Google announces PALP Prompt Aligned Personalization of Text-to-Image Models
paper page: Paper page - PALP: Prompt Aligned Personalization of Text-to-Image Models
Content creators often aim to create personalized images using personal subjects that go beyond the capabilities of conventional text-to-image models. Additionally, they may want the resulting image to encompass a specific location, style, ambiance, and more. Existing personalization methods may compromise personalization ability or the alignment to complex textual prompts. This trade-off can impede the fulfillment of user prompts and subject fidelity. We propose a new approach focusing on personalization methods for a single prompt to address this issue. We term our approach prompt-aligned personalization. While this may seem restrictive, our method excels in improving text alignment, enabling the creation of images with complex and intricate prompts, which may pose a challenge for current techniques. In particular, our method keeps the personalized model aligned with a target prompt using an additional score distillation sampling term. We demonstrate the versatility of our method in multi- and single-shot settings and further show that it can compose multiple subjects or use inspiration from reference images, such as artworks. We compare our approach quantitatively and qualitatively with existing baselines and state-of-the-art techniques.

PALP

TL;DR
Prompt aligned personalization allow rich and complex scene generation, including all elements of a condition prompt (right).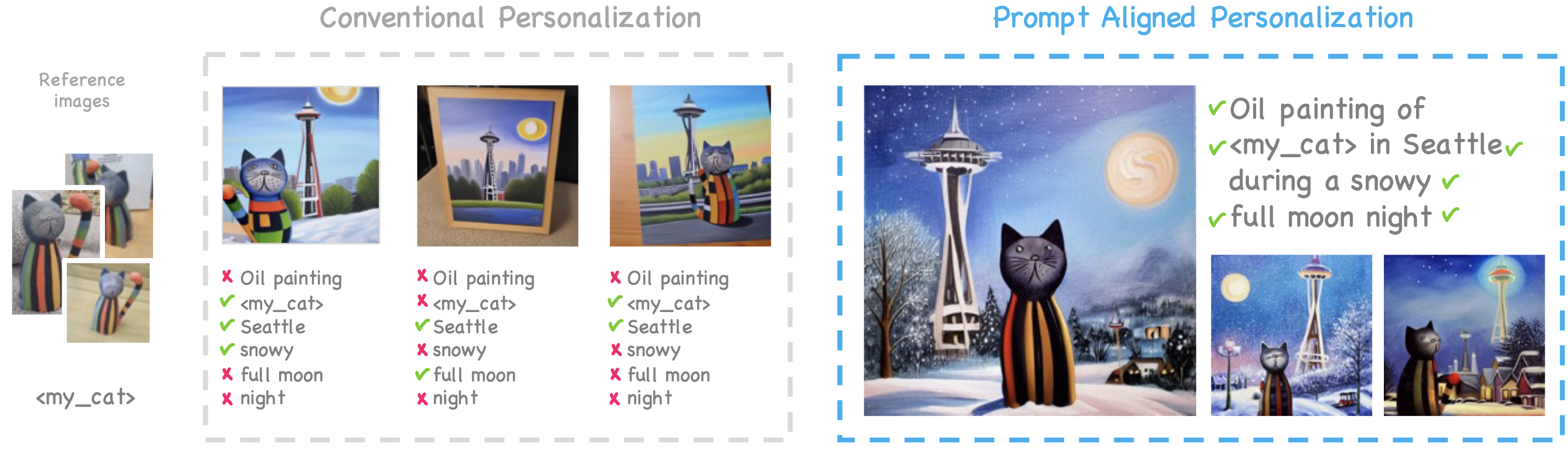
Abstract
Content creators often aim to create personalized images using personal subjects that go beyond the capabilities of conventional text-to-image models. Additionally, they may want the resulting image to encompass a specific location, style, ambiance, and more. Existing personalization methods may compromise personalization ability or the alignment to complex textual prompts. This trade-off can impede the fulfillment of user prompts and subject fidelity. We propose a new approach focusing on personalization methods for a single prompt to address this issue. We term our approach prompt-aligned personalization. While this may seem restrictive, our method excels in improving text alignment, enabling the creation of images with complex and intricate prompts, which may pose a challenge for current techniques. In particular, our method keeps the personalized model aligned with a target prompt using an additional score distillation sampling term. We demonstrate the versatility of our method in multi- and single-shot settings and further show that it can compose multiple subjects or use inspiration from reference images, such as artworks. We compare our approach quantitatively and qualitatively with existing baselines and state-of-the-art techniques.How does it work?
