1/12
New @GoogleDeepMind paper 
We trained Foundational Large Autorater Models (FLAMe) on extensive human evaluations, achieving the best RewardBench perf. among generative models trained solely on permissive data, surpassing both GPT-4 & 4o.
: [2407.10817] Foundational Autoraters: Taming Large Language Models for Better Automatic Evaluation
:
2/12
Human evaluations often lack standardization & adequate documentation, limiting their reusability. To address this, we curated FLAMe, a diverse collection of standardized human evaluations under permissive licenses, incl. 100+ quality assessment tasks & 5M+ human judgments.
3/12
Our collection covers diverse task types, from assessing summary quality to evaluating how well models follow instructions. It focuses on key evaluation pillars: general response quality, instruction-following, factuality, mathematical reasoning, coding, & safety.
4/12
Training instruction-tuned LLMs on our FLAMe collection significantly improves generalization to a wide variety of held-out tasks. Overall, our FLAMe model variants outperform popular proprietary LLM-as-a-Judge models like GPT-4 on 8 out of 12 autorater evaluation benchmarks.
5/12
FLAMe variants are among the most powerful generative models on RewardBench. Notably, FLAMe-RM-24B achieves an overall score of 87.8%, the best performance among generative models trained only on permissively licensed data, surpassing both GPT-4-0125 (85.9) & GPT-4o (84.7).
6/12
FLAMe variants outperform LLM-as-a-Judge models in most of the common use cases on LLM-AggreFact (factuality/attribution evaluation). FLAMe-24B achieves the highest overall performance of 81.1, while the next-best model GPT-4-0125 scores 80.6.
7/12

Finally, our analysis reveals that FLAMe is significantly less biased than popular LLM-as-a-Judge models like GPT-4 on the CoBBLEr autorater bias benchmark and adept at identifying high-quality responses for code generation.
8/12
w/ wonderful collaborators @kalpeshk2011 (co-lead, equal contribution), Sal, @ctar, @manaalfar, & @yunhsuansung.
We hope FLAMe will spur more fundamental research into reusable human evaluations, & the development of effective & efficient LLM autoraters.
9/12
great work. congrats
10/12
Thanks, Zhiyang!
11/12
Great work! I also explored the autorater for code generation a year ago
[2304.14317] ICE-Score: Instructing Large Language Models to Evaluate Code
12/12
Cool work, thanks for sharing!
To post tweets in this format, more info here: https://www.thecoli.com/threads/tips-and-tricks-for-posting-the-coli-megathread.984734/post-52211196


We trained Foundational Large Autorater Models (FLAMe) on extensive human evaluations, achieving the best RewardBench perf. among generative models trained solely on permissive data, surpassing both GPT-4 & 4o.



2/12

3/12

4/12

5/12

6/12

7/12


8/12

We hope FLAMe will spur more fundamental research into reusable human evaluations, & the development of effective & efficient LLM autoraters.
9/12
great work. congrats

10/12
Thanks, Zhiyang!
11/12
Great work! I also explored the autorater for code generation a year ago

[2304.14317] ICE-Score: Instructing Large Language Models to Evaluate Code
12/12
Cool work, thanks for sharing!
