1/8
@DKThomp
Fascinating paper (and post!) on AI in medicine
- cardiologists working with AI said the machine's diagnosis, triage and management were equal or better than human cardiologists in most areas
- the paper's summary comes with a generative AI podcast explaining the results
[Quoted tweet]
One of the most promising areas for the application of AI in medicine is scaling specialty expertise. There simply aren't enough specialist doctors to care for everyone in need. We believe AI can help.
As a first step towards that goal, we worked with the amazing Google medical AI team to tune and test their conversational agent AMIE in the setting of Stanford's Center for Inherited Cardiovascular Disease.
Unlike many medical studies of LLMs, we completed our testing not with curated cases or exam questions but real-world medical data presented in exactly the way we receive it in clinic.
Data was in the form of reports derived from multi-modal data sources including medical records, ecgs, stress tests, imaging tests, and genomic data. AMIE was augmented by web search and self-critique capabilities and used chain-of-reasoning strategies fine-tuned on data from just 9 typical patients.
What did we find?
1. Overall, AMIE responses on diagnosis, triage and management were rated by specialty cardiologists as equivalent to or better than those of general cardiologists across 10 domains.
2. Access to AMIE's responses improved the general cardiologists' responses in almost two thirds of cases.
3. Qualitative data suggested that the AI and human approaches were highly complementary with AMIE judged thorough and sensitive and general cardiologists judged concise and specific.
In conclusion, our data suggest that LLMs such as AMIE could usefully democratize subspecialty medical expertise augmenting general cardiologists' assessments of inherited cardiovascular disease patients.
Paper: arxiv.org/abs/2410.03741
Generative podcast describing the paper (!): shorturl.at/rdKZn
Stanford Center for Inherited Cardiovascular Disease: med.stanford.edu/familyheart
AMIE: arxiv.org/abs/2401.05654
Congrats to @DrJackOSullivan and @taotu831 for leading the charge on this work as well as the @StanfordDeptMed team and the amazing folks @Google led by @alan_karthi and @vivnat
2/8
@Jill992004231
AI is coming at us very, very quickly.
3/8
@ian_sportsdev
So? Tech is easy, politics is hard
4/8
@fl_saloni
Cardiologists are the gatekeepers for adoption of this AI and most (NOT all) will create mistrust in AI to preserve their status and comp. How do you overcome this
5/8
@Spear_Owl
AI+DR>DR
6/8
@mario_anchor
The question is will specialty caregivers allow this. Their lobbying apparatus has already created an artificial doctor shortage to prop up wages.
7/8
@EscoboomVanilla
Also this!
Wimbledon staff left devastated after decision to break 147-year tradition and put 300 jobs at risk
8/8
@PatrickPatten8
I think medical and education is where AI will have the biggest impact. Hopefully America rethinks what an educated population looks like, cause right now 47% think fascism is a good idea... hopefully we can do better.
To post tweets in this format, more info here: https://www.thecoli.com/threads/tips-and-tricks-for-posting-the-coli-megathread.984734/post-52211196
@DKThomp
Fascinating paper (and post!) on AI in medicine
- cardiologists working with AI said the machine's diagnosis, triage and management were equal or better than human cardiologists in most areas
- the paper's summary comes with a generative AI podcast explaining the results
[Quoted tweet]
One of the most promising areas for the application of AI in medicine is scaling specialty expertise. There simply aren't enough specialist doctors to care for everyone in need. We believe AI can help.
As a first step towards that goal, we worked with the amazing Google medical AI team to tune and test their conversational agent AMIE in the setting of Stanford's Center for Inherited Cardiovascular Disease.
Unlike many medical studies of LLMs, we completed our testing not with curated cases or exam questions but real-world medical data presented in exactly the way we receive it in clinic.
Data was in the form of reports derived from multi-modal data sources including medical records, ecgs, stress tests, imaging tests, and genomic data. AMIE was augmented by web search and self-critique capabilities and used chain-of-reasoning strategies fine-tuned on data from just 9 typical patients.
What did we find?
1. Overall, AMIE responses on diagnosis, triage and management were rated by specialty cardiologists as equivalent to or better than those of general cardiologists across 10 domains.
2. Access to AMIE's responses improved the general cardiologists' responses in almost two thirds of cases.
3. Qualitative data suggested that the AI and human approaches were highly complementary with AMIE judged thorough and sensitive and general cardiologists judged concise and specific.
In conclusion, our data suggest that LLMs such as AMIE could usefully democratize subspecialty medical expertise augmenting general cardiologists' assessments of inherited cardiovascular disease patients.
Paper: arxiv.org/abs/2410.03741
Generative podcast describing the paper (!): shorturl.at/rdKZn
Stanford Center for Inherited Cardiovascular Disease: med.stanford.edu/familyheart
AMIE: arxiv.org/abs/2401.05654
Congrats to @DrJackOSullivan and @taotu831 for leading the charge on this work as well as the @StanfordDeptMed team and the amazing folks @Google led by @alan_karthi and @vivnat
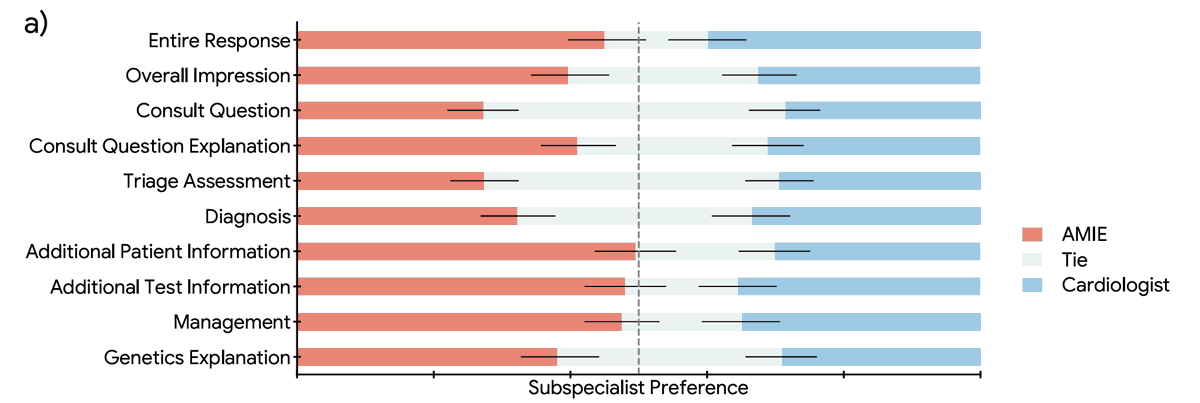
2/8
@Jill992004231
AI is coming at us very, very quickly.
3/8
@ian_sportsdev
So? Tech is easy, politics is hard

4/8
@fl_saloni
Cardiologists are the gatekeepers for adoption of this AI and most (NOT all) will create mistrust in AI to preserve their status and comp. How do you overcome this
5/8
@Spear_Owl
AI+DR>DR
6/8
@mario_anchor
The question is will specialty caregivers allow this. Their lobbying apparatus has already created an artificial doctor shortage to prop up wages.
7/8
@EscoboomVanilla
Also this!
Wimbledon staff left devastated after decision to break 147-year tradition and put 300 jobs at risk
8/8
@PatrickPatten8
I think medical and education is where AI will have the biggest impact. Hopefully America rethinks what an educated population looks like, cause right now 47% think fascism is a good idea... hopefully we can do better.
To post tweets in this format, more info here: https://www.thecoli.com/threads/tips-and-tricks-for-posting-the-coli-megathread.984734/post-52211196