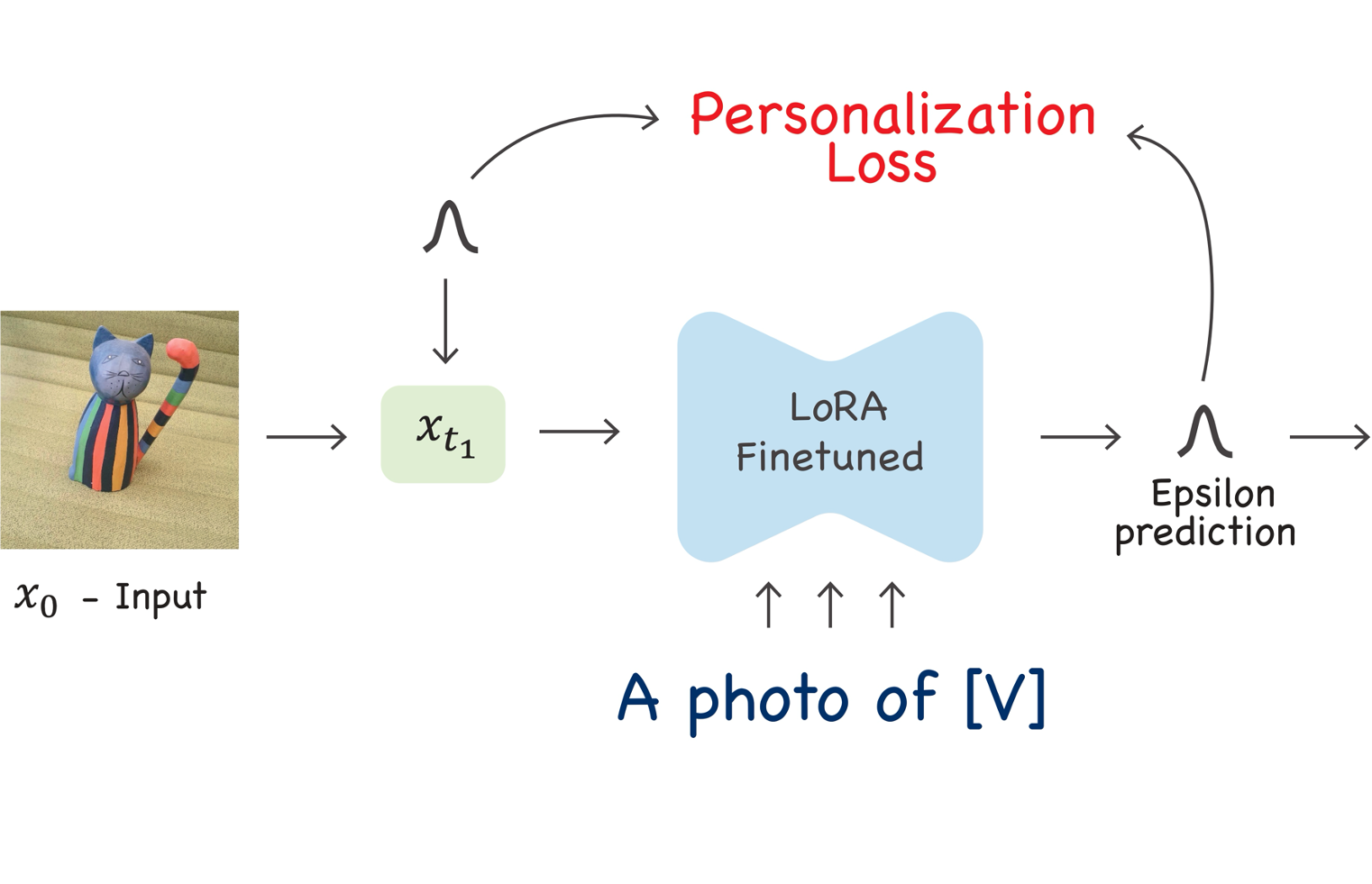
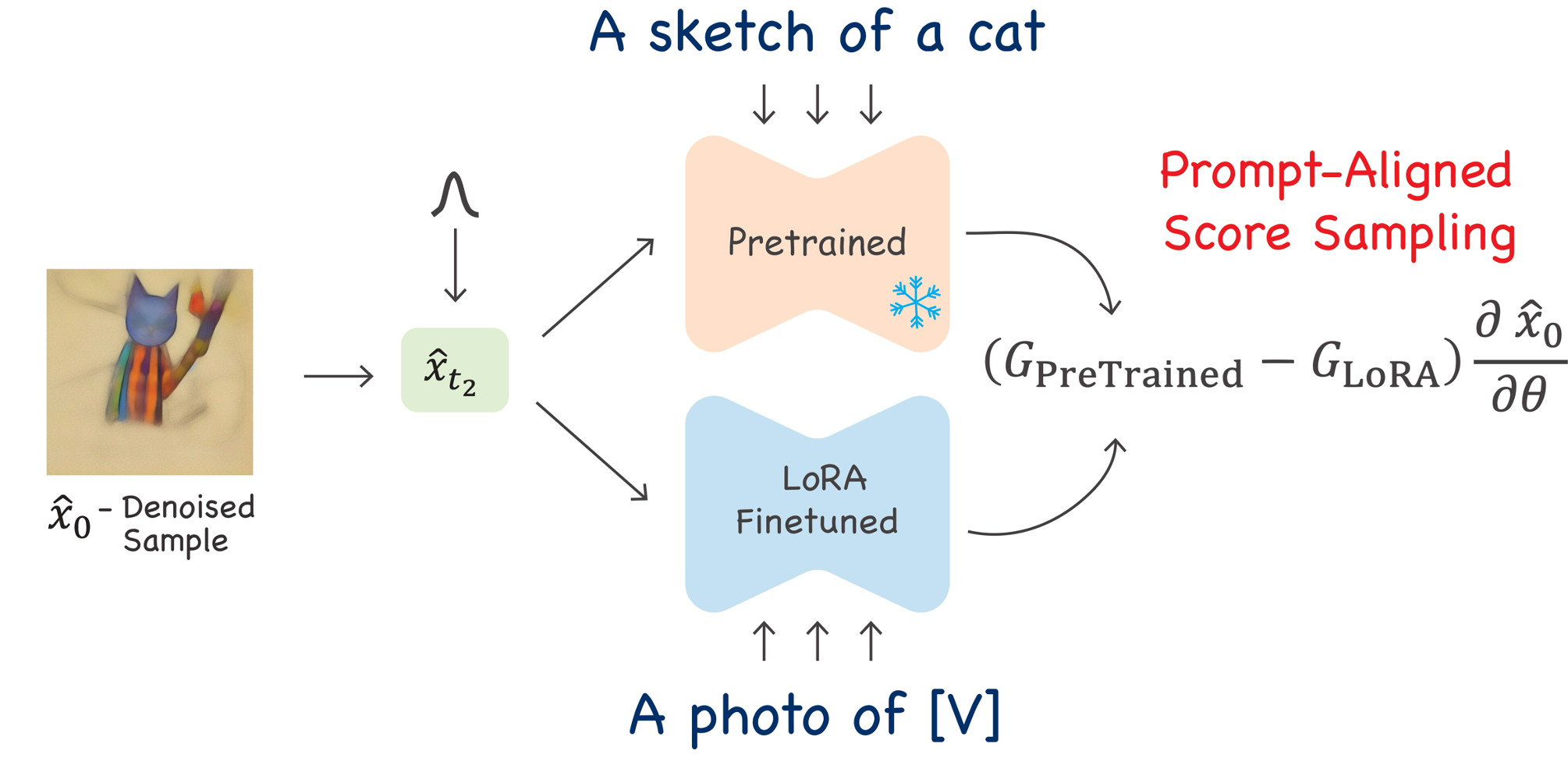
- Personalization: We achieve personalization by fine-tuning the pre-trained model using a simple reconstruction loss. We used DreamBooth-LoRA