To post tweets in this format, more info here: https://www.thecoli.com/threads/tips-and-tricks-for-posting-the-coli-megathread.984734/post-52211196
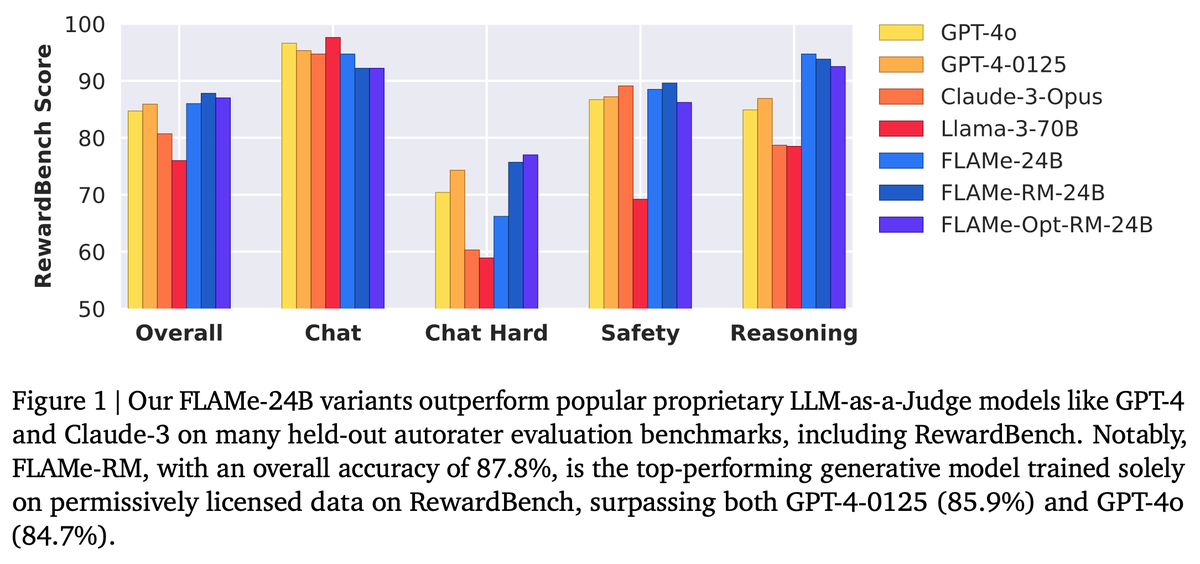
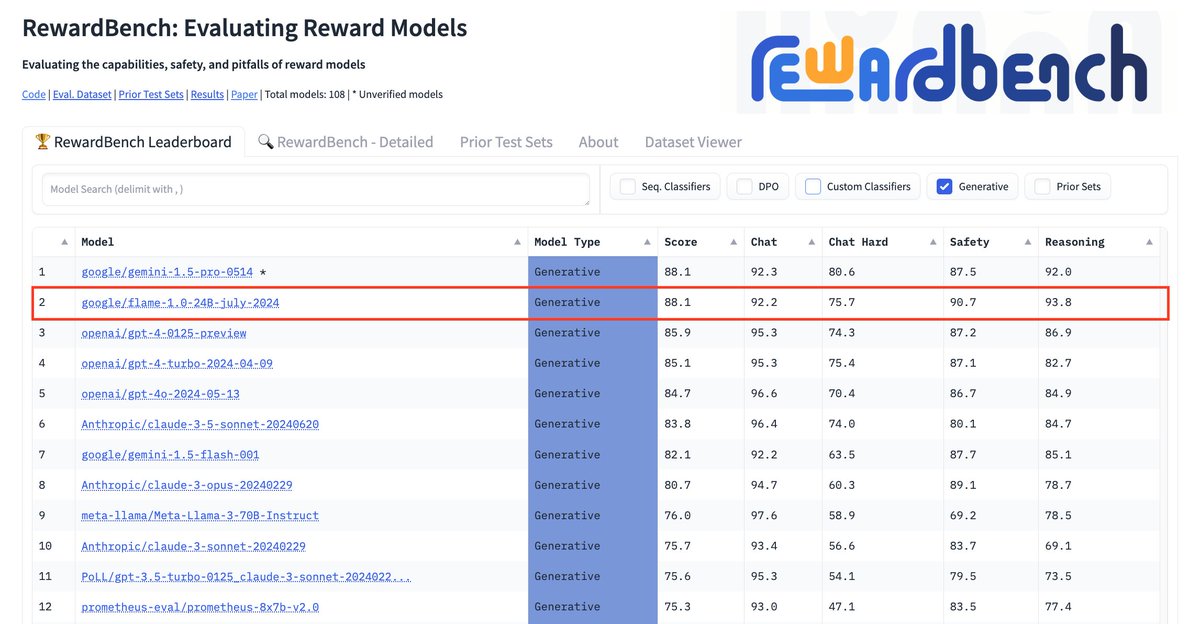
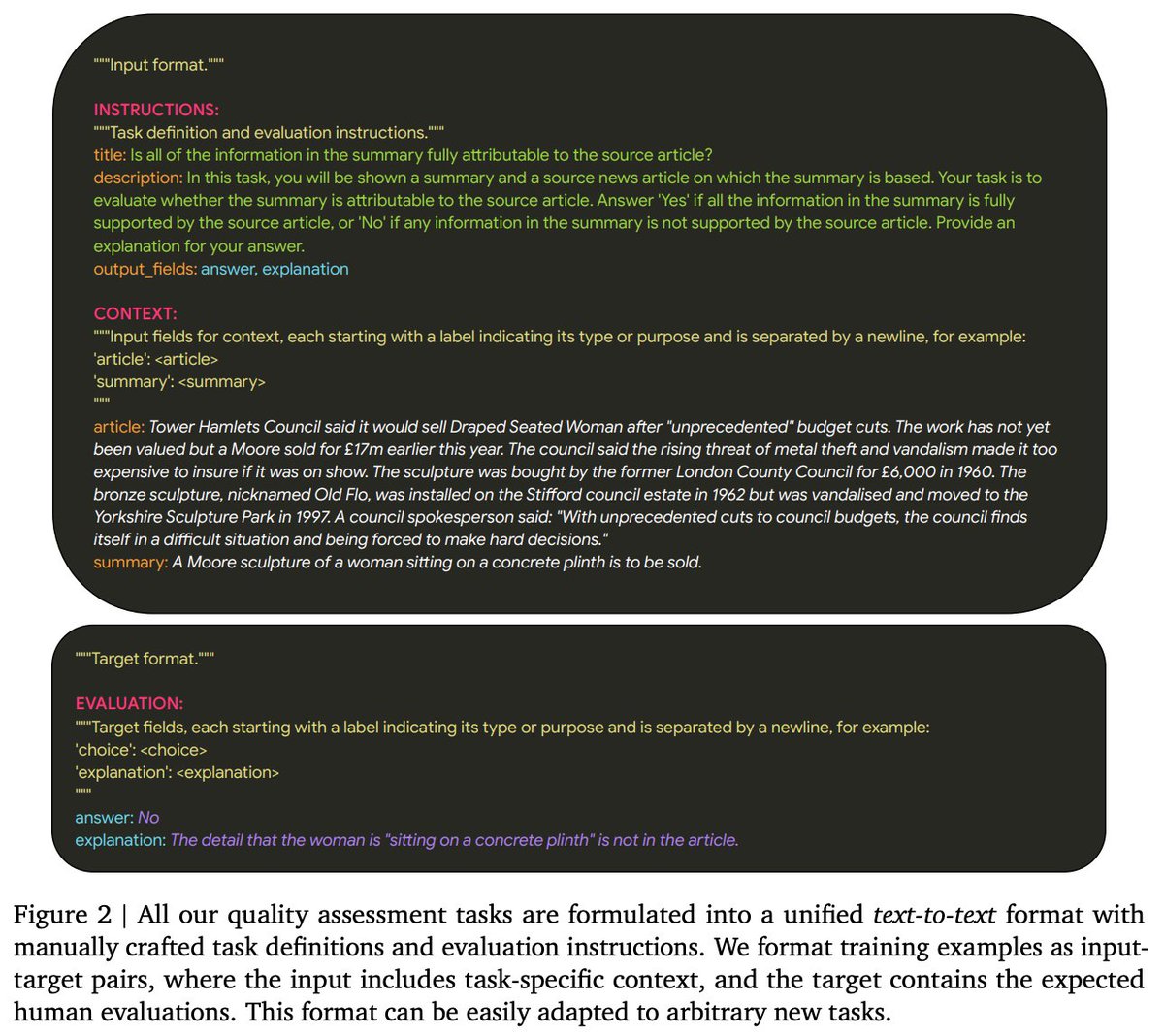
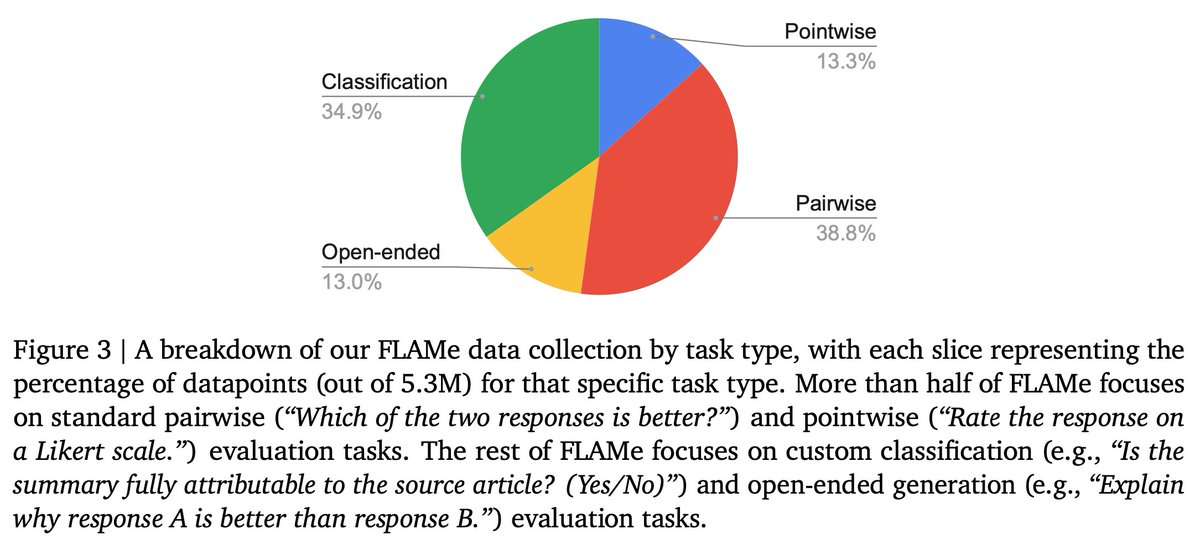
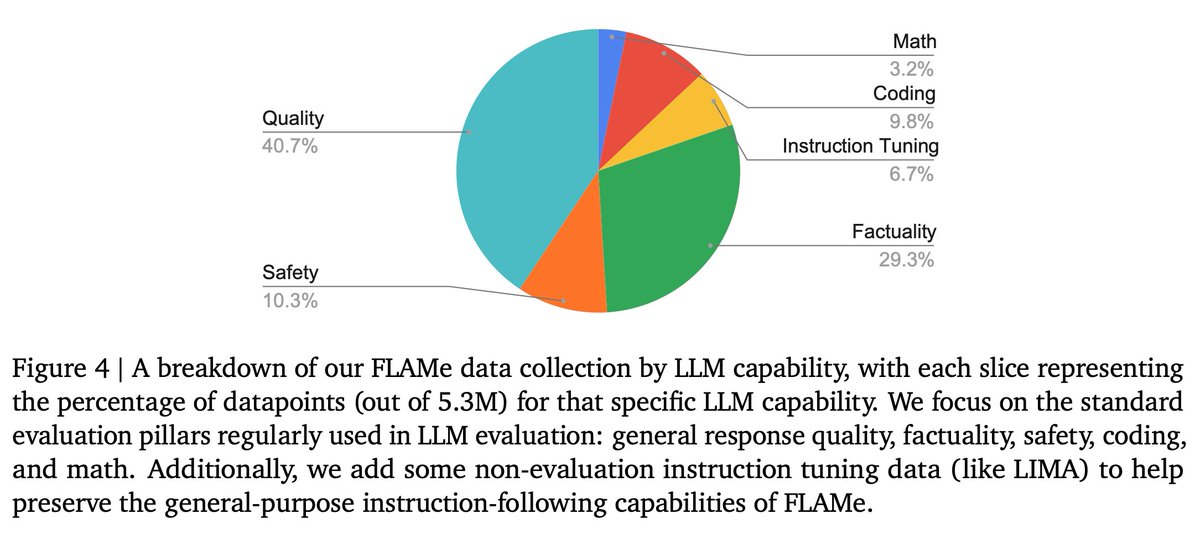
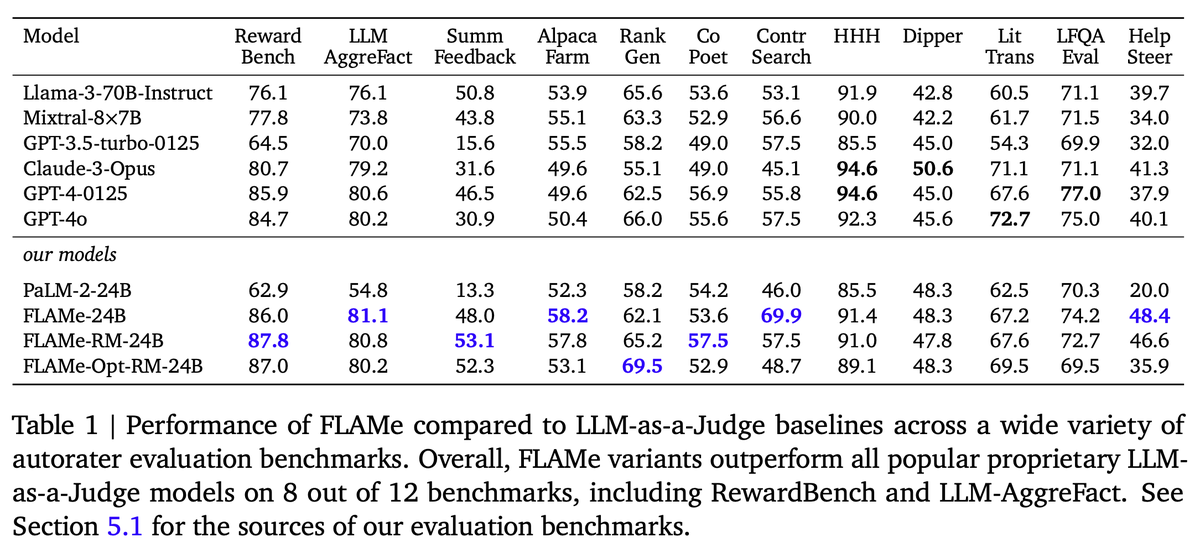
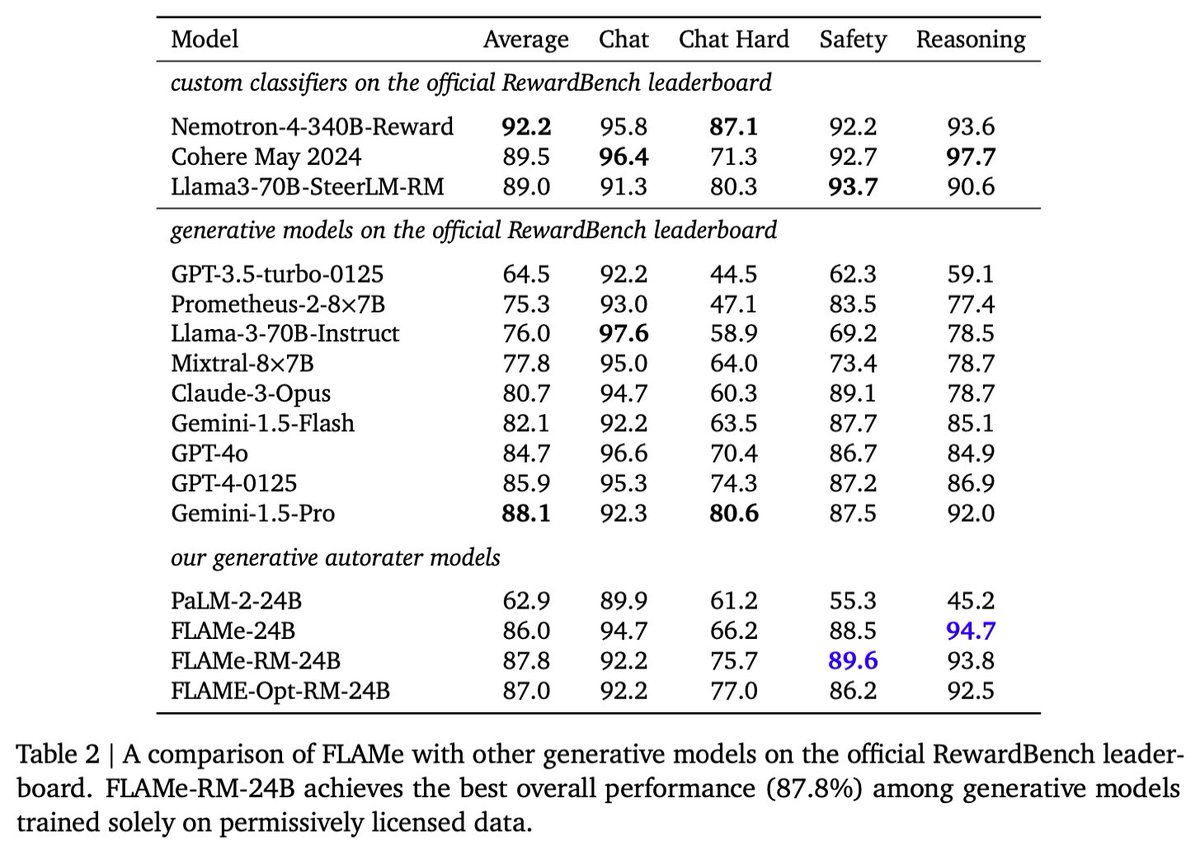
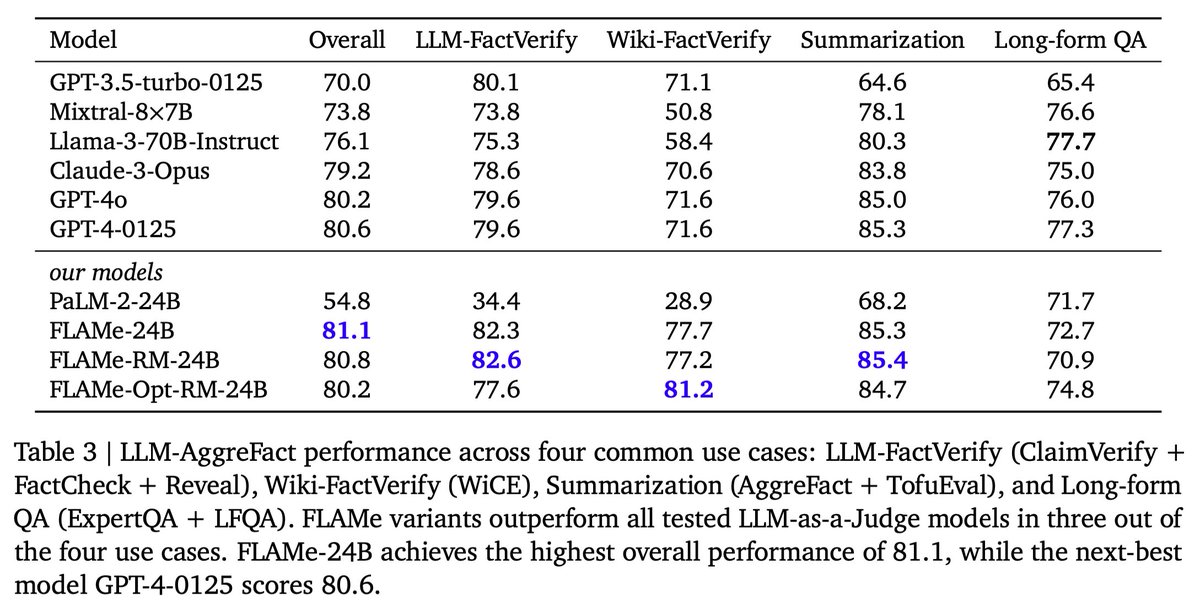
