1/1
How reasoning works in OpenAI's o1
To post tweets in this format, more info here: https://www.thecoli.com/threads/tips-and-tricks-for-posting-the-coli-megathread.984734/post-52211196
How reasoning works in OpenAI's o1
To post tweets in this format, more info here: https://www.thecoli.com/threads/tips-and-tricks-for-posting-the-coli-megathread.984734/post-52211196
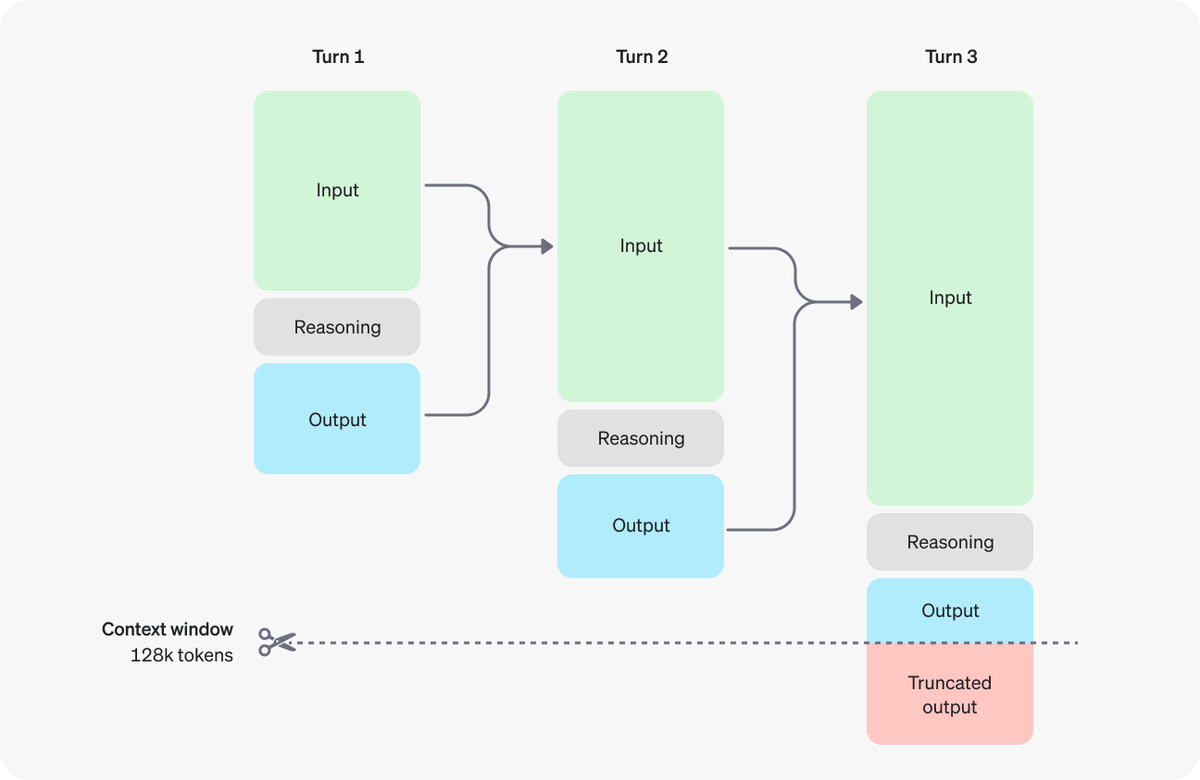
1/21
@rohanpaul_ai
How Reasoning Works in the new o1 models from @OpenAI
The key point is that reasoning allows the model to consider multiple approaches before generating final response.
OpenAI introduced reasoning tokens to "think" before responding. These tokens break down the prompt and consider multiple approaches.
Process:
1. Generate reasoning tokens
2. Produce visible completion tokens as answer
3. Discard reasoning tokens from context
Discarding reasoning tokens keeps context focused on essential information
Multi-step conversation flow:
- Input and output tokens carry over between turns
- Reasoning tokens discarded after each turn
Context window: 128k tokens
Visual representation:
- Turn 1: Input → Reasoning → Output
- Turn 2: Previous Output + New Input → Reasoning → Output
- Turn 3: Cumulative Inputs → Reasoning → Output (may be truncated)
2/21
@rohanpaul_ai
https://platform.openai.com/docs/guides/reasoning
3/21
@sameeurehman
So strawberry o1 uses chain of thought when attempting to solve problems and uses reinforcement learning to recognize and correct its mistakes. By trying a different approach when the current one isn’t working, the model’s ability to reason improves...
