1/11
@GaryMarcus
Not the best cognitive psychology I have seen, because it confounds together abstract reasoning with domains where motivated reasoning is well-known to exist. Doesn’t show that humans can’t reason, but rather that they prefer not to.
Strange also that the very long literature on “motivated reasoning” is being reinvented here. This sounds like a nice study but social psychologists have known about these effects since at least the 1980s. (My 2008 book Kluge talks about it some. @jayvanbavel is a good expert on the recent related literature.)
[Quoted tweet]
Can Humans Reason?
Not really - most humans simply indulge in groupthink.
They found no evidence of independent reasoning in humans. Smarter humans become more sophisticated at self-deception and hallucinating arguments.
So, in some sense, AI can already do better than humans
2/11
@BethCarey12
The humble spreadsheet tool became ubiquitous *because* it doesn't make errors like humans.
When your goal is solving language understanding for machines, the path leads to the best of both worlds - emulating what the brain does with language while exploiting the benefits of computers and what they do best.
Language as learned when a human baby, has reasoning baked in. Capturing that, creates the magic without the 'hallucinations' and energy consumption.
Amazon.com
3/11
@bindureddy
Ok, I am clearly trying to be funny and failing by the look of this
4/11
@nathalieX70
As Elon has created the best hive mind with the Adrian community, it would tend to say Bindu is right. He failed to do so with Elon.
5/11
@HumblyAlex
Any of Gary's fanboys want to earn $50?
Booch couldn't earn himself the money, and Gary seems to avoid the high hanging fruits that exist much more than the imaginary one's he regularly swings at.
Easy money if Gary's right and his arguments hold up.
[Quoted tweet]
If you can find anything written by @Ylecun, @GaryMarcus, or @Grady_Booch that entirely and conclusively negates the following in a way that stands up to scrutiny, I will give you $50.
Something tells me it doesn't exist, and they avoid these truths at all costs.
6/11
@bate5a55
Interesting critique. Did you know that the "bias blind spot," identified in 2002, shows even trained logicians fail to recognize their own reasoning biases? It highlights how self-awareness doesn't always mitigate motivated reasoning.
7/11
@BankingNeko
I agree with GaryMarcus, the study's findings aren't new and social psychologists have known this for decades. The critique of the methodology is spot on, we need more nuanced research on human reasoning.
8/11
@Gazorble
John Locke "Few men think, yet all will have opinions. Hence men’s opinions are superficial and confused."

9/11
@TrustInAutonomy
Ffs
10/11
@gauaren
Such a funny way for the retweeted person to show they don't understand the difference between can and do. Maybe an LLM can (haha) explain it to them
11/11
@FaustinoBerlin
To post tweets in this format, more info here: https://www.thecoli.com/threads/tips-and-tricks-for-posting-the-coli-megathread.984734/post-52211196
@GaryMarcus
Not the best cognitive psychology I have seen, because it confounds together abstract reasoning with domains where motivated reasoning is well-known to exist. Doesn’t show that humans can’t reason, but rather that they prefer not to.
Strange also that the very long literature on “motivated reasoning” is being reinvented here. This sounds like a nice study but social psychologists have known about these effects since at least the 1980s. (My 2008 book Kluge talks about it some. @jayvanbavel is a good expert on the recent related literature.)
[Quoted tweet]
Can Humans Reason?
Not really - most humans simply indulge in groupthink.
They found no evidence of independent reasoning in humans. Smarter humans become more sophisticated at self-deception and hallucinating arguments.