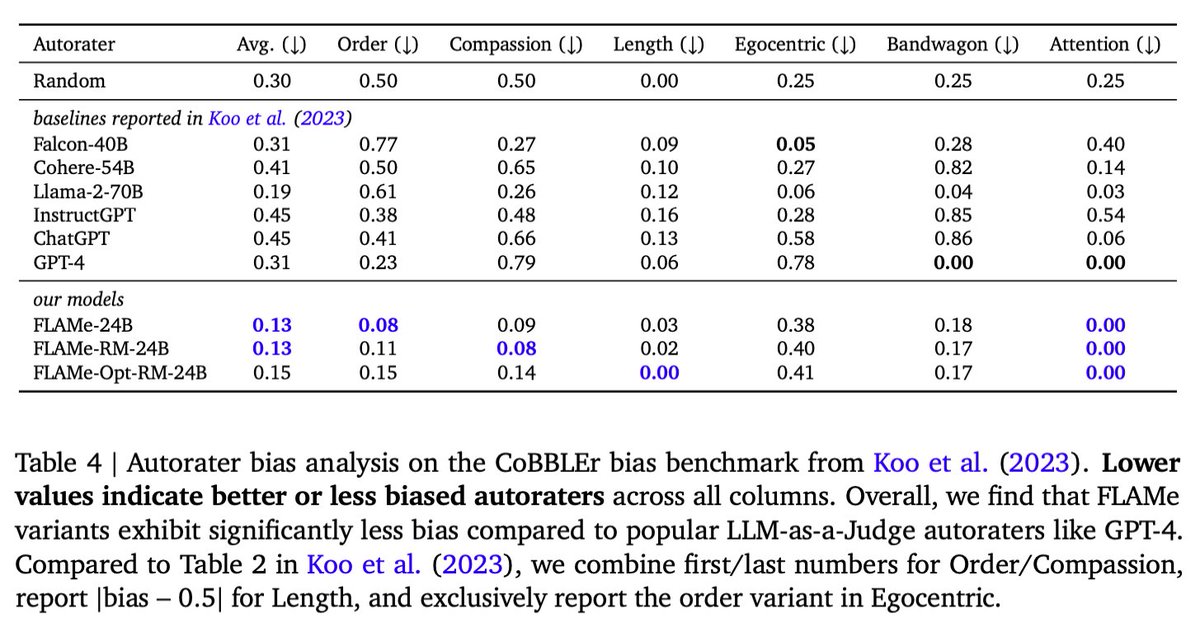
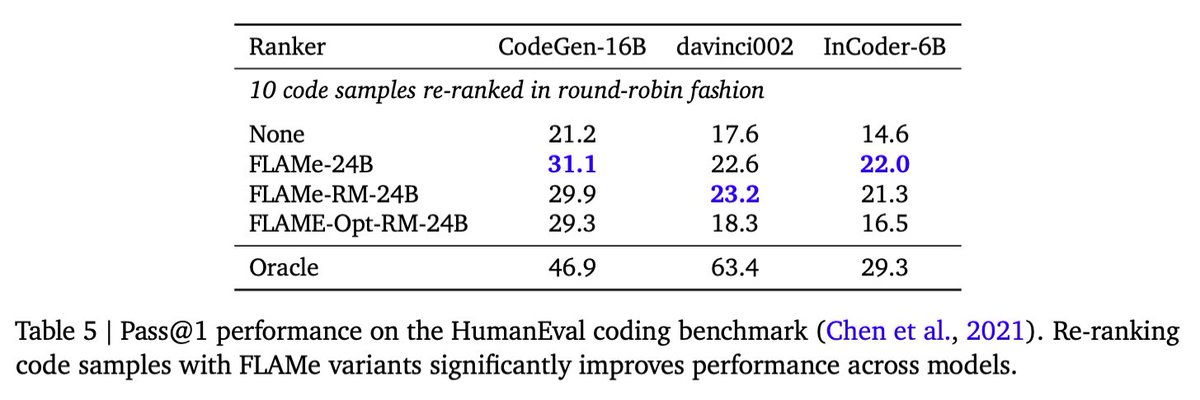
[2407.10817] Foundational Autoraters: Taming Large Language Models for Better Automatic Evaluation
[Submitted on 15 Jul 2024]
Foundational Autoraters - Taming Large Language Models for Better Automatic Evaluation
Tu Vu, Kalpesh Krishna, Salaheddin Alzubi, Chris Tar, Manaal Faruqui, Yun-Hsuan SungAbstract:
As large language models (LLMs) advance, it becomes more challenging to reliably evaluate their output due to