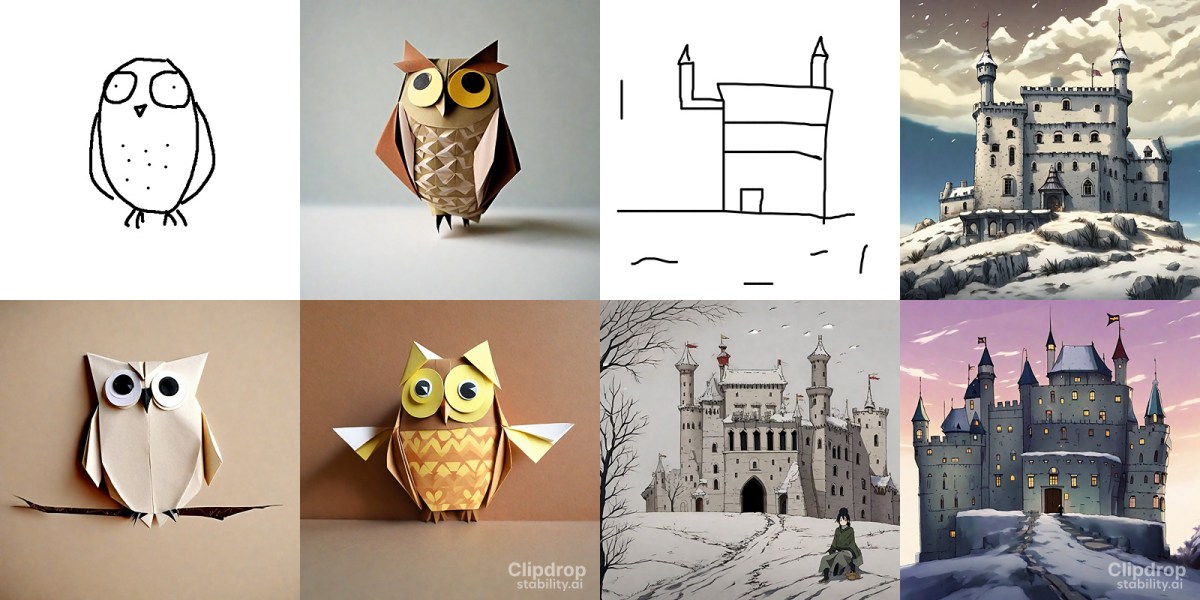
Stability AI releases Stable Doodle, a sketch-to-image tool | TechCrunch
Stability has released a new tool through Clipdrop, the AI art platform it acquired recently, that turns sketches into artwork.

Stability AI releases Stable Doodle, a sketch-to-image tool
Kyle Wiggers@kyle_l_wiggers / 8:00 AM EDT•July 13, 2023Comment
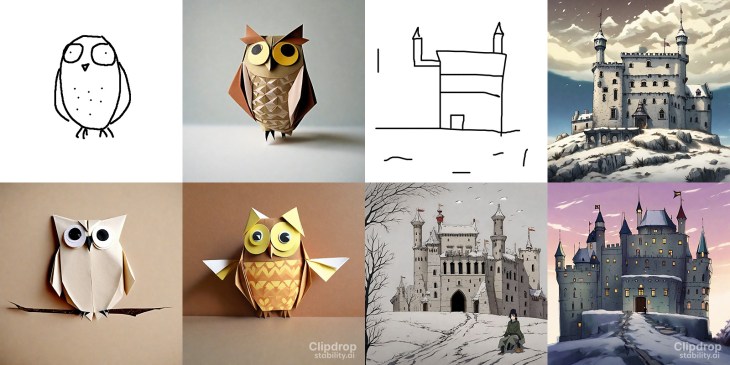
Image Credits: Stability AI
Stability AI, the startup behind the image-generating model Stable Diffusion, is launching a new service that turns sketches into images.
The sketch-to-image service, Stable Doodle, leverages the latest Stable Diffusion model to analyze the outline of a sketch and generate a “visually pleasing” artistic rendition of it. It’s available starting today through ClipDrop, a platform Stability acquired in March through its purchase of Init ML, an AI startup founded by ex-Googlers,
“Stable Doodle is geared toward both professionals and novices, regardless of their familiarity with AI tools,” Stability AI writes in a blog post shared with TechCrunch via email. “With Stable Doodle, anyone with basic drawing skills and online access can generate high-quality original images in seconds.”
There’s plenty of sketch-to-image AI tools out there, including open source projects and ad-supported apps. But Stable Doodle is unique in that it allows for more “precise” control over the image generation, Stability AI contends.
Under the hood, powering Stable Doodle is a Stable Diffusion model — Stable Diffusion XL — paired with a “conditional control solution” developed by one of Tencent’s R&D divisions, the Applied Research Center (ARC). Called T2I-Adapter, the control solution both allows Stable Diffusion XL to accept sketches as input and guides the model to enable better fine-tuning of the output artwork.