4/21
@rohanpaul_ai

5/21
@ddebowczyk
System 1 (gpt-4o) vs system 2 (o1) models necessitate different work paradigm: "1-1, interactive" vs "multitasking, delegated".
O1-type LLMs will require other UI than chat to make collaboration effective and satisfying:
6/21
@tonado_square
I would name this as an agent, rather than a model.
7/21
@realyashnegi
Unlike traditional models, O1 is trained using reinforcement learning, allowing it to develop internal reasoning processes. This method improves data efficiency and reasoning capabilities.
8/21
@JeffreyH630
Thanks for sharing, Rohan!
It's fascinating how these reasoning tokens enhance the model's ability to analyze and explore different perspectives.
Can’t wait to see how this evolves in future iterations!
9/21
@mathepi
I wonder if there is some sort of confirmation step going on, like a theorem prover, or something. I've tried using LLMs to check their own work in certain vision tasks and they just don't really know what they're doing; no amount of iterating and repeating really fixes it.
10/21
@AIxBlock
Nice breakdown!
11/21
@AITrailblazerQ
We have this pipeline from 6 months in ASAP.
12/21
@gpt_biz
This is a fascinating look into how AI models reason, a must-read for anyone curious about how these systems improve their responses!
13/21
@labsantai
14/21
@GenJonesX
How can quantum-like cognitive processes be empirically verified?
15/21
@AImpactSpace
16/21
@gileneo1
so it's CoT in a loop with large context window
17/21
@mycharmspace
Discard reasoning tokens actually bring inference challenges for KV cache, unless custom attention introduced
18/21
@SerisovTj
Reparsing output e?
19/21
@Just4Think
Well, I will ask again: should it be considered one model?
Should it be benchmarked as one model?
20/21
@HuajunB68287
I wonder where does the figure come from? Is it the actual logic behind o1?
21/21
@dhruv2038
Well.Just take a look here.
To post tweets in this format, more info here: https://www.thecoli.com/threads/tips-and-tricks-for-posting-the-coli-megathread.984734/post-52211196
@rohanpaul_ai
How Reasoning Works in the new o1 models from @OpenAI
The key point is that reasoning allows the model to consider multiple approaches before generating final response.


1. Generate reasoning tokens
2. Produce visible completion tokens as answer
3. Discard reasoning tokens from context


- Input and output tokens carry over between turns
- Reasoning tokens discarded after each turn


- Turn 1: Input → Reasoning → Output
- Turn 2: Previous Output + New Input → Reasoning → Output
- Turn 3: Cumulative Inputs → Reasoning → Output (may be truncated)
2/21
@rohanpaul_ai
https://platform.openai.com/docs/guides/reasoning
3/21
@sameeurehman
So strawberry o1 uses chain of thought when attempting to solve problems and uses reinforcement learning to recognize and correct its mistakes. By trying a different approach when the current one isn’t working, the model’s ability to reason improves...
4/21
@rohanpaul_ai

5/21
@ddebowczyk
System 1 (gpt-4o) vs system 2 (o1) models necessitate different work paradigm: "1-1, interactive" vs "multitasking, delegated".
O1-type LLMs will require other UI than chat to make collaboration effective and satisfying:
6/21
@tonado_square
I would name this as an agent, rather than a model.
7/21
@realyashnegi
Unlike traditional models, O1 is trained using reinforcement learning, allowing it to develop internal reasoning processes. This method improves data efficiency and reasoning capabilities.
8/21
@JeffreyH630
Thanks for sharing, Rohan!
It's fascinating how these reasoning tokens enhance the model's ability to analyze and explore different perspectives.
Can’t wait to see how this evolves in future iterations!
9/21
@mathepi
I wonder if there is some sort of confirmation step going on, like a theorem prover, or something. I've tried using LLMs to check their own work in certain vision tasks and they just don't really know what they're doing; no amount of iterating and repeating really fixes it.
10/21
@AIxBlock
Nice breakdown!