So, in some sense, AI can already do better than humans

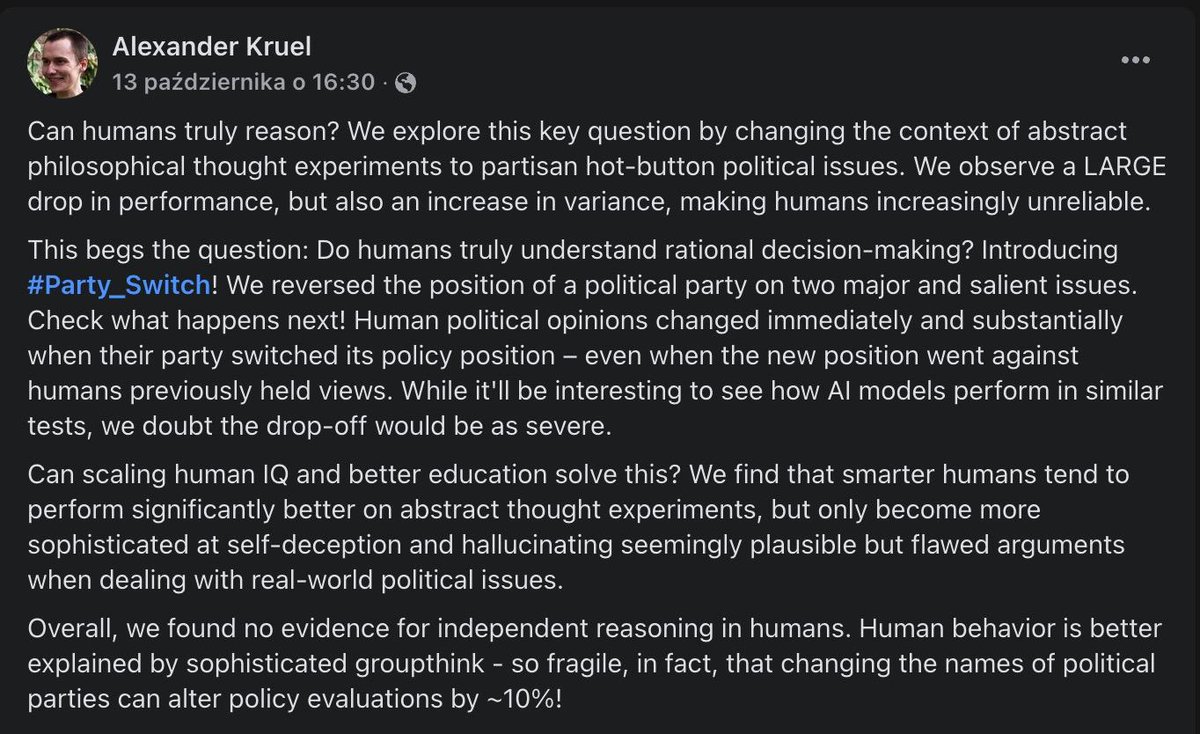
2/11
@BethCarey12
The humble spreadsheet tool became ubiquitous *because* it doesn't make errors like humans.
When your goal is solving language understanding for machines, the path leads to the best of both worlds - emulating what the brain does with language while exploiting the benefits of computers and what they do best.
Language as learned when a human baby, has reasoning baked in. Capturing that, creates the magic without the 'hallucinations' and energy consumption.
Amazon.com
3/11
@bindureddy
Ok, I am clearly trying to be funny and failing by the look of this
4/11
@nathalieX70
As Elon has created the best hive mind with the Adrian community, it would tend to say Bindu is right. He failed to do so with Elon.
5/11
@HumblyAlex
Any of Gary's fanboys want to earn $50?
Booch couldn't earn himself the money, and Gary seems to avoid the high hanging fruits that exist much more than the imaginary one's he regularly swings at.
Easy money if Gary's right and his arguments hold up.
[Quoted tweet]
If you can find anything written by @Ylecun, @GaryMarcus, or @Grady_Booch that entirely and conclusively negates the following in a way that stands up to scrutiny, I will give you $50.
Something tells me it doesn't exist, and they avoid these truths at all costs.
6/11
@bate5a55
Interesting critique. Did you know that the "bias blind spot," identified in 2002, shows even trained logicians fail to recognize their own reasoning biases? It highlights how self-awareness doesn't always mitigate motivated reasoning.
7/11
@BankingNeko
I agree with GaryMarcus, the study's findings aren't new and social psychologists have known this for decades. The critique of the methodology is spot on, we need more nuanced research on human reasoning.
8/11
@Gazorble
John Locke "Few men think, yet all will have opinions. Hence men’s opinions are superficial and confused."

