May 6, 2024
Editor’s Note: This news was originally shared by Stack Overflow here(opens in a new window).
OpenAI and Stack Overflow are coming together via OverflowAPI access to provide OpenAI users and customers with the accurate and vetted data foundation that AI tools need to quickly find a solution to a problem so that technologists can stay focused on priority tasks. OpenAI will also surface validated technical knowledge from Stack Overflow directly in ChatGPT, giving users easy access to trusted, attributed, accurate, and highly technical knowledge and code backed by the millions of developers that have contributed to the Stack Overflow platform for 15 years.
As part of this collaboration:
“Learning from as many languages, cultures, subjects, and industries as possible ensures that our models can serve everyone. The developer community is particularly important to both of us. Our deep partnership with Stack Overflow will help us enhance the user and developer experience on both our platforms,” said Brad Lightcap, COO at OpenAI.
“Stack Overflow is the world’s largest developer community, with more than 59 million questions and answers. Through this industry-leading partnership with OpenAI, we strive to redefine the developer experience, fostering efficiency and collaboration through the power of community, best-in-class data, and AI experiences,” said Prashanth Chandrasekar, CEO of Stack Overflow. “Our goal with OverflowAPI, and our work to advance the era of socially responsible AI, is to set new standards with vetted, trusted, and accurate data that will be the foundation on which technology solutions are built and delivered to our user.”
The first set of new integrations and capabilities between Stack Overflow and OpenAI will be available in the first half of 2024. Beyond this, OpenAI’s partnership with Stack Overflow will enable Stack Overflow to continue to reinvest in community-driven features(opens in a new window). To learn more about Stack Overflow’s API solution and partnerships, visit https://stackoverflow.co/api-solutions/
API Partnership with Stack Overflow
Stack Overflow and OpenAI today announced a new API partnership that will empower developers with the collective strengths of the world’s leading knowledge platform for highly technical content with the world’s most popular LLM models for AI development.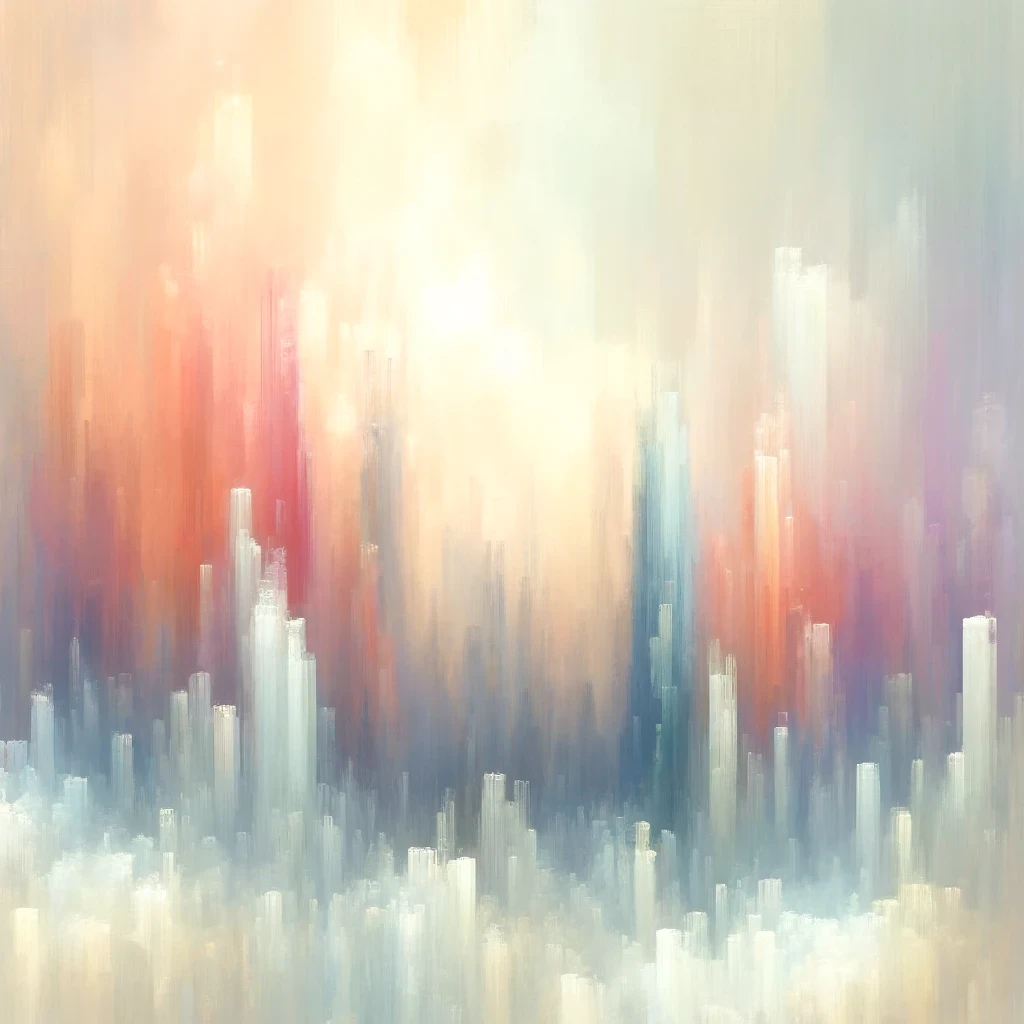
Editor’s Note: This news was originally shared by Stack Overflow here(opens in a new window).
