Generating Illustrated Instructions
Generating illustrated instructions for AI-generated instructional articles.
facebookresearch.github.io
Generating Illustrated Instructions
Our method, StackedDiffusion, addresses the new task of generating illustrated instructions for any user query.
Abstract
We introduce the new task of generating Illustrated Instructions, i.e., visual instructions customized to a user's needs.
We identify desiderata unique to this task, and formalize it through a suite of automatic and human evaluation metrics, designed to measure the validity, consistency, and efficacy of the generations. We combine the power of large language models (LLMs) together with strong text-to-image generation diffusion models to propose a simple approach called StackedDiffusion, which generates such illustrated instructions given text as input. The resulting model strongly outperforms baseline approaches and state-of-the-art multimodal LLMs; and in 30% of cases, users even prefer it to human-generated articles.
Most notably, it enables various new and exciting applications far beyond what static articles on the web can provide, such as personalized instructions complete with intermediate steps and pictures in response to a user's individual situation.
We identify desiderata unique to this task, and formalize it through a suite of automatic and human evaluation metrics, designed to measure the validity, consistency, and efficacy of the generations. We combine the power of large language models (LLMs) together with strong text-to-image generation diffusion models to propose a simple approach called StackedDiffusion, which generates such illustrated instructions given text as input. The