1/1
[CV] Understanding Alignment in Multimodal LLMs: A Comprehensive Study
[2407.02477] Understanding Alignment in Multimodal LLMs: A Comprehensive Study
- This paper examines alignment strategies for Multimodal Large Language Models (MLLMs) to reduce hallucinations and improve visual grounding. It categorizes alignment methods into offline (e.g. DPO) and online (e.g. Online-DPO).
- The paper reviews recently published multimodal preference datasets like POVID, RLHF-V, VLFeedback and analyzes their components: prompts, chosen responses, rejected responses.
- It introduces a new preference data sampling method called Bias-Driven Hallucination Sampling (BDHS) which restricts image access to induce language model bias and trigger hallucinations.
- Experiments align the LLaVA 1.6 model and compare offline, online and mixed DPO strategies. Results show combining offline and online can yield benefits.
- The proposed BDHS method achieves strong performance without external annotators or preference data, just using self-supervised data.
To post tweets in this format, more info here: https://www.thecoli.com/threads/tips-and-tricks-for-posting-the-coli-megathread.984734/post-52211196
[CV] Understanding Alignment in Multimodal LLMs: A Comprehensive Study
[2407.02477] Understanding Alignment in Multimodal LLMs: A Comprehensive Study
- This paper examines alignment strategies for Multimodal Large Language Models (MLLMs) to reduce hallucinations and improve visual grounding. It categorizes alignment methods into offline (e.g. DPO) and online (e.g. Online-DPO).
- The paper reviews recently published multimodal preference datasets like POVID, RLHF-V, VLFeedback and analyzes their components: prompts, chosen responses, rejected responses.