9/11
@TrustInAutonomy
Ffs
10/11
@gauaren
Such a funny way for the retweeted person to show they don't understand the difference between can and do. Maybe an LLM can (haha) explain it to them
11/11
@FaustinoBerlin
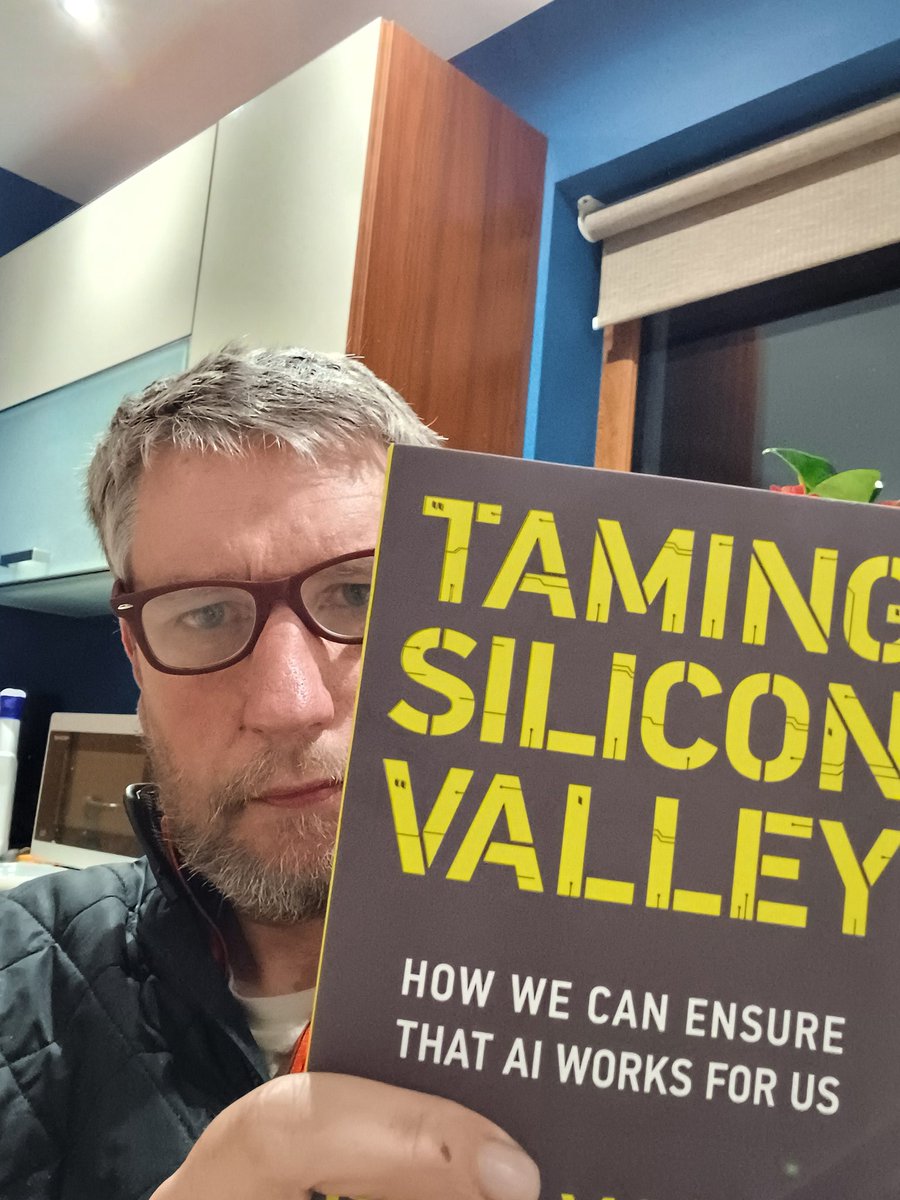
To post tweets in this format, more info here: https://www.thecoli.com/threads/tips-and-tricks-for-posting-the-coli-megathread.984734/post-52211196
1/11
@bindureddy
Can Humans Reason?
Not really - most humans simply indulge in groupthink.
They found no evidence of independent reasoning in humans. Smarter humans become more sophisticated at self-deception and hallucinating arguments.
So, in some sense, AI can already do better than humans
2/11
@Hello_World
Humans can reason LLMs cant. Humans don't always reason but LLMs never do.
3/11
@01Singularity01
What I've been saying. Every time I see "AI can't" or "AI doesn't" I immediately think "the same can be said for most humans". Most humans do not generalize well, for example, and there is a wide range of generalization capabilities. Overwhelmingly, people want their bias confirmed. New information is disruptive to the established training, and to be avoided at all costs.