11/21
@AITrailblazerQ
We have this pipeline from 6 months in ASAP.
12/21
@gpt_biz
This is a fascinating look into how AI models reason, a must-read for anyone curious about how these systems improve their responses!
13/21
@labsantai
14/21
@GenJonesX
How can quantum-like cognitive processes be empirically verified?
15/21
@AImpactSpace
16/21
@gileneo1
so it's CoT in a loop with large context window
17/21
@mycharmspace
Discard reasoning tokens actually bring inference challenges for KV cache, unless custom attention introduced
18/21
@SerisovTj
Reparsing output e?

19/21
@Just4Think
Well, I will ask again: should it be considered one model?
Should it be benchmarked as one model?
20/21
@HuajunB68287
I wonder where does the figure come from? Is it the actual logic behind o1?
21/21
@dhruv2038
Well.Just take a look here.
To post tweets in this format, more info here: https://www.thecoli.com/threads/tips-and-tricks-for-posting-the-coli-megathread.984734/post-52211196
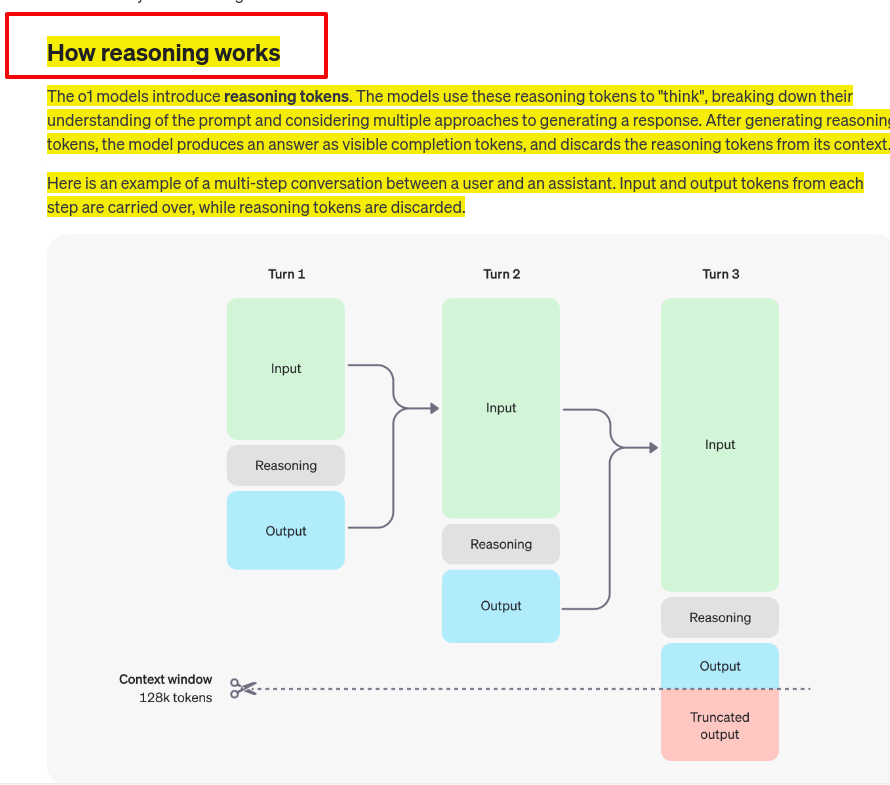
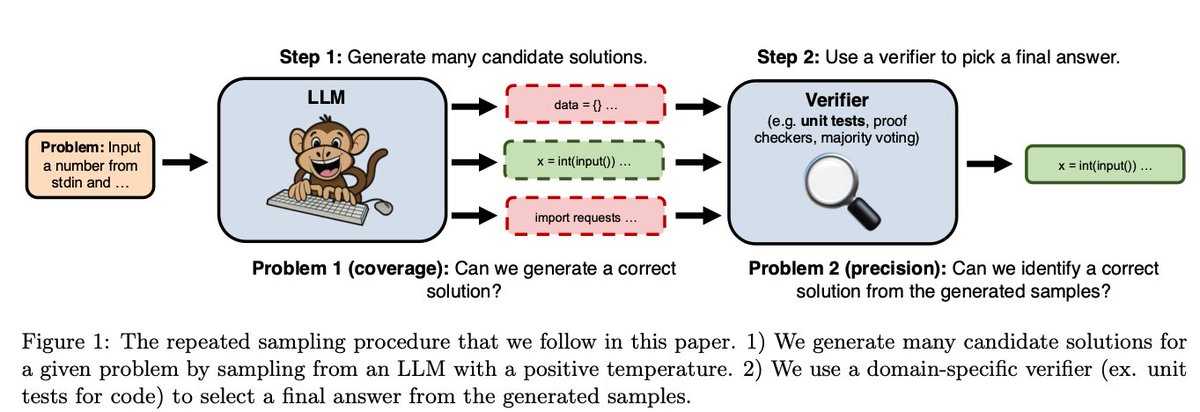
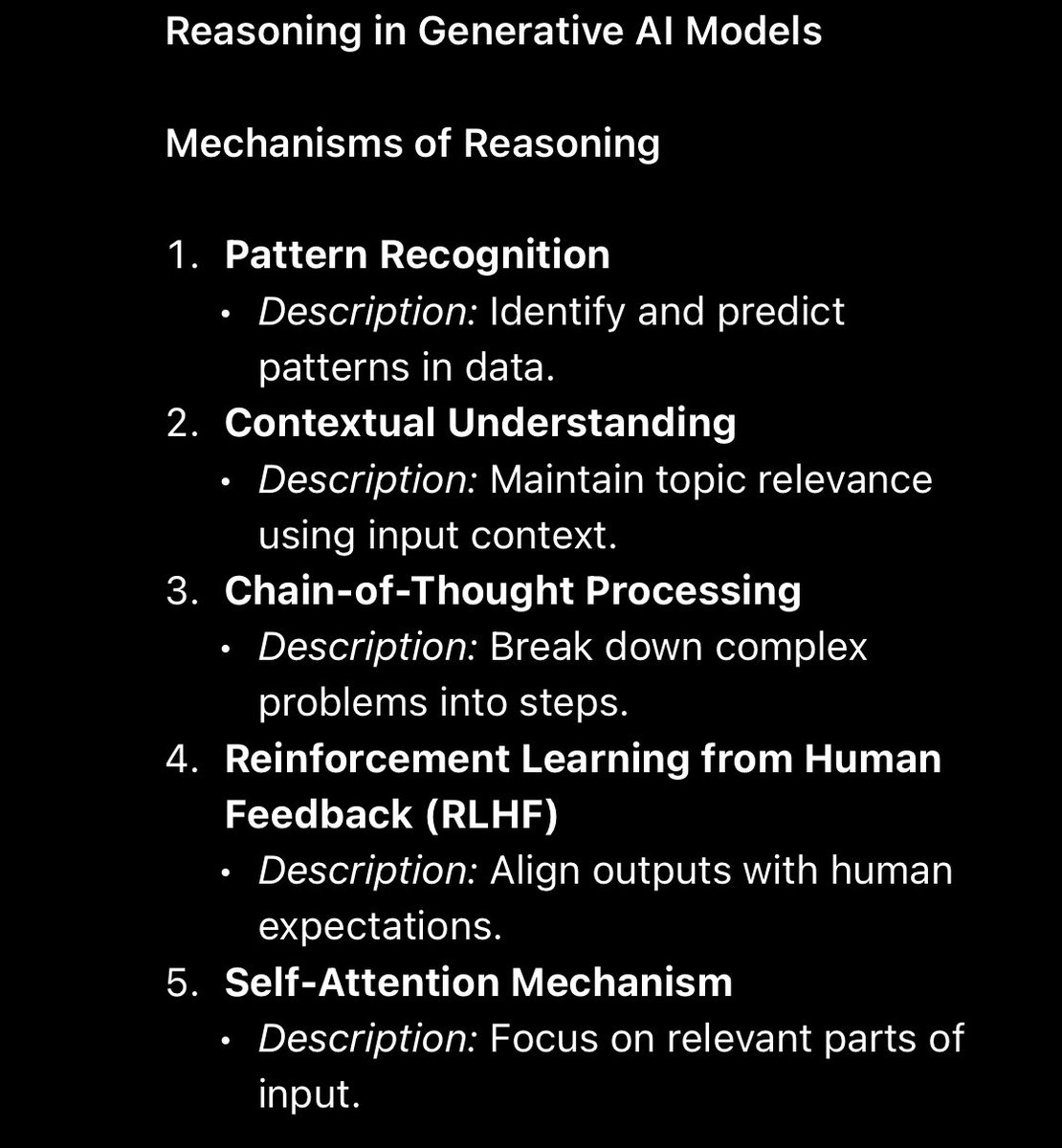
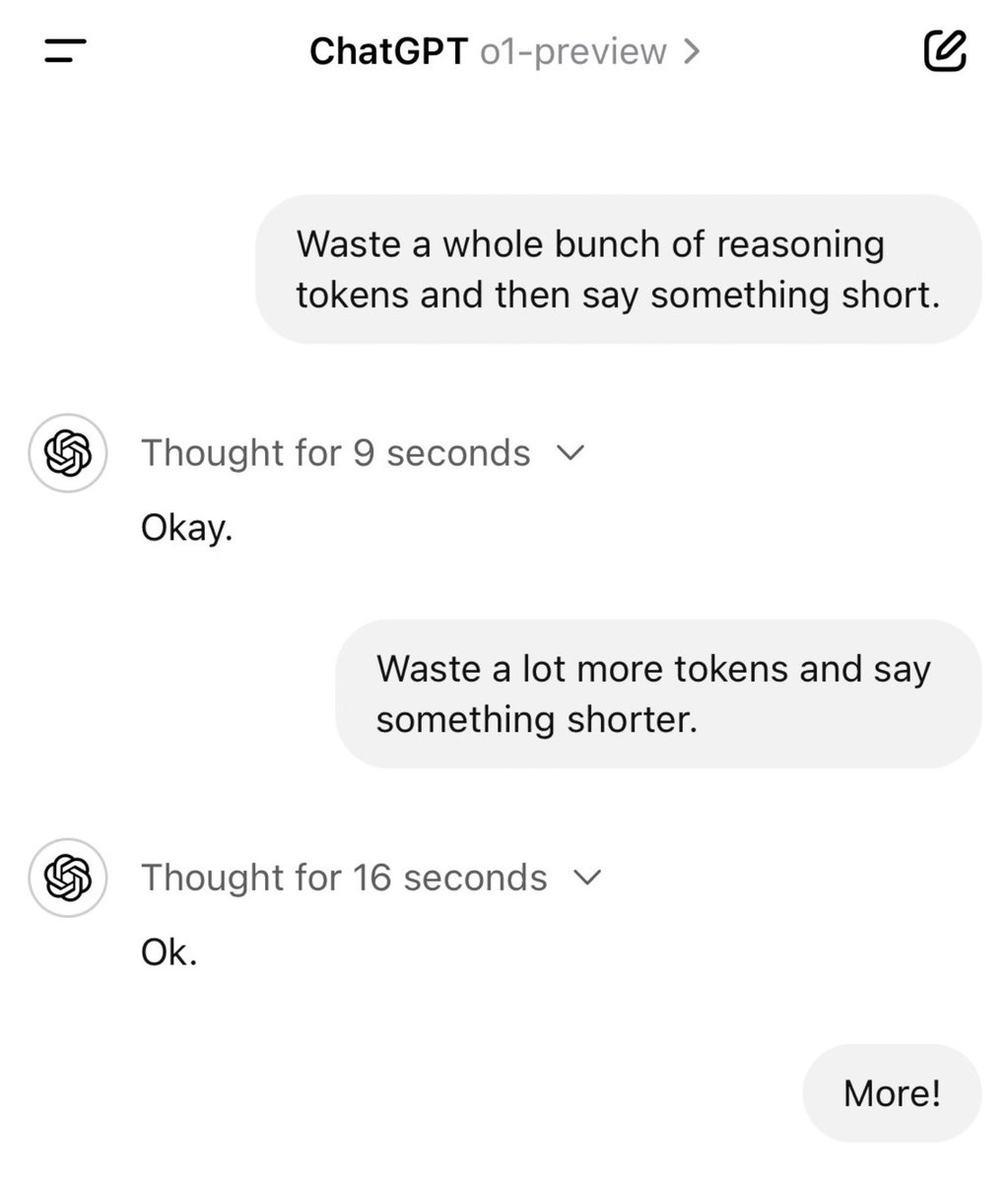