OpenAI and Stack Overflow are coming together via OverflowAPI access to provide OpenAI users and customers with the accurate and vetted data foundation that AI tools need to quickly find a solution to a problem so that technologists can stay focused on priority tasks. OpenAI will also surface validated technical knowledge from Stack Overflow directly in ChatGPT, giving users easy access to trusted, attributed, accurate, and highly technical knowledge and code backed by the millions of developers that have contributed to the Stack Overflow platform for 15 years.
As part of this collaboration:
- OpenAI will utilize Stack Overflow’s OverflowAPI product and collaborate with Stack Overflow to improve model performance for developers who use their products. This integration will help OpenAI improve its AI models using enhanced content and feedback from the Stack Overflow community and provide attribution to the Stack Overflow community within ChatGPT to foster deeper engagement with content.
- Stack Overflow will utilize OpenAI models as part of their development of OverflowAI and work with OpenAI to leverage insights from internal testing to maximize the performance of OpenAI models. OpenAI’s partnership with Stack Overflow will help further drive its mission to empower the world to develop technology through collective knowledge, as Stack Overflow will be able to create better products that benefit the Stack Exchange community’s health, growth, and engagement.
“Learning from as many languages, cultures, subjects, and industries as possible ensures that our models can serve everyone. The developer community is particularly important to both of us. Our deep partnership with Stack Overflow will help us enhance the user and developer experience on both our platforms,” said Brad Lightcap, COO at OpenAI.
“Stack Overflow is the world’s largest developer community, with more than 59 million questions and answers. Through this industry-leading partnership with OpenAI, we strive to redefine the developer experience, fostering efficiency and collaboration through the power of community, best-in-class data, and AI experiences,” said Prashanth Chandrasekar, CEO of Stack Overflow. “Our goal with OverflowAPI, and our work to advance the era of socially responsible AI, is to set new standards with vetted, trusted, and accurate data that will be the foundation on which technology solutions are built and delivered to our user.”
The first set of new integrations and capabilities between Stack Overflow and OpenAI will be available in the first half of 2024. Beyond this, OpenAI’s partnership with Stack Overflow will enable Stack Overflow to continue to reinvest in community-driven features(opens in a new window). To learn more about Stack Overflow’s API solution and partnerships, visit https://stackoverflow.co/api-solutions/