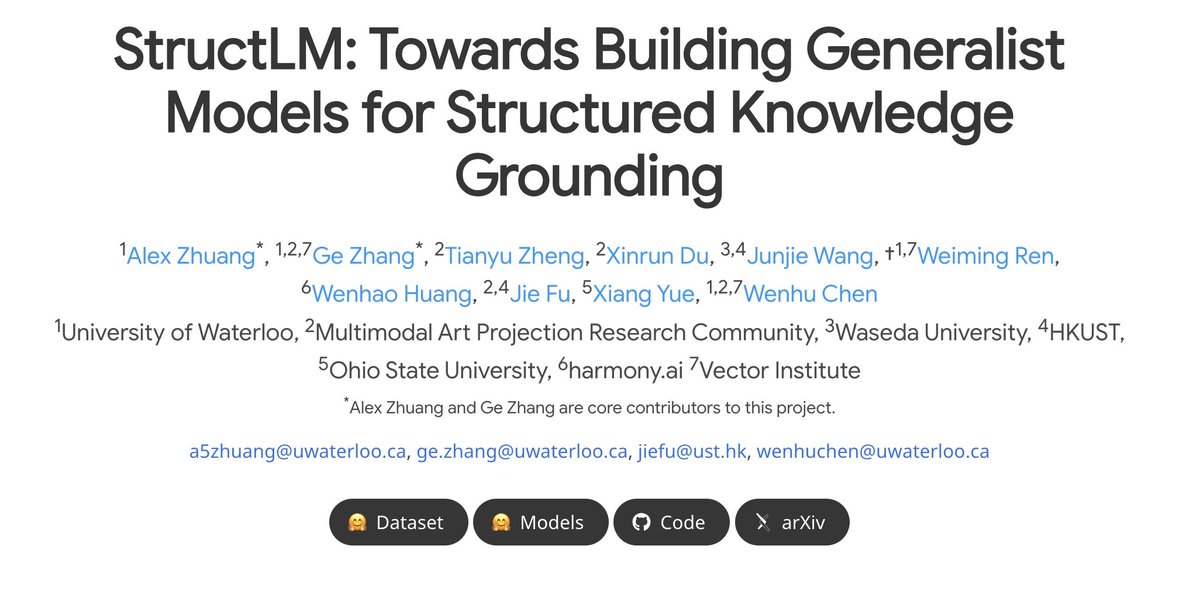
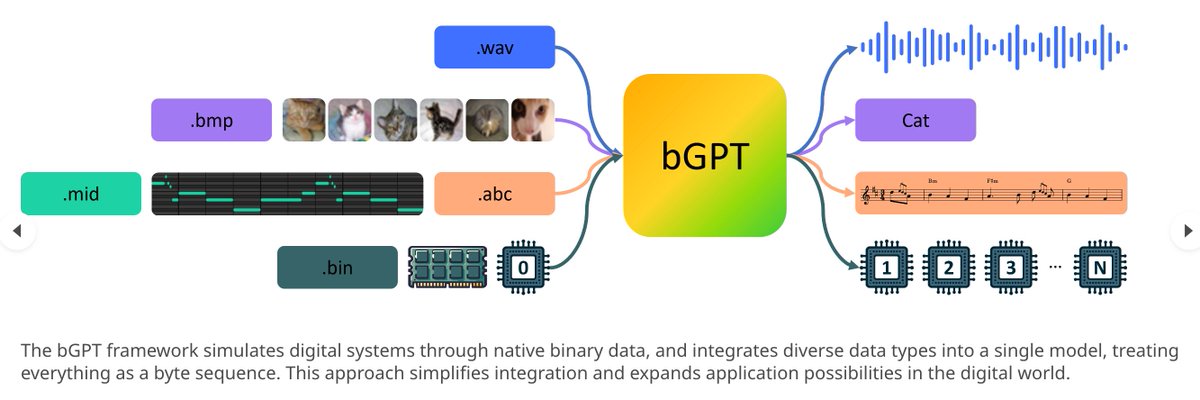
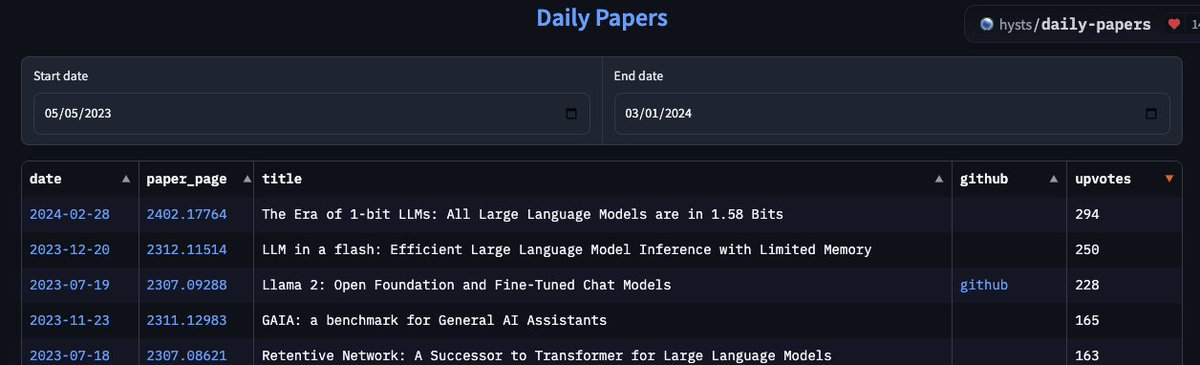