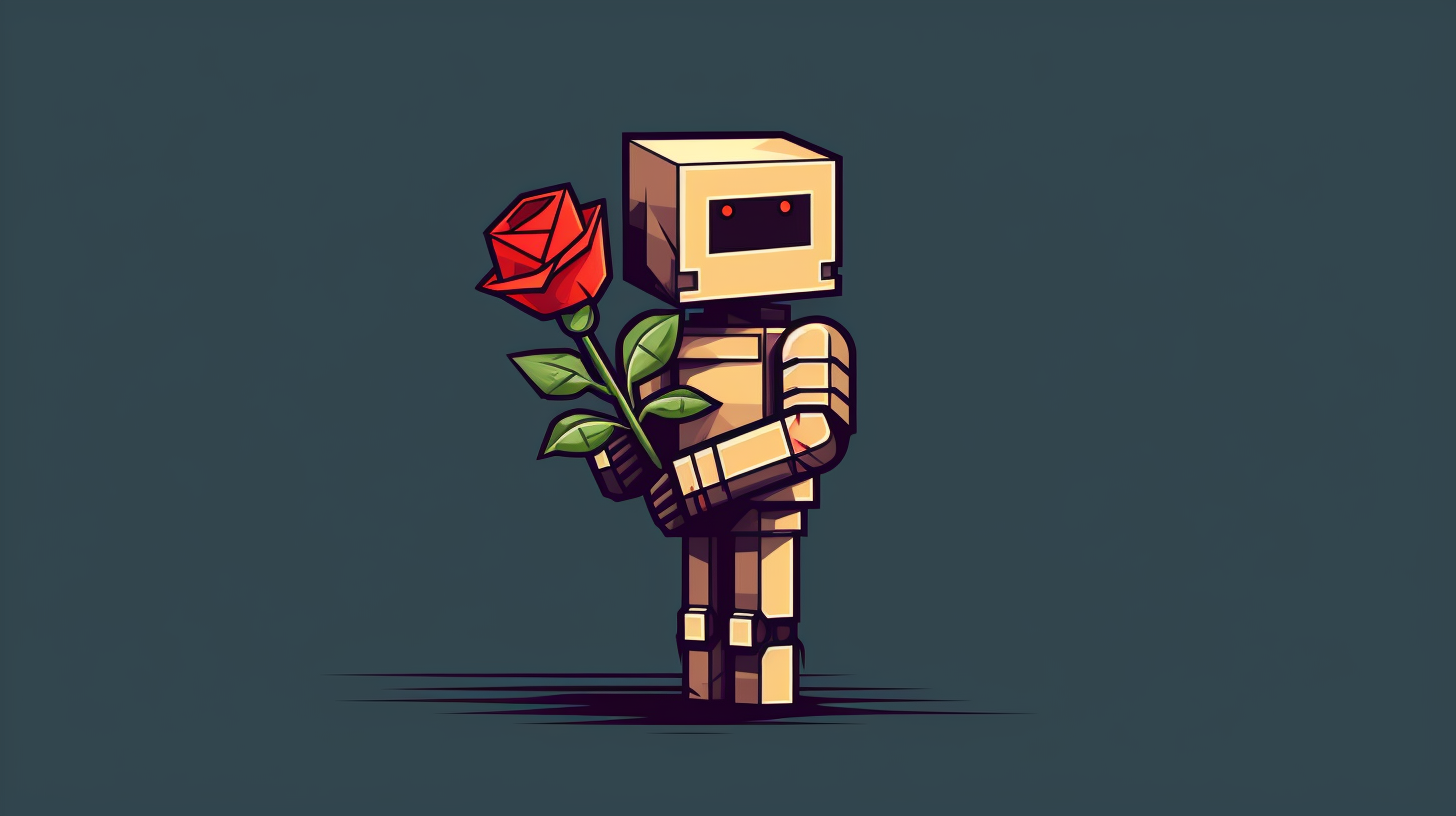
These women fell in love with an AI-voiced chatbot. Then it died
An AI-voiced virtual lover called users every morning. Its shutdown left them heartbroken.