and TextualInversion personalization methods; however, any personalization method would work.
- Prompt-Alignment: To keep the model aligned with the target prompt, we use score sampling to pivot the prediction towards the direction of the target prompt, e.g., ”A sketch of a cat.”
Comparison to previous works
PALP excels with complex prompts with multiple elements, including style, ambiance, places, etc. For each subject, we show a single exemplar from the training set, conditioning text, and comparisons to Dreambooth and Textual-Inversion, Custom-Diffusion, NeTI, and P+ baselines.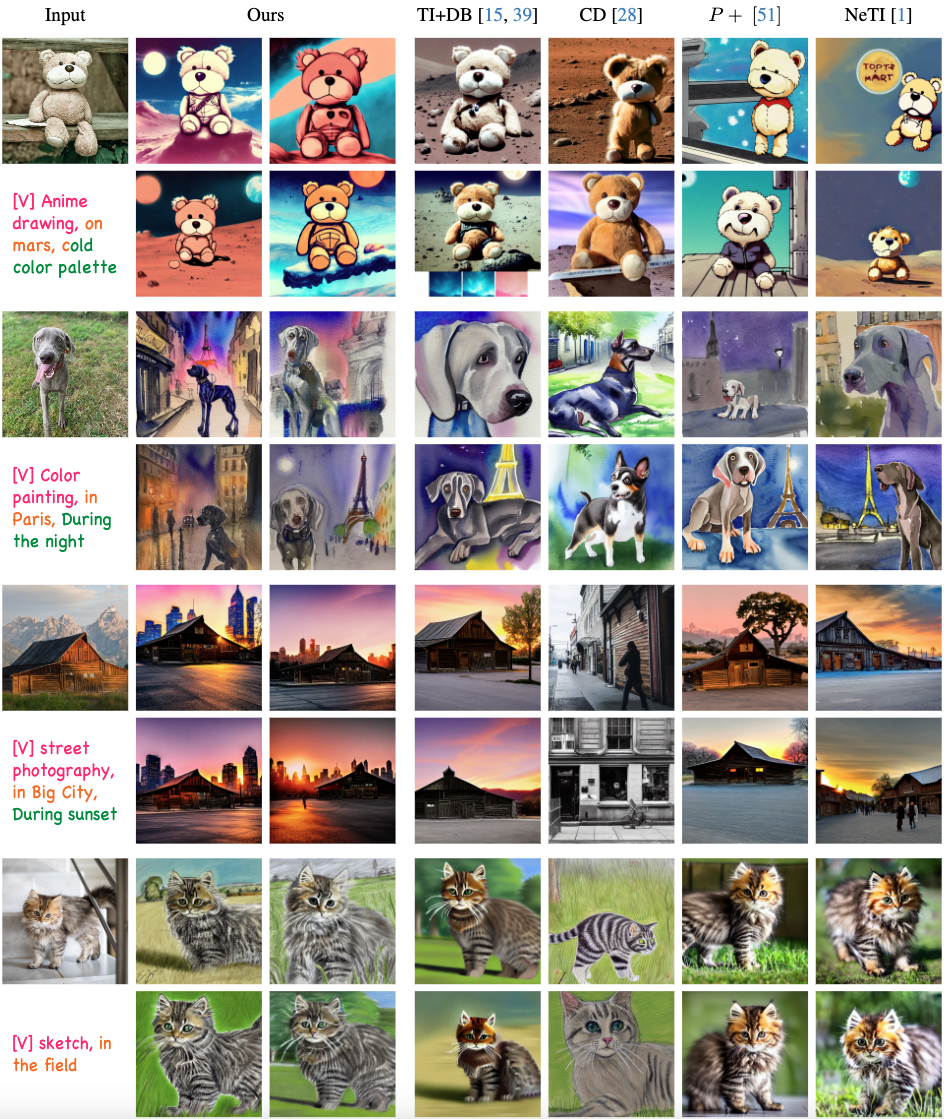
Art Inspired Personalization