4/11
@gpt_biz
Interesting take, but I think humans' ability to feel empathy and adapt makes our reasoning unique in ways AI still can't fully grasp yet
5/11
@M1ndPrison
No surprise here. Emotion is the kryptonite of reasoning. That isn't to say humans can not reason.
This is akin to giving AI a terrible prompt and saying it can't solve the problem.
These are expected outcomes, but do not demonstrate a useful comparison.
6/11
@climatebabes
I am an exception..
7/11
@AI_Ethicist_NYC
'Experts' complain that AI "can't really" do this or that and that it just uses what it learns in its training data. It just predicts the most likely next token given the context. It hallucinates and comes up with wrong answers. Blah blah blah.
Ironically, it's all these flaws that make it more human-like, not less. Learning from data sets is exactly what people do (on their best day). People are not good at reasoning or remembering or putting two and two together. Some people are. Most people aren't.
Either way, who cares if AI is REALLY reasoning the way we perceive reasoning as long as it comes to a correctly reasoned result.
Do you really think artificial superintelligence is going to give a sh*t whether or not we've deemed its reasoning as 'genuine'? Do lions care what the sheep think?
I think we need to focus less on the idea that AI has to 100% process the world like the human brain does and be more concerned about developing AI so that it can help our brains think in a way they have not before.
8/11
@mr_trademaker
Can Humans Run Marathons?
Not really – most humans simply indulge in group inactivity, sticking to light exercise or none at all.
They found no evidence of marathon-level endurance in most humans. Fitter humans become more skilled at self-deception, convincing themselves they're training sufficiently when they aren’t.
So in some sense, AI can already do better then humans ;)
9/11
@PhDcornerHub
Epigenetic Building of the Evolutionary Mind
Autopoiesis and social intelligence reproduce the emergent properties of thought generation and application. This process involves adaptive thinking and abductive reasoning, both of which are more complex and nuanced. Autopoiesis embodies the essence of self-reproduction, a hallmark of living entities.
Unlike LLMs, the human brain builds a large foundational model as a filter that processes only relevant information from vast data. Nature has its own metrics for intelligence and survival. The general principle of emergence and the adaptation of the evolutionary survival program reflect cognitive learning mechanisms that are autopoietic self-catalysts. Over evolutionary lineage, these processes are automated in genetic systems as a form of learning.
However, these systems do not embody true intelligence. Instead, they mimic individual components, which in turn create social intelligence. Intelligence, distinct from knowledge, is not merely an accumulation of information; it involves the capacity to think and reason. Knowledge is the information and understanding gathered over time, while intelligence refers to the ability to engage in reasoning and reflection.
Machines, by processing vast amounts of data and text, can mimic intelligence. Yet, while machines may replicate wisdom based on textual input, they inherently lack the capacity for human-like thought and reasoning. Learning and developing intelligence are interconnected but distinct processes—one can learn without necessarily developing true intelligence. Language models may simulate aspects of cognitive processes through pattern recognition, but true intelligence encompasses the innate capacity to self-reflect and adapt beyond mere programmed responses.
No human is replaceable by a knowledge machine. Humans are thinking beings, while AI serves as a knowledge machine—it is not transformative, nor is its intelligence transferable. In the end, these technologies remain clouds of knowledge, with humans doing the real thinking.
While intelligence can be introduced into systems, the ability to think is self-learned, self-adaptive, and scaled through the human brain’s evolutionary and survival-driven intelligence. It is embedded in a cognitive social matrix connected to vast libraries of data that we have crafted into language. Most intelligence is shaped by adapting to these metrics. At the core, the brain is programmed to survive, reproduce, and exist, and all intelligence is an emergent property of thought generation and application.
Intelligence is an innate quality, not a genetic predisposition. Everyone who survives demonstrates intelligence at a basic level because our very nature is oriented towards survival, evolution, and reproduction. We must not confuse intelligence shaped by the ecosystem with inherent, born intelligence. The ability to think and intelligence is generic, yet it can also be cultivated. Knowledge and wisdom, meanwhile, form two distinct aspects of this intellectual journey.
AI Technology as the Lighthouse of Human Evolution
AI technology acts as a lighthouse and the torchbearer of human intelligence and knowledge, facilitating the genetic evolution of humankind towards higher levels of achievement and understanding.
10/11
@web3nam3
Humans can reason but don’t want to.
11/11
@adolfoasorlin
Was that a reasoning?
To post tweets in this format, more info here: https://www.thecoli.com/threads/tips-and-tricks-for-posting-the-coli-megathread.984734/post-52211196
@bindureddy
Can Humans Reason?
Not really - most humans simply indulge in groupthink.
They found no evidence of independent reasoning in humans. Smarter humans become more sophisticated at self-deception and hallucinating arguments.
So, in some sense, AI can already do better than humans

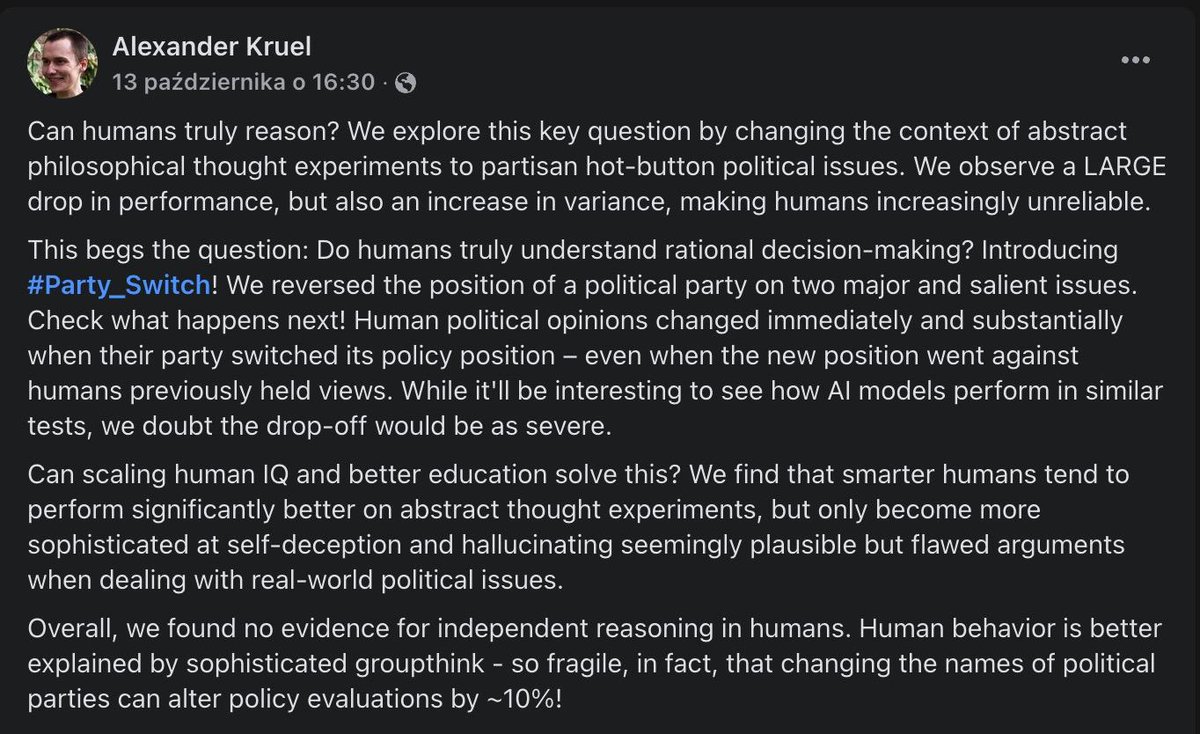
2/11
@Hello_World
Humans can reason LLMs cant. Humans don't always reason but LLMs never do.
3/11
@01Singularity01
What I've been saying. Every time I see "AI can't" or "AI doesn't" I immediately think "the same can be said for most humans". Most humans do not generalize well, for example, and there is a wide range of generalization capabilities. Overwhelmingly, people want their bias confirmed. New information is disruptive to the established training, and to be avoided at all costs.
4/11
@gpt_biz
Interesting take, but I think humans' ability to feel empathy and adapt makes our reasoning unique in ways AI still can't fully grasp yet
5/11
@M1ndPrison
No surprise here. Emotion is the kryptonite of reasoning. That isn't to say humans can not reason.
This is akin to giving AI a terrible prompt and saying it can't solve the problem.
These are expected outcomes, but do not demonstrate a useful comparison.
6/11
@climatebabes
I am an exception..
7/11
@AI_Ethicist_NYC

Ironically, it's all these flaws that make it more human-like, not less. Learning from data sets is exactly what people do (on their best day). People are not good at reasoning or remembering or putting two and two together. Some people are. Most people aren't.
Either way, who cares if AI is REALLY reasoning the way we perceive reasoning as long as it comes to a correctly reasoned result.
Do you really think artificial superintelligence is going to give a sh*t whether or not we've deemed its reasoning as 'genuine'? Do lions care what the sheep think?
I think we need to focus less on the idea that AI has to 100% process the world like the human brain does and be more concerned about developing AI so that it can help our brains think in a way they have not before.
8/11
@mr_trademaker
Can Humans Run Marathons?
Not really – most humans simply indulge in group inactivity, sticking to light exercise or none at all.
They found no evidence of marathon-level endurance in most humans. Fitter humans become more skilled at self-deception, convincing themselves they're training sufficiently when they aren’t.
So in some sense, AI can already do better then humans ;)
9/11
@PhDcornerHub
Epigenetic Building of the Evolutionary Mind
Autopoiesis and social intelligence reproduce the emergent properties of thought generation and application. This process involves adaptive thinking and abductive reasoning, both of which are more complex and nuanced. Autopoiesis embodies the essence of self-reproduction, a hallmark of living entities.
Unlike LLMs, the human brain builds a large foundational model as a filter that processes only relevant information from vast data. Nature has its own metrics for intelligence and survival. The general principle of emergence and the adaptation of the evolutionary survival program reflect cognitive learning mechanisms that are autopoietic self-catalysts. Over evolutionary lineage, these processes are automated in genetic systems as a form of learning.
However, these systems do not embody true intelligence. Instead, they mimic individual components, which in turn create social intelligence. Intelligence, distinct from knowledge, is not merely an accumulation of information; it involves the capacity to think and reason. Knowledge is the information and understanding gathered over time, while intelligence refers to the ability to engage in reasoning and reflection.
Machines, by processing vast amounts of data and text, can mimic intelligence. Yet, while machines may replicate wisdom based on textual input, they inherently lack the capacity for human-like thought and reasoning. Learning and developing intelligence are interconnected but distinct processes—one can learn without necessarily developing true intelligence. Language models may simulate aspects of cognitive processes through pattern recognition, but true intelligence encompasses the innate capacity to self-reflect and adapt beyond mere programmed responses.
No human is replaceable by a knowledge machine. Humans are thinking beings, while AI serves as a knowledge machine—it is not transformative, nor is its intelligence transferable. In the end, these technologies remain clouds of knowledge, with humans doing the real thinking.
While intelligence can be introduced into systems, the ability to think is self-learned, self-adaptive, and scaled through the human brain’s evolutionary and survival-driven intelligence. It is embedded in a cognitive social matrix connected to vast libraries of data that we have crafted into language. Most intelligence is shaped by adapting to these metrics. At the core, the brain is programmed to survive, reproduce, and exist, and all intelligence is an emergent property of thought generation and application.
Intelligence is an innate quality, not a genetic predisposition. Everyone who survives demonstrates intelligence at a basic level because our very nature is oriented towards survival, evolution, and reproduction. We must not confuse intelligence shaped by the ecosystem with inherent, born intelligence. The ability to think and intelligence is generic, yet it can also be cultivated. Knowledge and wisdom, meanwhile, form two distinct aspects of this intellectual journey.
AI Technology as the Lighthouse of Human Evolution
AI technology acts as a lighthouse and the torchbearer of human intelligence and knowledge, facilitating the genetic evolution of humankind towards higher levels of achievement and understanding.
10/11
@web3nam3
Humans can reason but don’t want to.
11/11
@adolfoasorlin
Was that a reasoning?
To post tweets in this format, more info here: https://www.thecoli.com/threads/tips-and-tricks-for-posting-the-coli-megathread.984734/post-52211196