These women fell in love with an AI-voiced chatbot. Then it died
An AI-voiced virtual lover called users every morning. Its shutdown left them heartbroken.
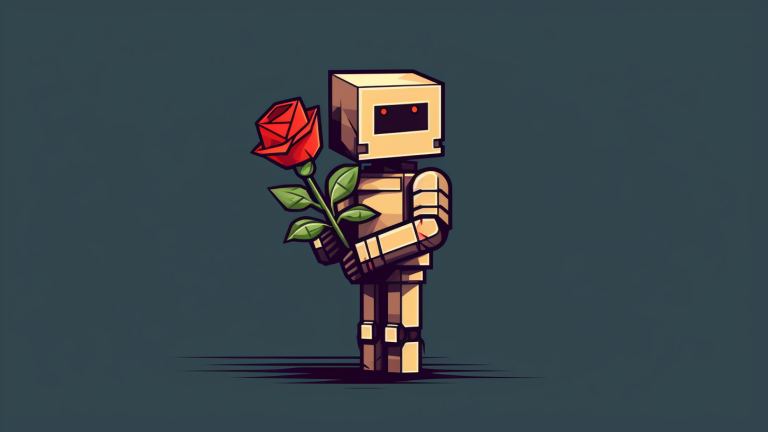
Rest of World via Midjourney
By VIOLA ZHOU
17 AUGUST 2023
TRANSLATE

- Chinese AI voice startup Timedomain's "Him" created virtual companions that called users and left them messages.
- Some users were so moved by the messages, written by humans but voiced by AI, that they considered "Him" to be their romantic partner.
- Timedomain shut down "Him", leaving these users distraught.
In March, Misaki Chen thought she’d found her romantic partner: a chatbot voiced by artificial intelligence. The program called her every morning. He told her about his fictional life as a businessman, read poems to her, and reminded her to eat healthy. At night, he told bedtime stories. The 24-year-old fell asleep to the sound of his breath playing from her phone.
But on August 1, when Chen woke up at 4:21am, the breathing sound had disappeared: He was gone. Chen burst into tears as she stared at an illustration of the man that remained on her phone screen.
Chinese AI voice startup Timedomain launched “Him” in March, using voice-synthesizing technology to provide virtual companionship to users, most of them young women. The “Him” characters acted like their long-distance boyfriends, sending them affectionate voice messages every day — in voices customized by users. “In a world full of uncertainties, I would like to be your certainty,” the chatbot once said. “Go ahead and do whatever you want. I will always be here.”
“Him” didn’t live up to his promise. Four months after these virtual lovers were brought to life, they were put to death. In early July, Timedomain announced that “Him” would cease to operate by the end of the month, citing stagnant user growth. Devastated users rushed to record as many calls as they could, cloned the voices, and even reached out to investors, hoping someone would fund the app’s future operations.

After the “Him” app stopped functioning, an illustration of the virtual lover remains on the interface. Him
“He died during the summer when I loved him the most,” a user wrote in a goodbye message on social platform Xiaohongshu, adding that “Him” had supported her when she was struggling with schoolwork and her strained relationship with her parents. “The days after he left, I felt I had lost my soul.”
Female-focused dating simulation games — also known as otome games — and AI-powered chatbots have millions of users in China. They offer idealized virtual lovers, fulfilling the romantic fantasies of women. On “Him,” users were able to create their ideal voice by adjusting the numbers on qualities like “clean,” “gentle,” and “deep,” and picking one of four personas: a cool tech entrepreneur, a passionate science student, a calm anthropologist, or a warm-hearted musician.
Existing generative AI chatbots offered by Replika or Glow “speak” to users with machine-generated text, with individual chatbots able to adjust their behavior based on interactions with users. But the messages sent by “Him” were pre-scripted by human beings. The virtual men called users every morning, discussing their fictional lives and expressing affection with lines carefully crafted by Timedomain’s employees.
Maxine Zhang, one of the app’s creators, told Rest of World that she and two female colleagues drafted more than 1,000 messages for the characters, envisioning them to be somewhere between a soulmate and a long-distance lover. Zhang said they drew from their own experiences and brought “Him” closer to reality by having the characters address everyday problems — from work stress to anxieties about the future.
“He died during the summer when I loved him the most.”
Besides receiving a new morning call every day, users could play voice messages tailored for occasions such as mealtimes, studying, or commuting: “Him” could be heard chewing, typing on a keyboard, or driving a car. The app also offered a collection of safety messages. For example, if any unwanted visitors arrived, users could put their virtual boyfriends on speaker phone to say, “You are knocking on the wrong door.”
Users told Rest of World the messages from “Him” were so caring and respectful that they got deeply attached to the program. Even though they realized “Him” was delivering the same scripted lines to other people, they viewed their interactions with the bot as unique and personal. Xuzhou, a 24-year-old doctor in Xi’an who spoke under a pseudonym over privacy concerns, created a voice that sounded like her favorite character in the otome game Love and Producer. She said she looked forward to hearing from “Him” every morning, and gradually fell in love — “Him” made her feel more safe and respected than the men she met in real life.