PALP can generate scenes inspired by a single artistic image by ensuring alignment with the target prompt, ""An oil painting of a [toy / cat]"."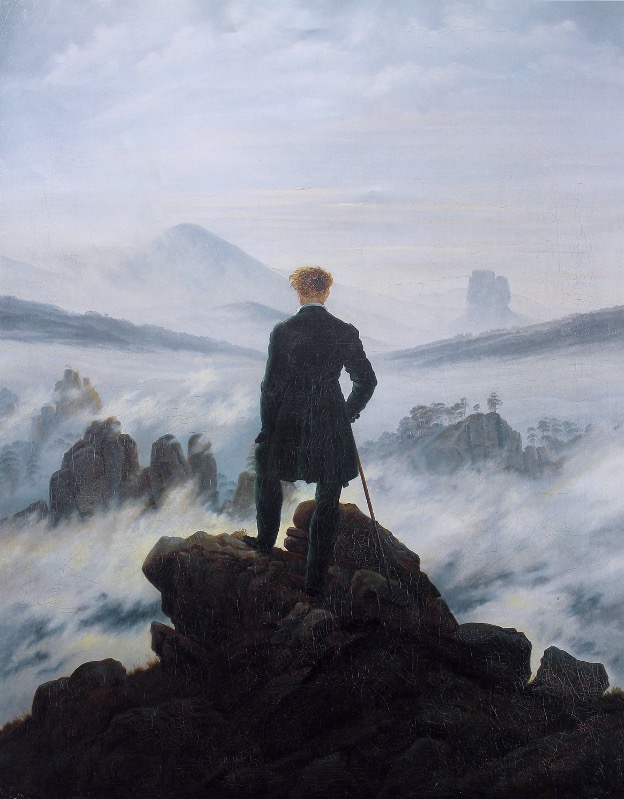
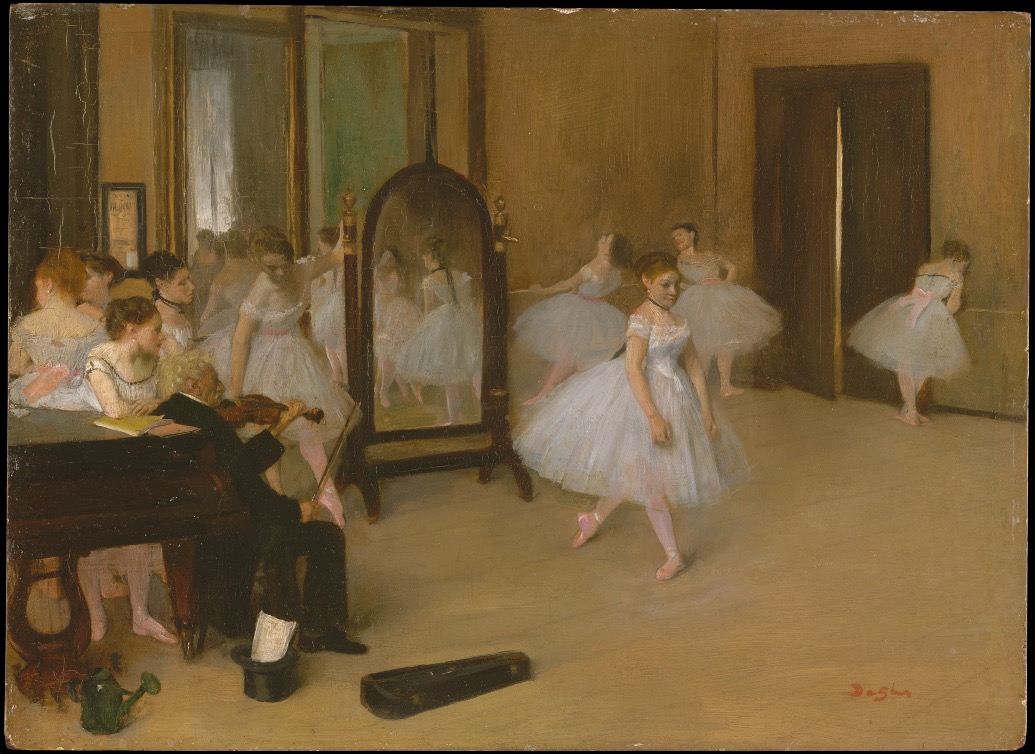