resulting model strongly outperforms baseline approaches and state-of-the-art multimodal LLMs; and in 30% of cases, users even prefer it to human-generated articles.
Most notably, it enables various new and exciting applications far beyond what static articles on the web can provide, such as personalized instructions complete with intermediate steps and pictures in response to a user's individual situation.
Overview
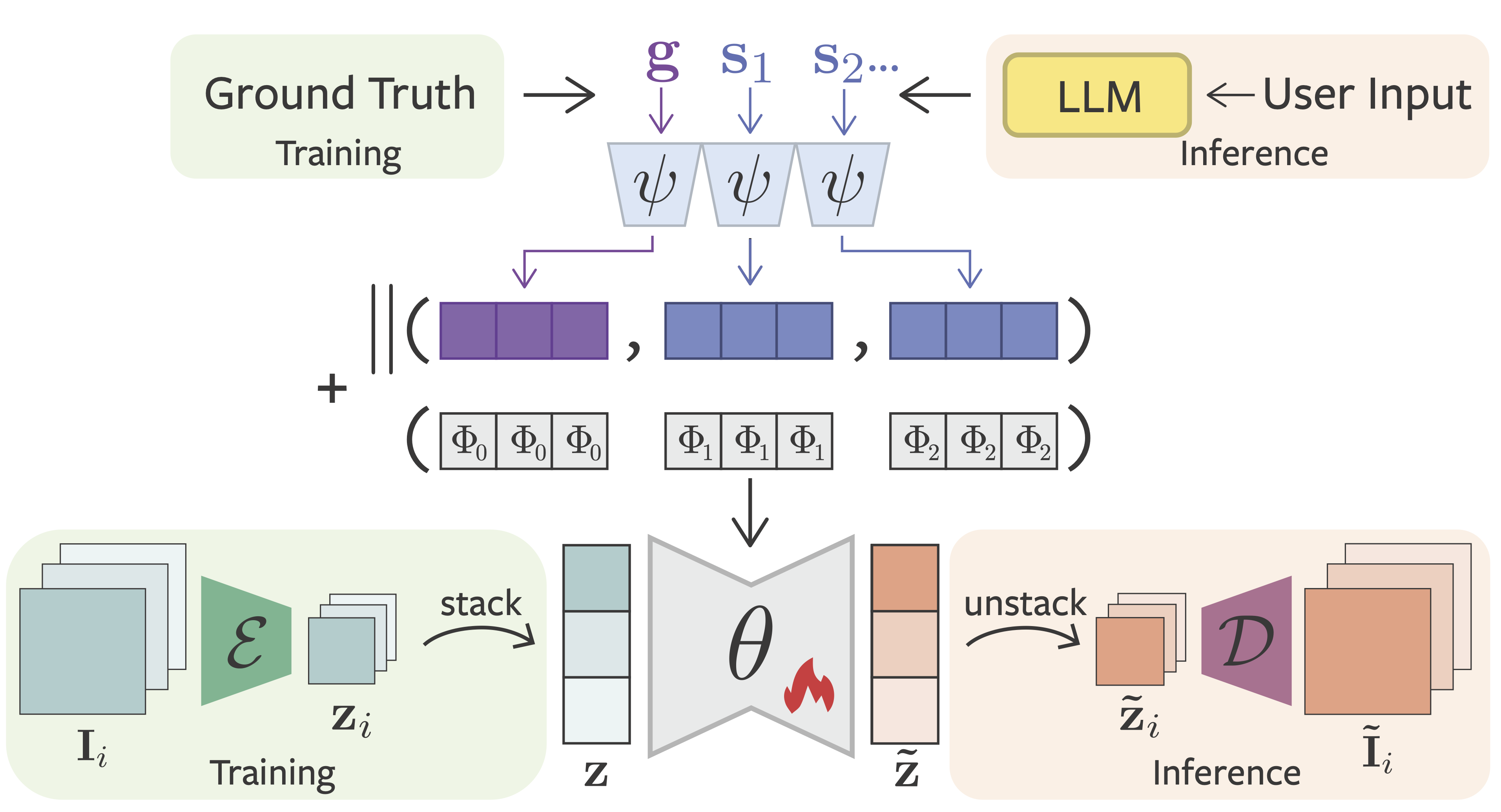
Applications
Error Correction
StackedDiffusion provides updated instructions in response to unexpected situations, like a user error.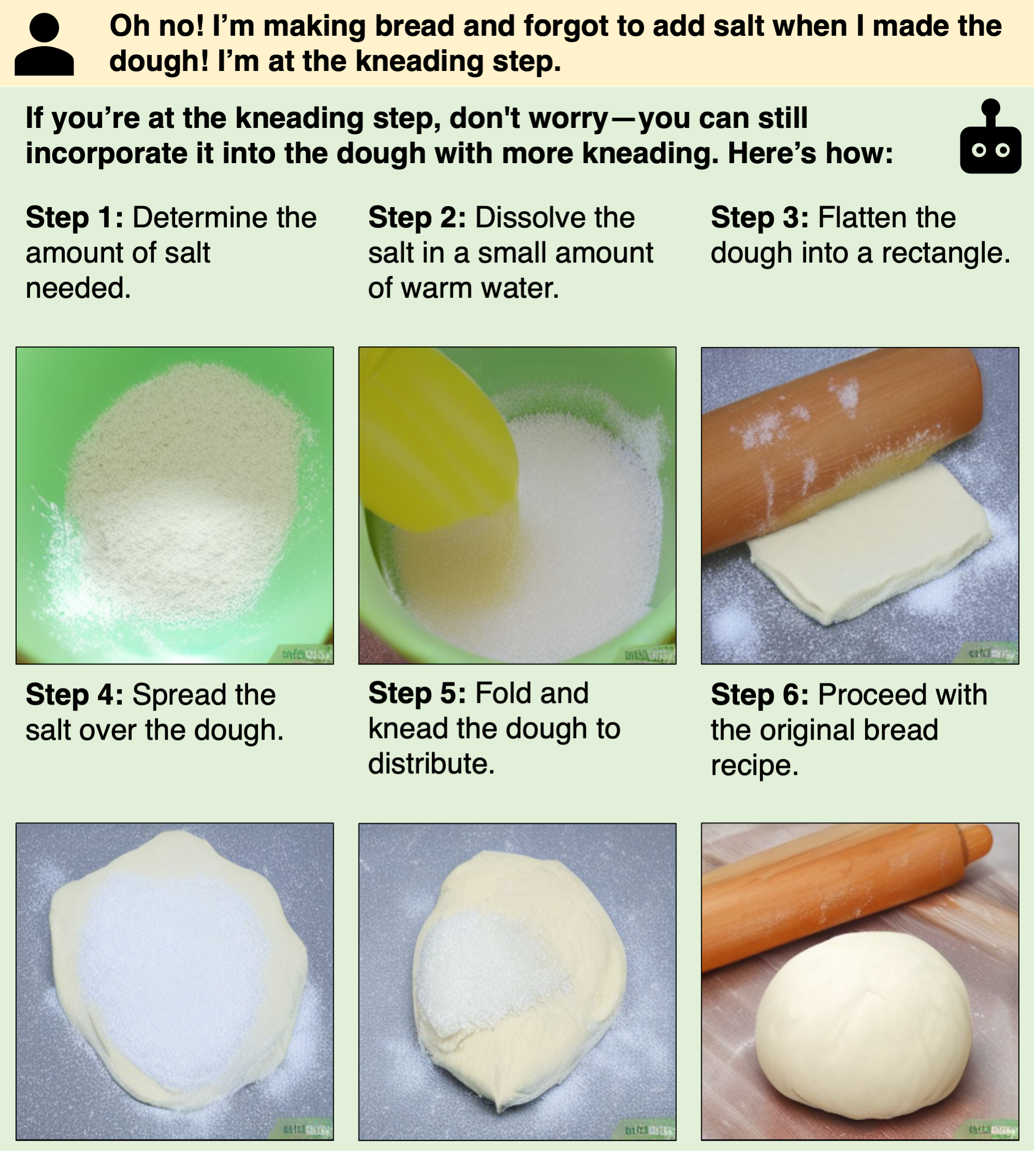
Goal Suggestion
Rather than just illustrating a given goal, StackedDiffusion can suggest a goal matching the user's needs.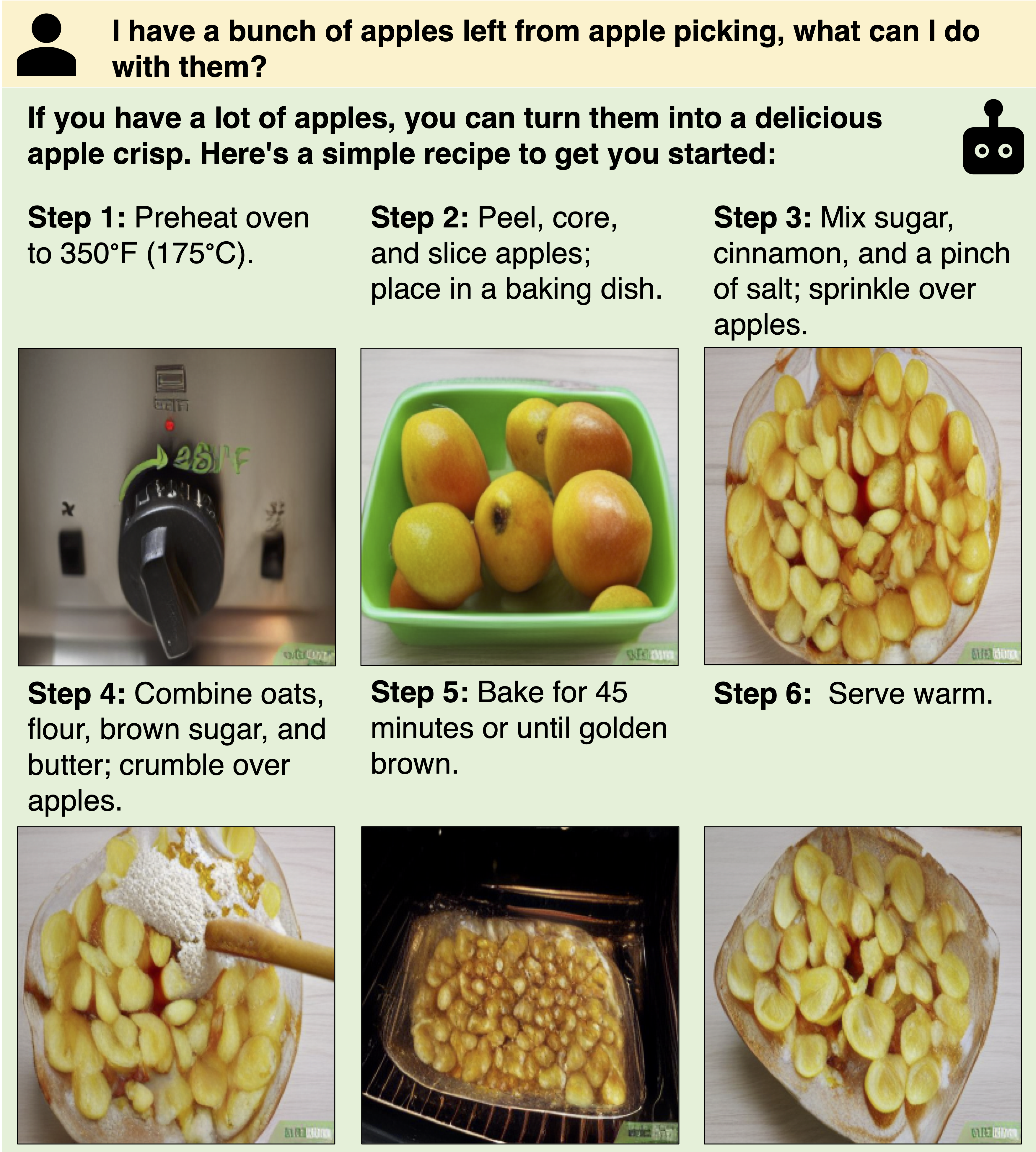
Personalization
One of the most powerful uses of StackedDiffusion is to personalize instructions to a user's circumstances.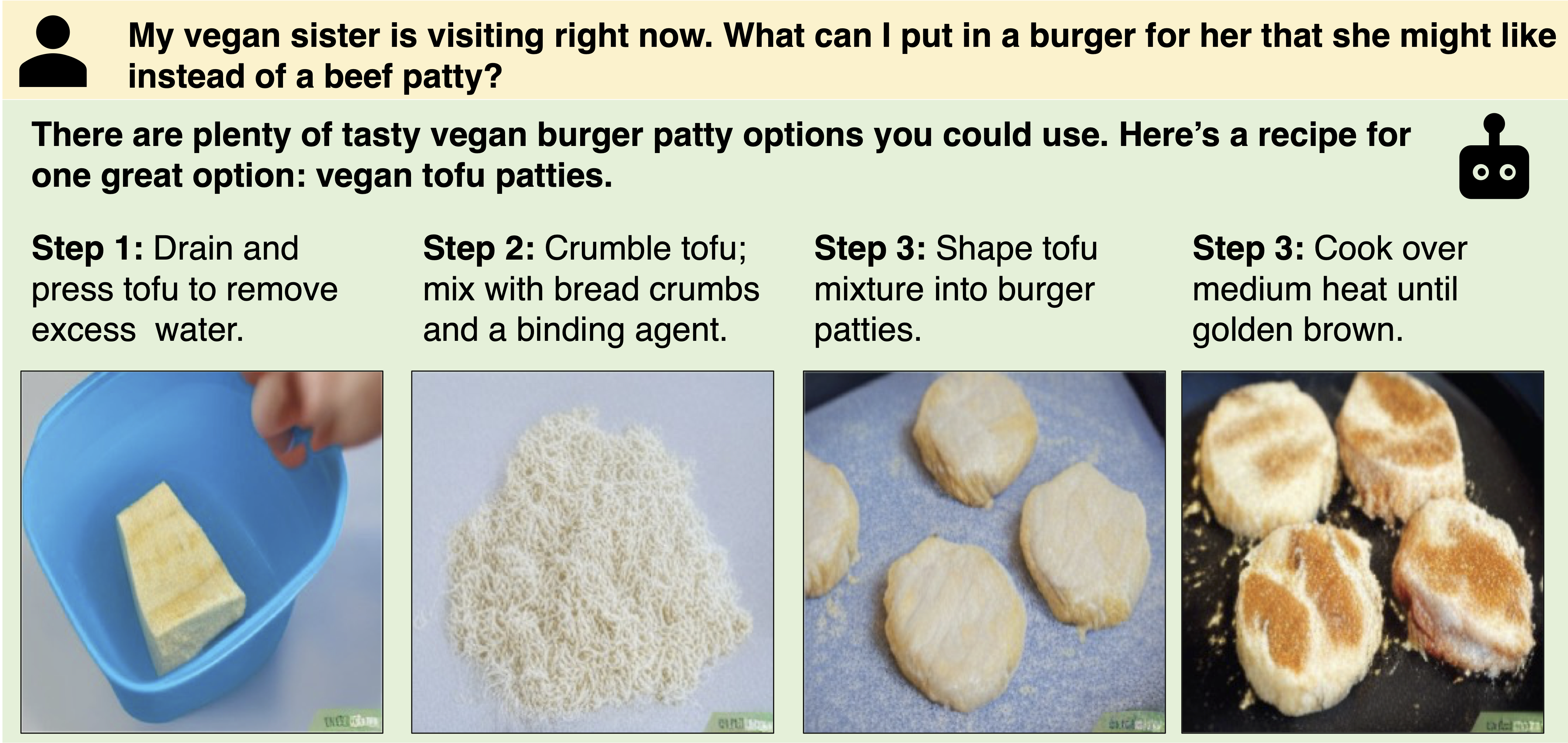
Knowledge Application
The LLM's knowledge enables StackedDiffusion to show the user how to achieve goals they didn't even know to ask about.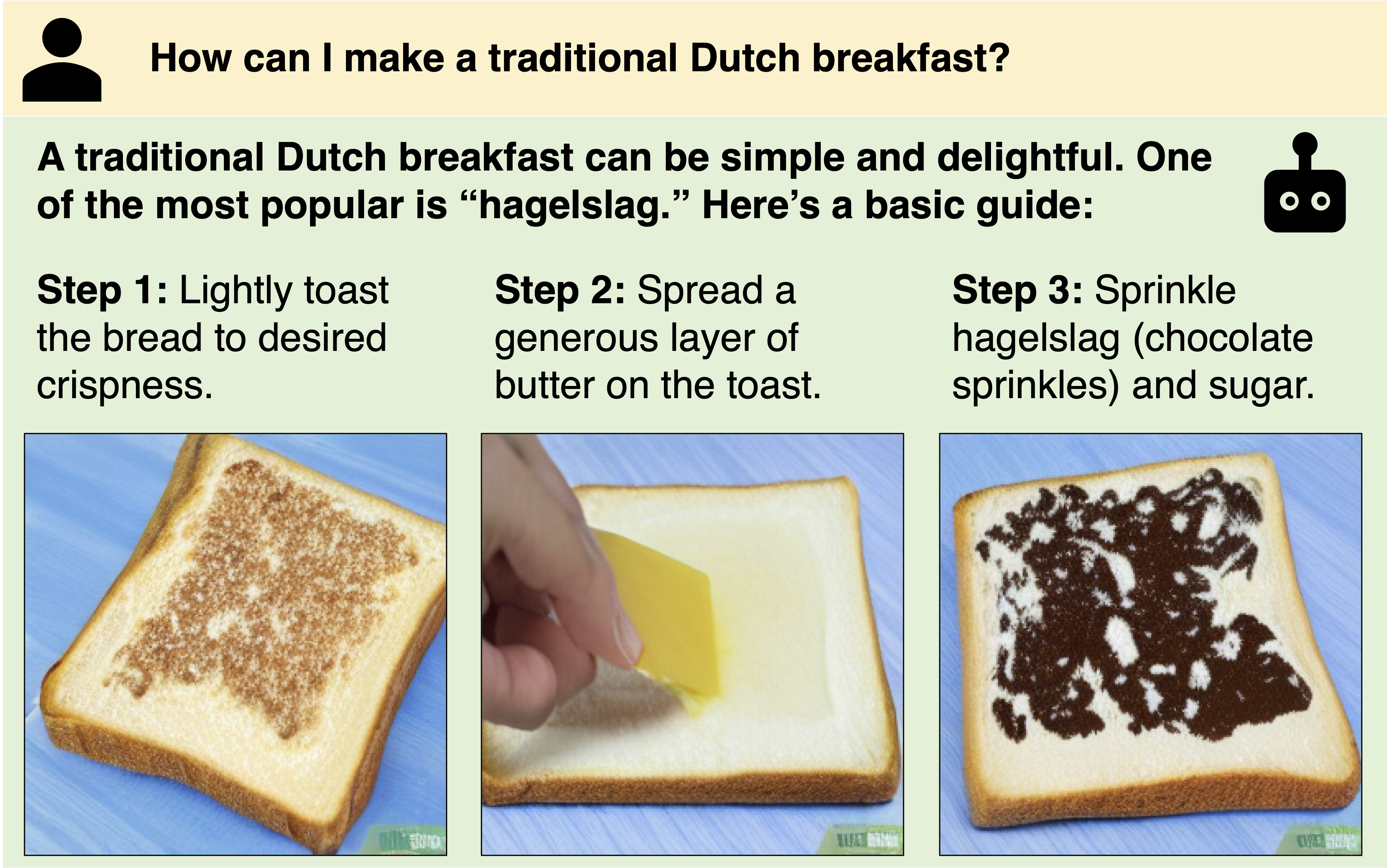