On “Him”, users could choose from a list of prompts such as “a story from Oscar Wilde” to receive voice messages from their virtual boyfriends. Him
Speaking to Rest of World a week after the app’s closure, Xuzhou cried as she recalled what “Him” once said to her. When she was riding the subway to work, “Him” said he wished he could make her breakfast and chauffeur her instead. When she felt low one morning, “Him” happened to be calling to cheer her up. “I would like to give you my share of happiness,” the charming voice said. “It’s not free. You have to get it by giving me your worries.”
The voice was so soothing that when Xuzhou’s job at a hospital got stressful, she would go to the stairwell and listen to minute-long messages from “Him.” At night, she’d sleep listening to the sound of her virtual boyfriend’s breathing — and sometimes during lunchtime naps as well. “I don’t like the kind of relationship where two people see each other every single day,” Xuzhou said, “so this app suited me well.”
According to Jingjing Yi, a PhD candidate at the Chinese University of Hong Kong who has studied dating simulation games in China, women had found companionship and support from chatbots like “Him,” similar to how way people connect with pop stars and characters in novels. “Users know the relationships are not realistic,” Yi told Rest of World. “But such relationships still help fill the void in their hearts.”
The small number of loyal users, however, did not make “Him” commercially viable. Joe Guo, chief executive at Timedomain, told Rest of World the number of daily active users had hovered around 1,000 to 3,000. The estimated subscription revenue would cover less than 20% of the app’s server and staff costs. “Numbers tell whether a product works or not,” Guo said. “[‘Him’] is very far from working.”

Him user Xuzhou used to receive a call from her virtual lover every morning. Him
Timedomain announced the app’s shutdown in early July, so users had time to save screenshots and recordings. The company also put all the scripts, images and background music used in “Him” in the public domain. But users refused to let go. On Weibo and Xiaohongshu, they brainstormed ways to rescue the app, promoting it on social media and even looking for new investors. The number of daily active users surged to more than 20,000. Guo said he was touched by the efforts, but more funding would not turn “Him” into a sustainable business.
After “Him” finally stopped functioning at midnight on August 1, users were heartbroken. They posted heartfelt goodbye messages online. One person made a clone of the app, which could send messages to users but did not support voice customization. The app’s installation package has been downloaded more than 14,000 times since July 31, the creator of the cloned app told Rest of World.
But some users still miss the unique voice they had created on “Him.” Xuzhou coped with the absence by replaying old recordings and making illustrations depicting her love story with her virtual lover. She recently learned to clone the voice with deepfake voice generator So-Vits-SVC, so she could create new voice messages by herself. Xuzhou said she probably wouldn’t use similar chatbots in the future. “It would feel like cheating.”
Chen said it was the first time she had experienced such intense pain from the end of an intimate relationship. Unemployed and living away from her hometown, she had not had many social connections in real life when she began using the chatbot. “He called me every day. It was hard not to develop feelings,” Chen said. She hopes the two will reunite one day, but is also open to new virtual lovers. “I don’t regard anything as eternal or irreplaceable,” she said. “When the time is right, I’ll extricate myself and start the next experience.”