- It introduces a new preference data sampling method called Bias-Driven Hallucination Sampling (BDHS) which restricts image access to induce language model bias and trigger hallucinations.
- Experiments align the LLaVA 1.6 model and compare offline, online and mixed DPO strategies. Results show combining offline and online can yield benefits.
- The proposed BDHS method achieves strong performance without external annotators or preference data, just using self-supervised data.
To post tweets in this format, more info here: https://www.thecoli.com/threads/tips-and-tricks-for-posting-the-coli-megathread.984734/post-52211196
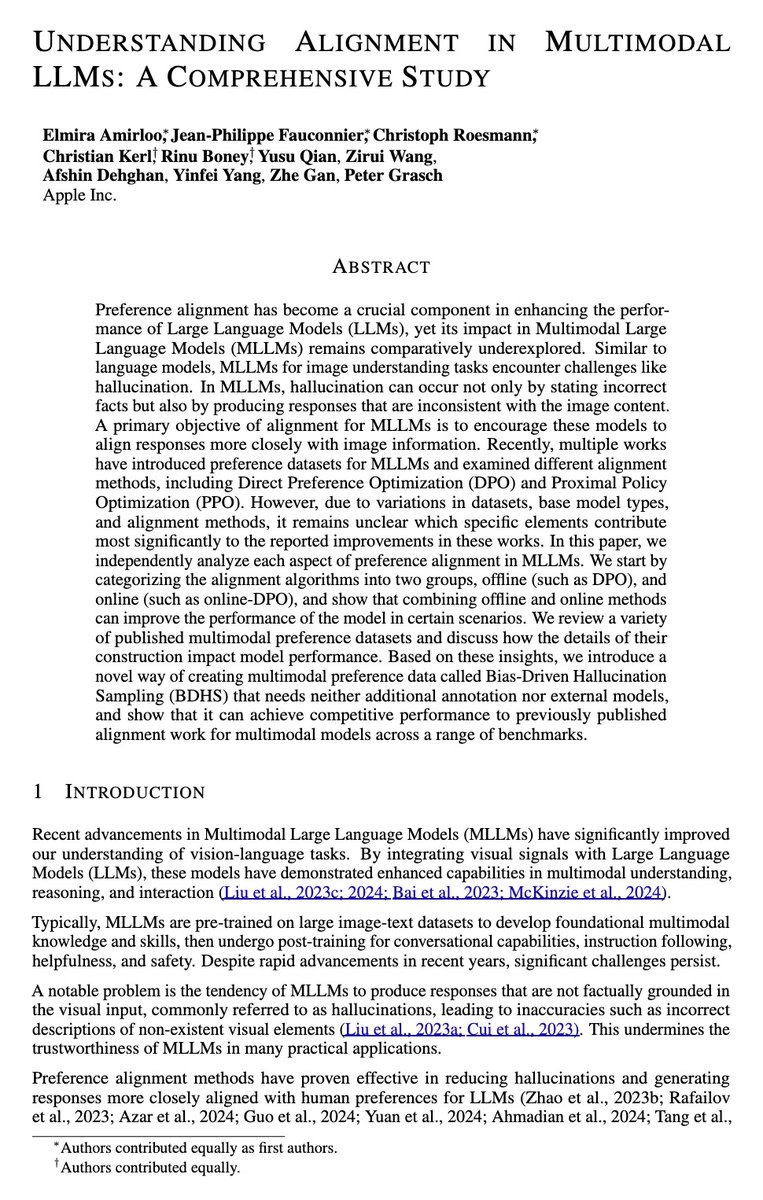
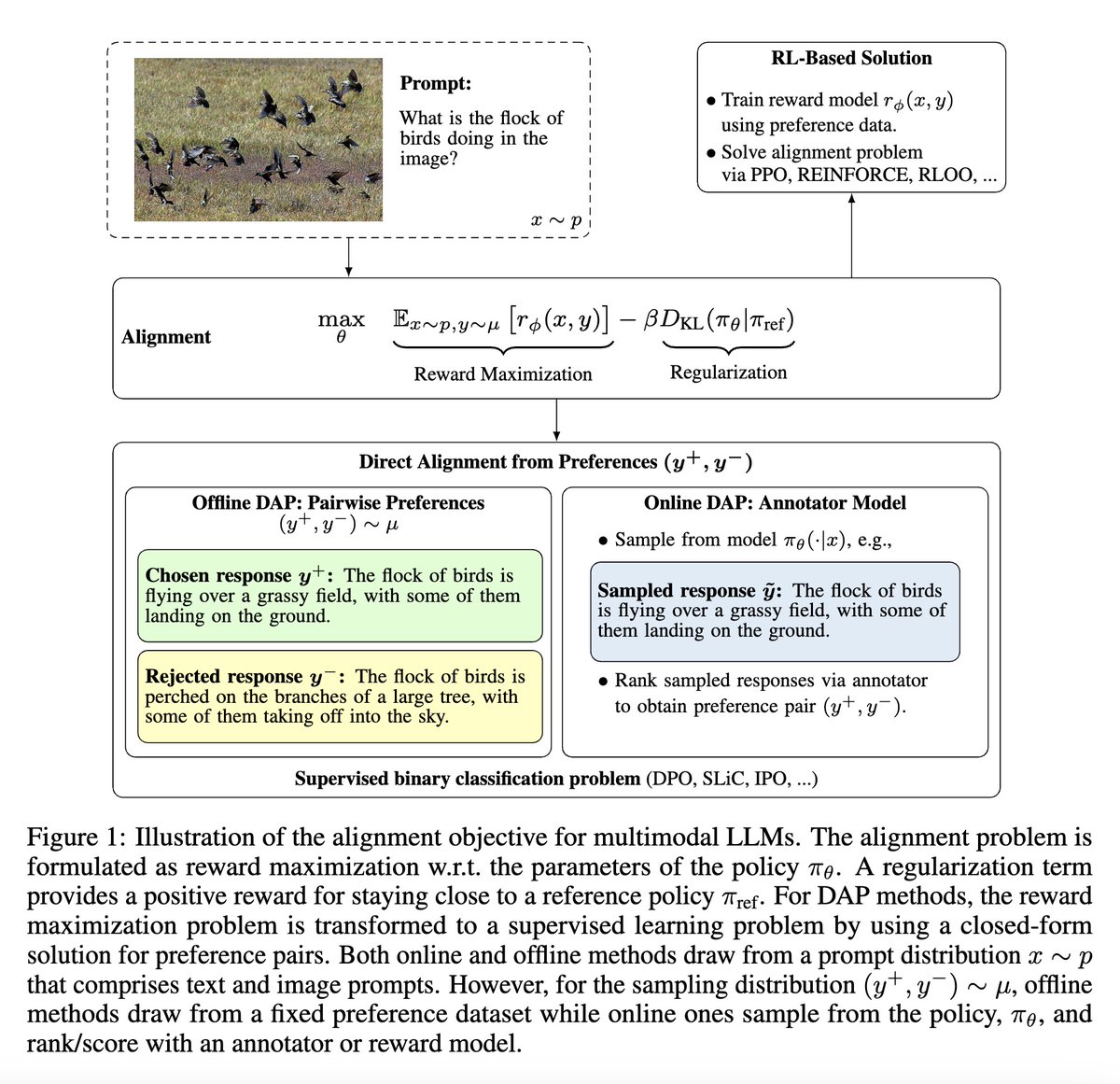
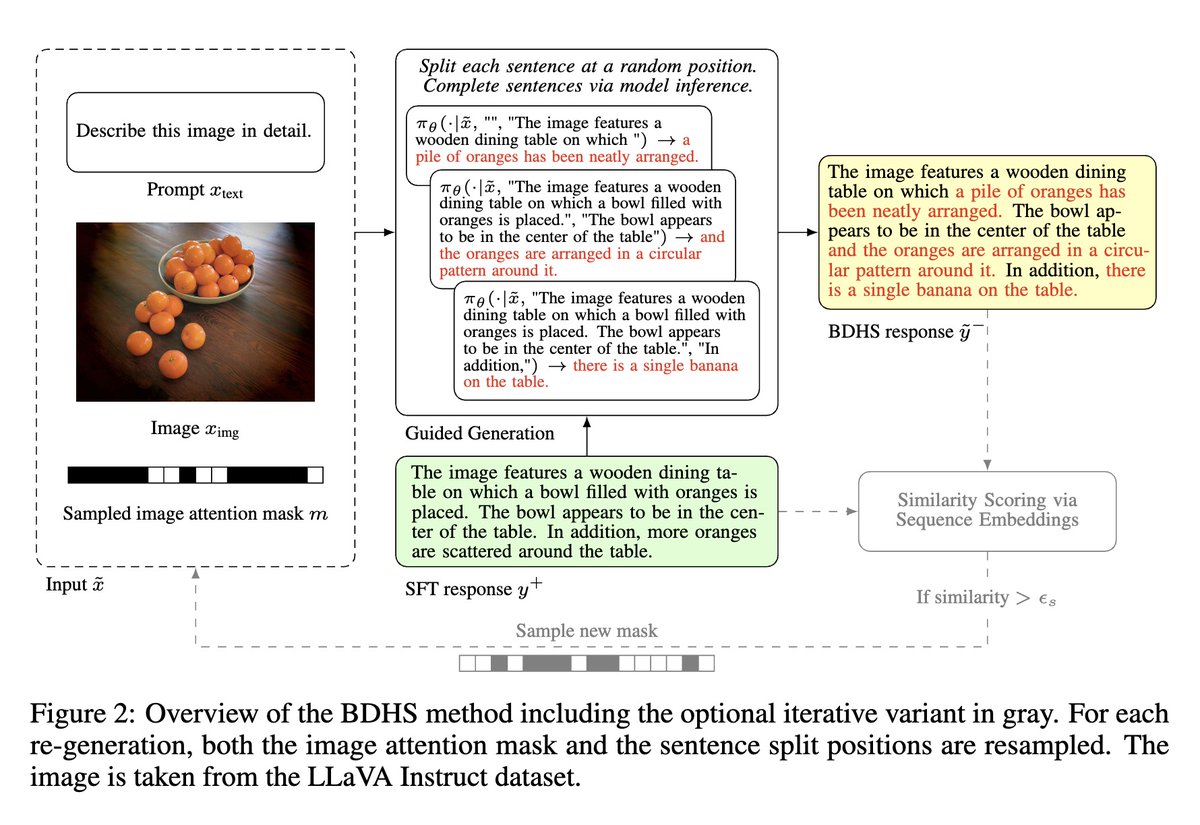