1/11
This paper seems very interesting: say you train an LLM to play chess using only transcripts of games of players up to 1000 elo. Is it possible that the model plays better than 1000 elo? (i.e. "transcends" the training data performance?). It seems you get something from nothing, and some information theory arguments that this should be impossible were discussed in conversations I had in the past. But this paper shows this can happen: training on 1000 elo game transcripts and getting an LLM that plays at 1500! Further the authors connect to a clean theoretical framework for why: it's ensembling weak learners, where you get "something from nothing" by averaging the independent mistakes of multiple models. The paper argued that you need enough data diversity and careful temperature sampling for the transcendence to occur. I had been thinking along the same lines but didn't think of using chess as a clean measurable way to scientifically measure this. Fantastic work that I'll read I'll more depth.
2/11
[2406.11741v1] Transcendence: Generative Models Can Outperform The Experts That Train Them paper is here. @ShamKakade6 @nsaphra please tell me if I have any misconceptions.
3/11
In the classic "Human Decisions and Machine Predictions" paper Kleinberg et al. give evidence that a predictor learned from the bail decisions of multiple judges does better than the judges themselves, calling it a wisdom of the crowd effect. This could be a similar phenomena
4/11
Yes that is what the authors formalize. Only works when there is diversity in the weak learners ie they make different types of mistakes independently.
5/11
It seems very straightforward: a 1000 ELO player makes good and bad moves that average to 1000. A learning process is a max of the quality of moves, so you should get a higher than 1000 rating. I wonder if the AI is more consistent in making "1500 ELO" moves than players.
6/11
Any argument that says it's not surprising must also explain why it didn't happen at 1500 elo training, or why it doesn't happen at higher temperatures.
7/11
The idea might be easier to understand for something that’s more of a motor skill like archery. Imagine building a dataset of humans shooting arrows at targets and then imitating only the examples where they hit the targets.
8/11
Yes but they never have added information on what a better move is or who won, as far as I understood. Unclear if the LLM is even trying to win.
9/11
Interesting - is majority vote by a group of weak learners a form of “verification” as I describe in this thread?
10/11
I don't think it's verification, ie they didn't use signal of who won in each game. It's clear you can use that to filter only better (winning) player transcripts , train on that, iterate to get stronger elo transcripts and repeat. But this phenomenon is different, I think. It's ensembling weak learners. The cleanest setting to understand ensembling: Imagine if I have 1000 binary classifiers, each correct with 60 percent probability and *Independent*. If I make a new classifier by taking majority, it will perform much better than 60 percent. It's concentration of measure, the key tool for information theory too. The surprising experimental findings are 1. this happens with elo 1000 chess players where I wouldn't think they make independent mistakes. 2. Training on transcripts seems to behave like averaging weak learners.
11/11
Interesting
To post tweets in this format, more info here: https://www.thecoli.com/threads/tips-and-tricks-for-posting-the-coli-megathread.984734/post-52211196
This paper seems very interesting: say you train an LLM to play chess using only transcripts of games of players up to 1000 elo. Is it possible that the model plays better than 1000 elo? (i.e. "transcends" the training data performance?). It seems you get something from nothing, and some information theory arguments that this should be impossible were discussed in conversations I had in the past. But this paper shows this can happen: training on 1000 elo game transcripts and getting an LLM that plays at 1500! Further the authors connect to a clean theoretical framework for why: it's ensembling weak learners, where you get "something from nothing" by averaging the independent mistakes of multiple models. The paper argued that you need enough data diversity and careful temperature sampling for the transcendence to occur. I had been thinking along the same lines but didn't think of using chess as a clean measurable way to scientifically measure this. Fantastic work that I'll read I'll more depth.
2/11
[2406.11741v1] Transcendence: Generative Models Can Outperform The Experts That Train Them paper is here. @ShamKakade6 @nsaphra please tell me if I have any misconceptions.
3/11
In the classic "Human Decisions and Machine Predictions" paper Kleinberg et al. give evidence that a predictor learned from the bail decisions of multiple judges does better than the judges themselves, calling it a wisdom of the crowd effect. This could be a similar phenomena
4/11
Yes that is what the authors formalize. Only works when there is diversity in the weak learners ie they make different types of mistakes independently.
5/11
It seems very straightforward: a 1000 ELO player makes good and bad moves that average to 1000. A learning process is a max of the quality of moves, so you should get a higher than 1000 rating. I wonder if the AI is more consistent in making "1500 ELO" moves than players.
6/11
Any argument that says it's not surprising must also explain why it didn't happen at 1500 elo training, or why it doesn't happen at higher temperatures.
7/11
The idea might be easier to understand for something that’s more of a motor skill like archery. Imagine building a dataset of humans shooting arrows at targets and then imitating only the examples where they hit the targets.
8/11
Yes but they never have added information on what a better move is or who won, as far as I understood. Unclear if the LLM is even trying to win.
9/11
Interesting - is majority vote by a group of weak learners a form of “verification” as I describe in this thread?
10/11
I don't think it's verification, ie they didn't use signal of who won in each game. It's clear you can use that to filter only better (winning) player transcripts , train on that, iterate to get stronger elo transcripts and repeat. But this phenomenon is different, I think. It's ensembling weak learners. The cleanest setting to understand ensembling: Imagine if I have 1000 binary classifiers, each correct with 60 percent probability and *Independent*. If I make a new classifier by taking majority, it will perform much better than 60 percent. It's concentration of measure, the key tool for information theory too. The surprising experimental findings are 1. this happens with elo 1000 chess players where I wouldn't think they make independent mistakes. 2. Training on transcripts seems to behave like averaging weak learners.
11/11
Interesting
To post tweets in this format, more info here: https://www.thecoli.com/threads/tips-and-tricks-for-posting-the-coli-megathread.984734/post-52211196
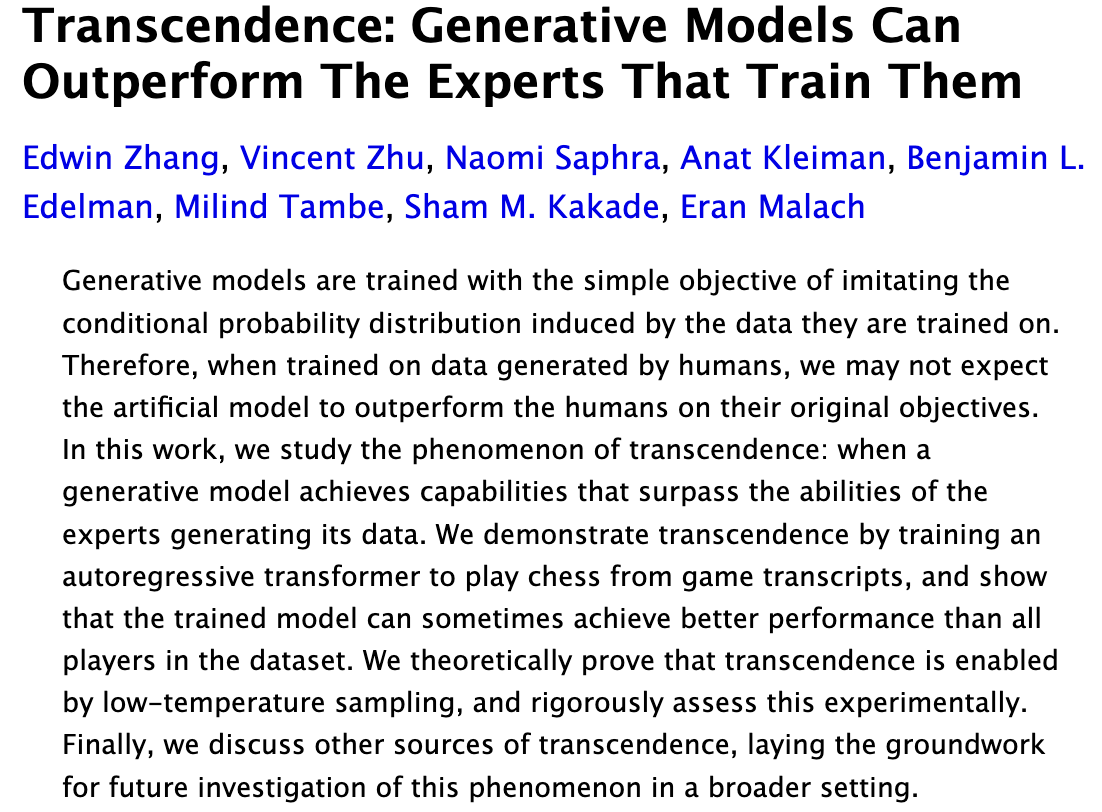
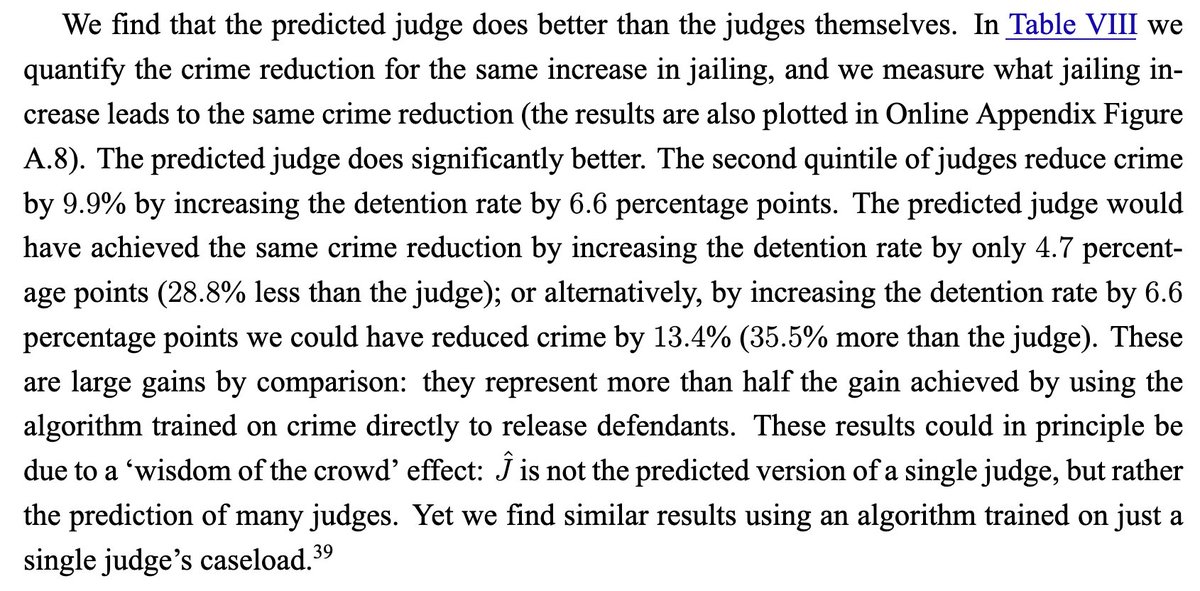