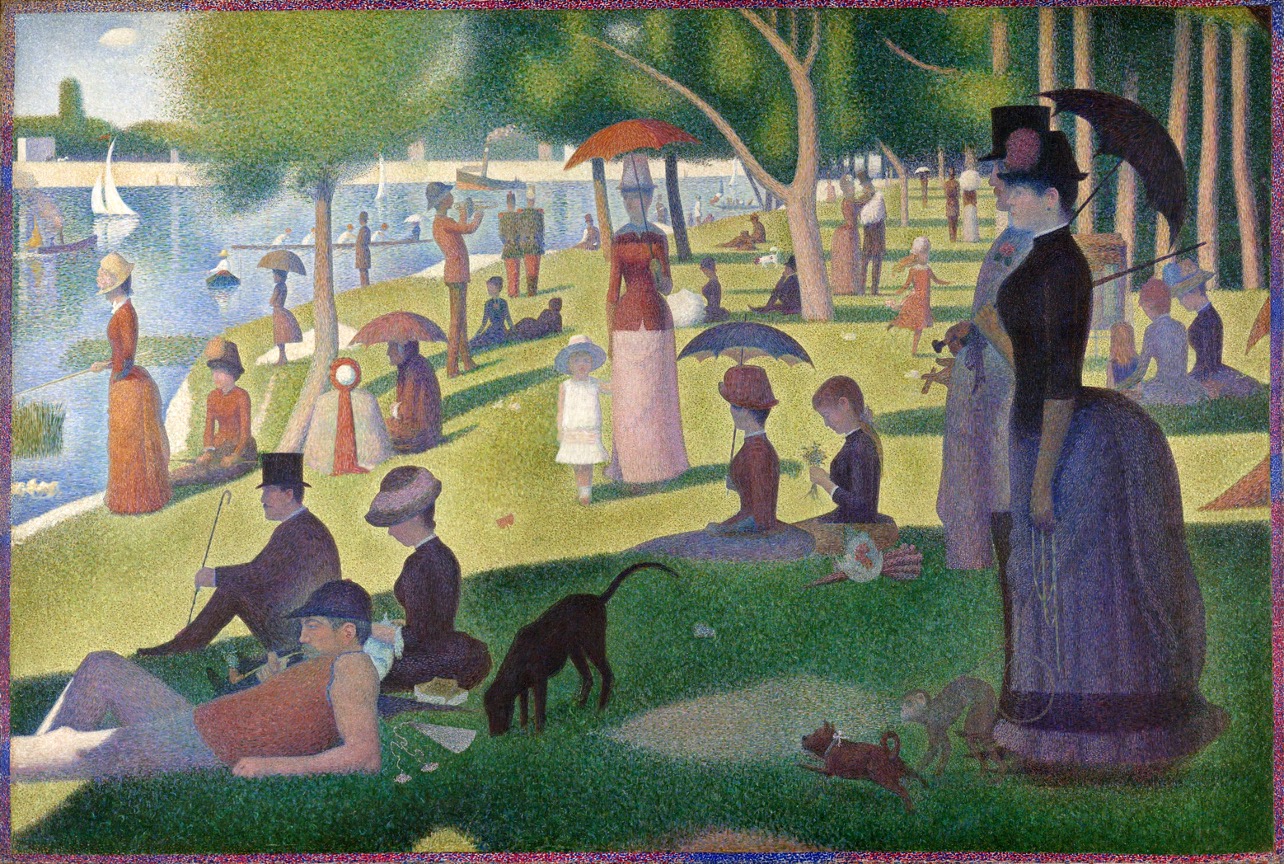
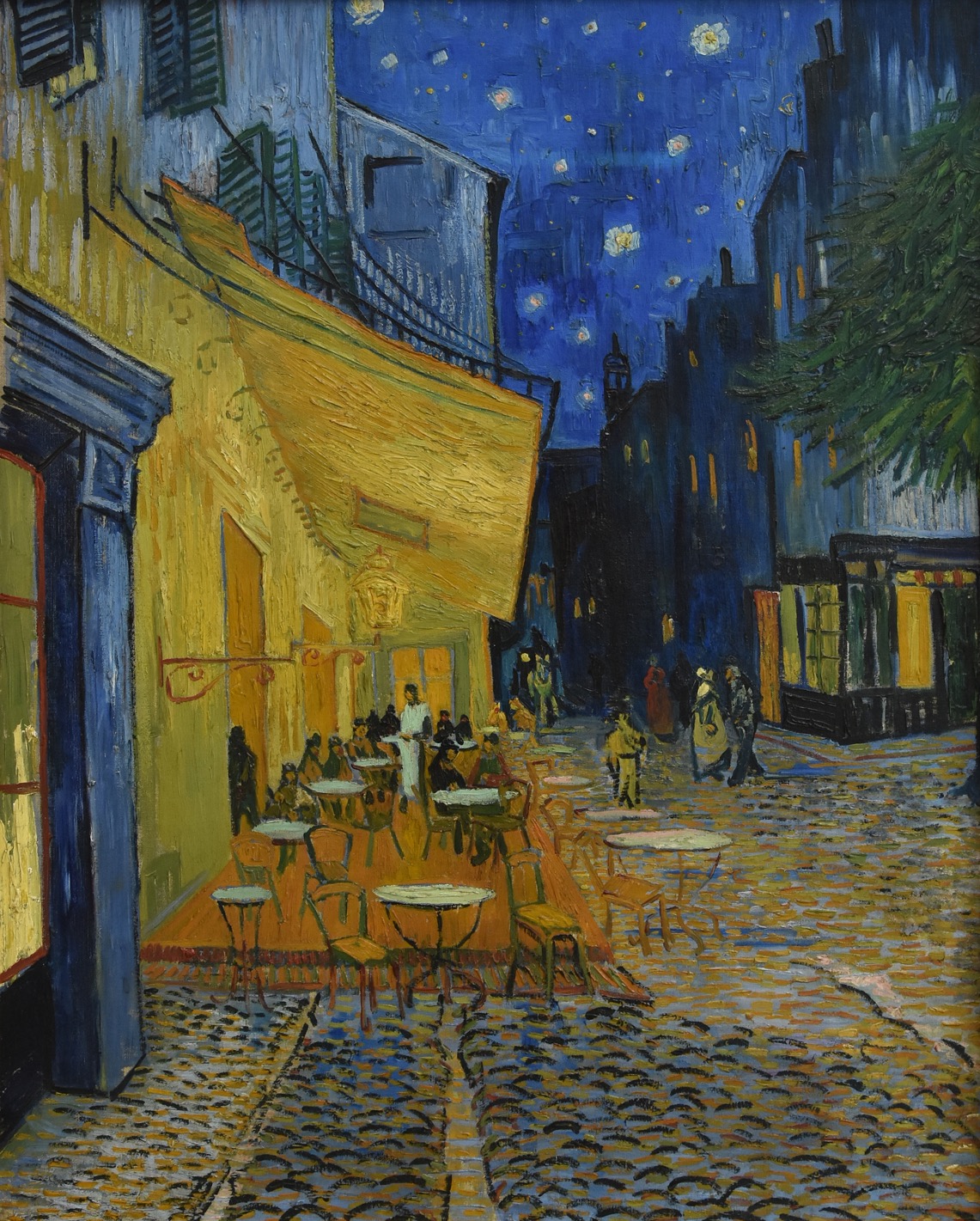
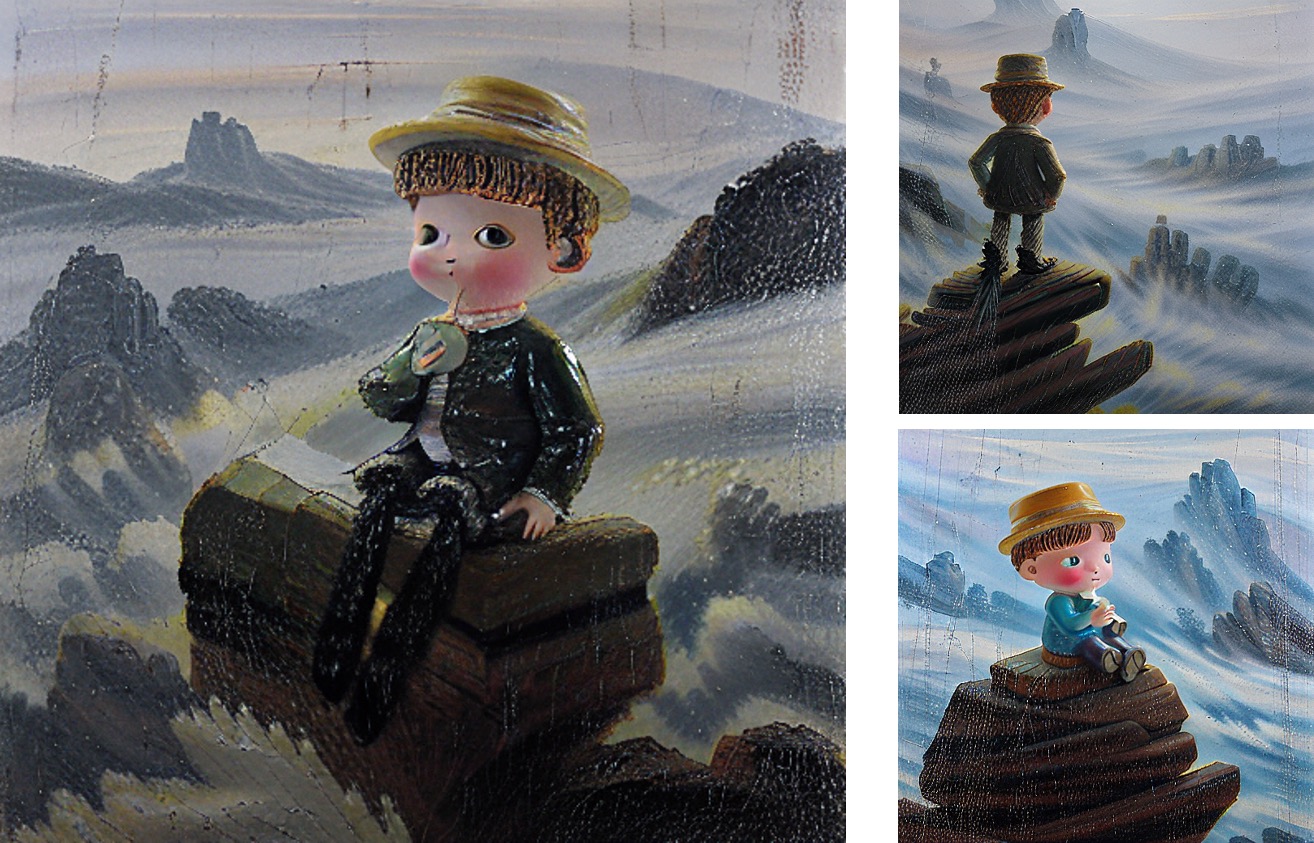
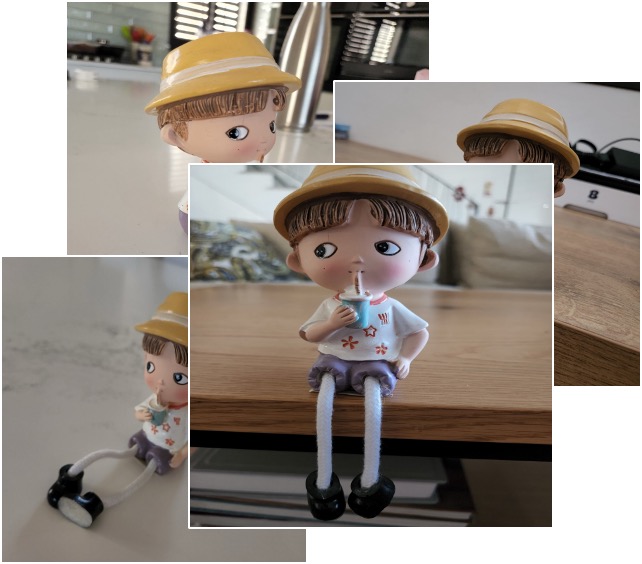
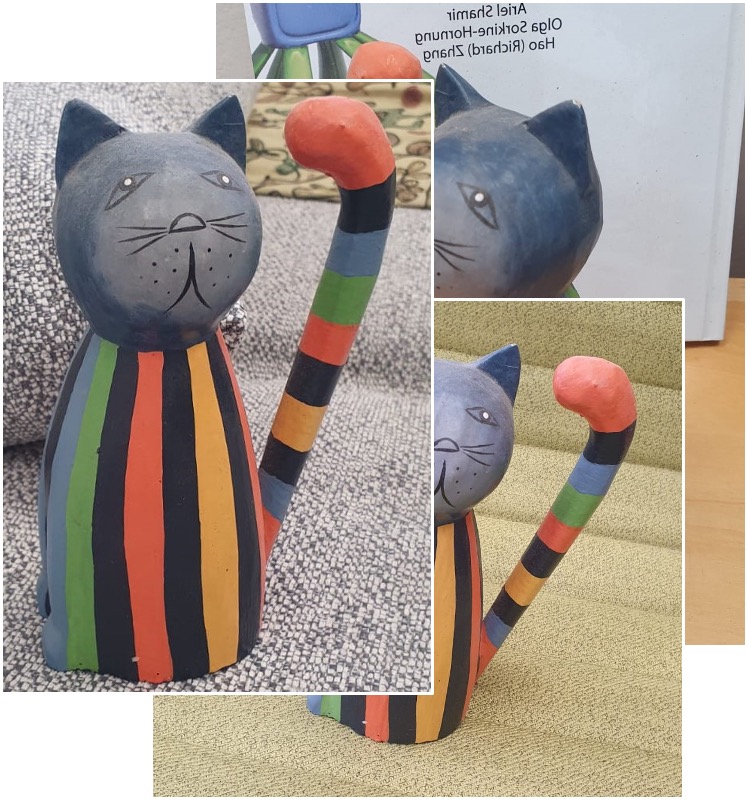
Single-Image Personalization
PALP achieves high fidelity and prompt-aligned even from a single reference image. Here we present a un-curated results of eight randomly sampled noises.1 / 5
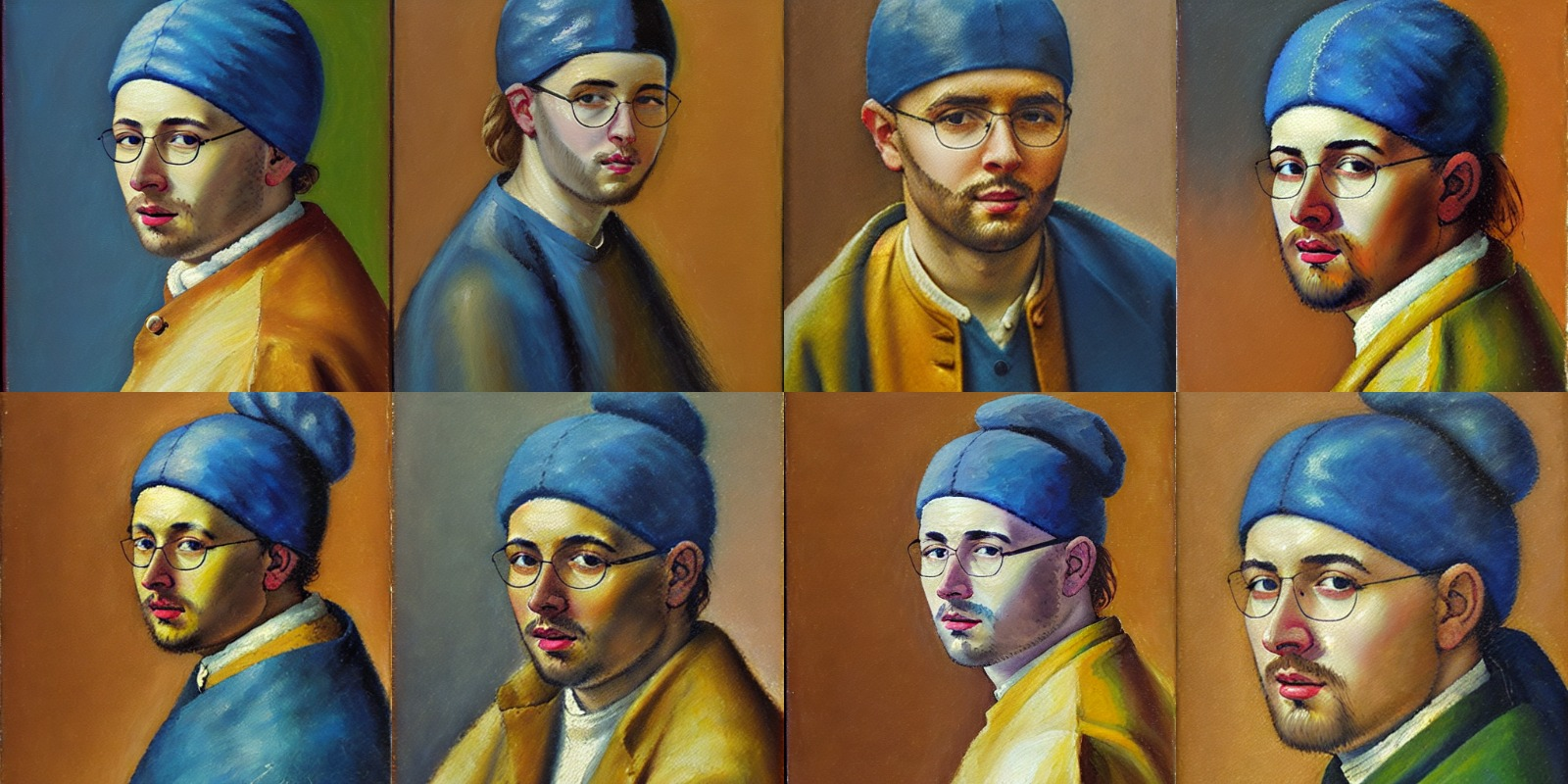
Vermeer
❮❯