Image Credits: Stability AI
“T2I-Adapter enable Stable Doodle to understand the outlines of sketches and generate images based on prompts combined with the outlines defined by the model,” Stability AI explains in the blog post.
This writer didn’t have the opportunity to test Stable Doodle prior to its release. But the cherry-picked images Stability AI sent me looked quite good, at least in comparison to the doodle that inspired them.
In addition to a sketch, Stable Doodle accepts a prompt to guide the image generation process, such as “A comfy chair, ‘isometric’ style” or “Cat with a jeans jacket, ‘digital art’ style.” There’s a limit to the customization, though — at launch, Stable Doodle only supports 14 styles of art.
Stability AI envisions Stable Doodle serving as a tool for designers, illustrators and other professionals to “free up valuable time” and “maximize efficiency” in their work. At the same time, the company cautions that the quality of output images is dependent on the detail of the initial drawing and the descriptiveness of the prompt, as well as the complexity of the scene being depicted.
“Ideas drawn as sketches can be immediately implemented into works to create designs for clients, material for presentation decks and websites or even create logos,” the company proposes. “Moving forward, Stable Doodle will enable users to import a sketch. Further, we will include use cases for specific verticals, including real estate applications, for example.”
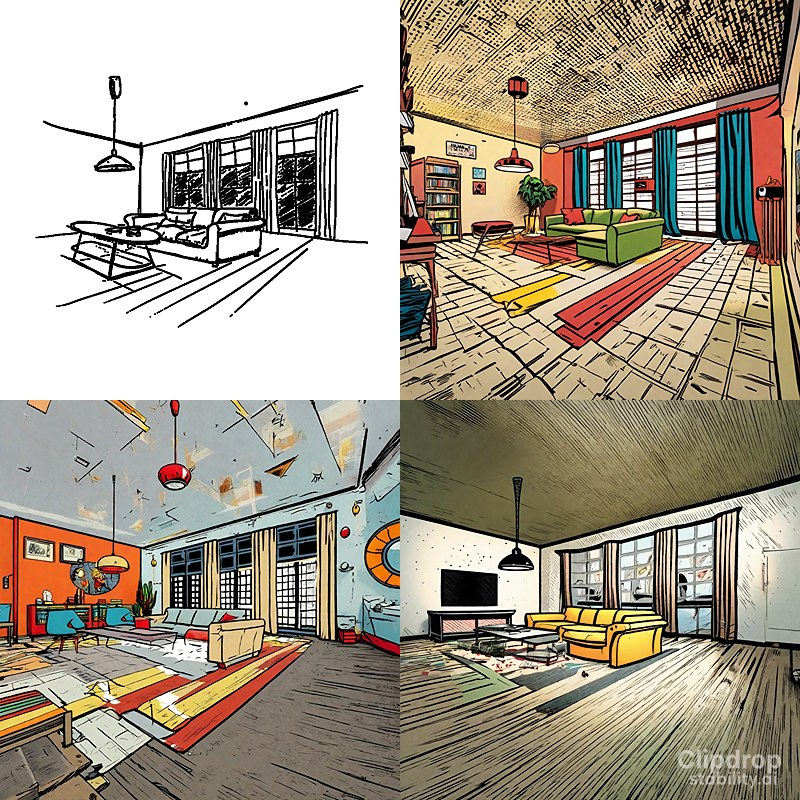
Image Credits: Stability AI
With tools like Stable Doodle, Stability AI is chasing after new sources of revenue following a lull in its commercial endeavors. (Stable Doodle is free, but subject to limits.) In April, Semafor reported that Stability AI was burning through cash, leading to an executive hunt to help ramp up sales.
Last month, Stability AI raised $25 million through a convertible note (i.e. debt that converts to equity), bringing its total raised to over $125 million. But it hasn’t closed new funding at a higher valuation. The startup was last valued at $1 billion; reportedly, Stability was seeking to quadruple that within the next few months.