the high costs of human evaluation. To make progress towards better LLM autoraters, we introduce FLAMe, a family of Foundational Large Autorater Models. FLAMe is trained on our large and diverse collection of 100+ quality assessment tasks comprising 5M+ human judgments, curated and standardized using publicly released human evaluations from previous research. FLAMe significantly improves generalization to a wide variety of held-out tasks, outperforming LLMs trained on proprietary data like GPT-4 and Claude-3 on many tasks. We show that FLAMe can also serve as a powerful starting point for further downstream fine-tuning, using reward modeling evaluation as a case study (FLAMe-RM). Notably, on RewardBench, our FLAMe-RM-24B model (with an accuracy of 87.8%) is the top-performing generative model trained exclusively on permissively licensed data, outperforming both GPT-4-0125 (85.9%) and GPT-4o (84.7%). Additionally, we explore a more computationally efficient approach using a novel tail-patch fine-tuning strategy to optimize our FLAMe multitask mixture for reward modeling evaluation (FLAMe-Opt-RM), offering competitive RewardBench performance while requiring approximately 25x less training datapoints. Overall, our FLAMe variants outperform all popular proprietary LLM-as-a-Judge models we consider across 8 out of 12 autorater evaluation benchmarks, encompassing 53 quality assessment tasks, including RewardBench and LLM-AggreFact. Finally, our analysis reveals that FLAMe is significantly less biased than these LLM-as-a-Judge models on the CoBBLEr autorater bias benchmark, while effectively identifying high-quality responses for code generation.
Comments: | 31 pages, 5 figures, 7 tables |
Subjects: | Computation and Language (cs.CL); Artificial Intelligence (cs.AI); Machine Learning (cs.LG) |
Cite as: | arXiv:2407.10817 |
arXiv:2407.10817v1 |
Submission history
From: [v1] [ view email][v1]
https://arxiv.org/pdf/2407.10817