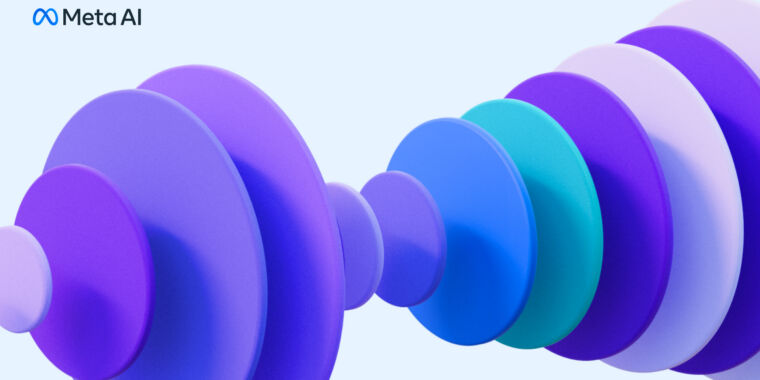
Meta releases open source AI audio tools, AudioCraft
Meta's suite of three AI models can create sound effects and music from descriptions.
Meta releases open source AI audio tools, AudioCraft
Meta's suite of three AI models can create sound effects and music from descriptions.
BENJ EDWARDS - Wednesday at undefined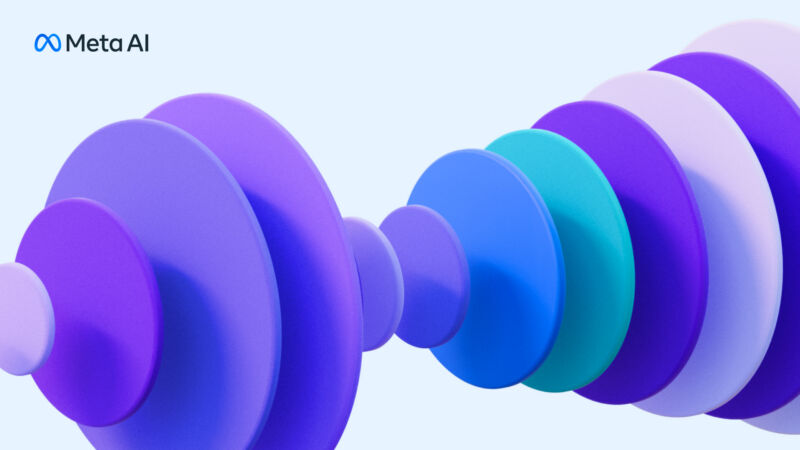
On Wednesday, Meta announced it is open-sourcing AudioCraft, a suite of generative AI tools for creating music and audio from text prompts. With the tools, content creators can input simple text descriptions to generate complex audio landscapes, compose melodies, or even simulate entire virtual orchestras.
AudioCraft consists of three core components: AudioGen, a tool for generating various audio effects and soundscapes; MusicGen, which can create musical compositions and melodies from descriptions; and EnCodec, a neural network-based audio compression codec.
In particular, Meta says that EnCodec, which we first covered in November, has recently been improved and allows for "higher quality music generation with fewer artifacts." Also, AudioGen can create audio sound effects like a dog barking, a car horn honking, or footsteps on a wooden floor. And MusicGen can whip up songs of various genres from scratch, based on descriptions like "Pop dance track with catchy melodies, tropical percussions, and upbeat rhythms, perfect for the beach."
Meta has provided several audio samples on its website for evaluation. The results seem in line with their state-of-the-art labeling, but arguably they aren't quite high quality enough to replace professionally produced commercial audio effects or music.
Meta notes that while generative AI models centered around text and still pictures have received lots of attention (and are relatively easy for people to experiment with online), development in generative audio tools has lagged behind. "There’s some work out there, but it’s highly complicated and not very open, so people aren’t able to readily play with it," they write. But they hope that AudioCraft's release under the MIT License will contribute to the broader community by providing accessible tools for audio and musical experimentation.
"The models are available for research purposes and to further people’s understanding of the technology. We’re excited to give researchers and practitioners access so they can train their own models with their own datasets for the first time and help advance the state of the art," Meta said.
Meta isn't the first company to experiment with AI-powered audio and music generators. Among some of the more notable recent attempts, OpenAI debuted its Jukebox in 2020, Google debuted MusicLM in January, and last December, an independent research team created a text-to-music generation platform called Riffusion using a Stable Diffusion base.
None of these generative audio projects have attracted as much attention as image synthesis models, but that doesn't mean the process of developing them isn't any less complicated, as Meta notes on its website:
Amid controversy over undisclosed and potentially unethical training material used to create image synthesis models such as Stable Diffusion, DALL-E, and Midjourney, it's notable that Meta says that MusicGen was trained on "20,000 hours of music owned by Meta or licensed specifically for this purpose." On its surface, that seems like a move in a more ethical direction that may please some critics of generative AI.Generating high-fidelity audio of any kind requires modeling complex signals and patterns at varying scales. Music is arguably the most challenging type of audio to generate because it’s composed of local and long-range patterns, from a suite of notes to a global musical structure with multiple instruments. Generating coherent music with AI has often been addressed through the use of symbolic representations like MIDI or piano rolls. However, these approaches are unable to fully grasp the expressive nuances and stylistic elements found in music. More recent advances leverage self-supervised audio representation learning and a number of hierarchical or cascaded models to generate music, feeding the raw audio into a complex system in order to capture long-range structures in the signal while generating quality audio. But we knew that more could be done in this field.
It will be interesting to see how open source developers choose to integrate these Meta audio models in their work. It may result in some interesting and easy-to-use generative audio tools in the near future. For now, the more code-savvy among us can find model weights and code for the three AudioCraft tools on GitHub.
GitHub - facebookresearch/audiocraft: Audiocraft is a library for audio processing and generation with deep learning. It features the state-of-the-art EnCodec audio compressor / tokenizer, along with MusicGen, a simple and controllable music generati
Audiocraft is a library for audio processing and generation with deep learning. It features the state-of-the-art EnCodec audio compressor / tokenizer, along with MusicGen, a simple and controllable...