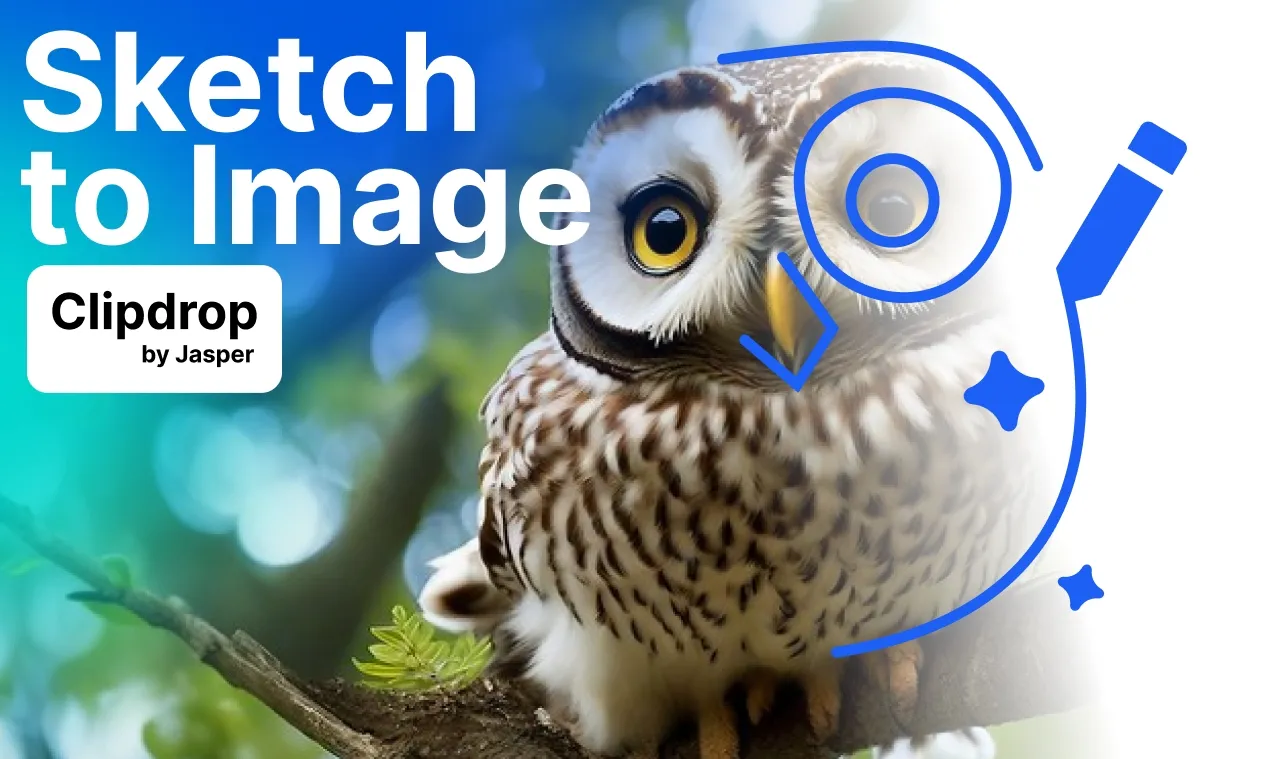
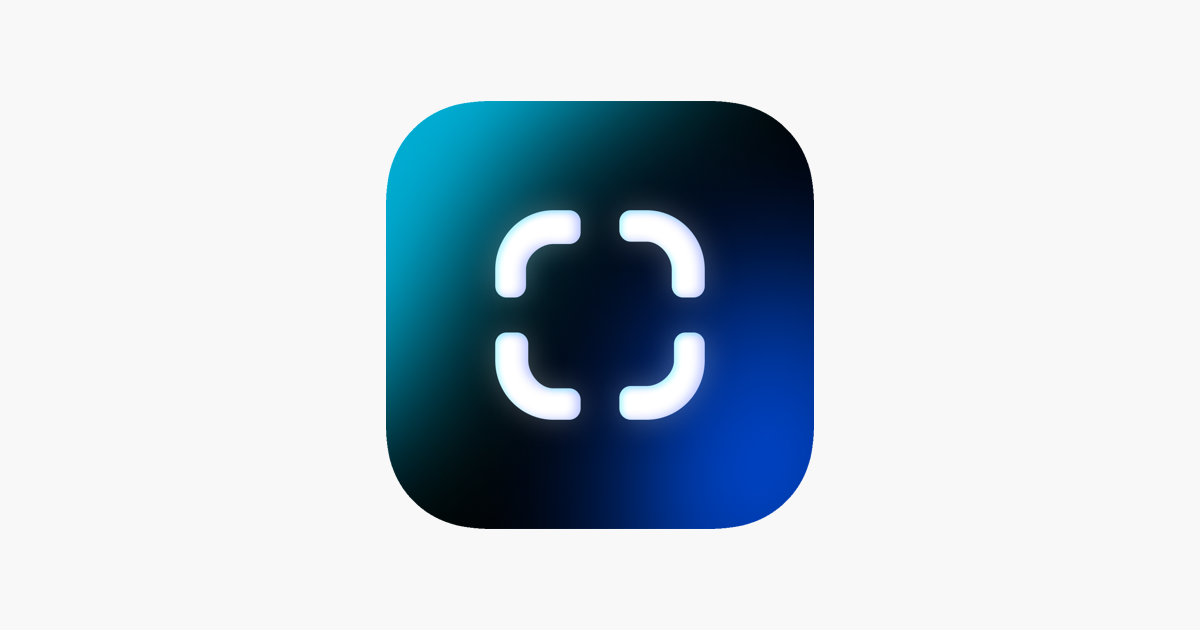
ClipDrop - Cleanup Pictures
Clipdrop is an app suite allowing you to easily modify your images with AI. - Incredibly accurate Background Removal - Remove objects, text, defects, or people from pictures - Relight your photos & drawings in seconds - Teleport anything, anywhere with AI - Generate images from text - Create...
apps.apple.com
Last edited: