
Animate Anyone
Animate Anyone: Consistent and Controllable Image-to-Video Synthesis for Character Animation
Animate Anyone: Consistent and Controllable Image-to-Video Synthesis for Character Animation
Li Hu, Xin Gao, Peng Zhang, Ke Sun, Bang Zhang, Liefeng BoInstitute for Intelligent Computing,Alibaba Group
Paper video Code arXiv
Abstract
Character Animation aims to generating character videos from still images through driving signals. Currently, diffusion models have become the mainstream in visual generation research, owing to their robust generative capabilities. However, challenges persist in the realm of image-to-video, especially in character animation, where temporally maintaining consistency with detailed information from character remains a formidable problem. In this paper, we leverage the power of diffusion models and propose a novel framework tailored for character animation. To preserve consistency of intricate appearance features from reference image, we design ReferenceNet to merge detail features via spatial attention. To ensure controllability and continuity, we introduce an efficient pose guider to direct character's movements and employ an effective temporal modeling approach to ensure smooth inter-frame transitions between video frames. By expanding the training data, our approach can animate arbitrary characters, yielding superior results in character animation compared to other image-to-video methods. Furthermore, we evaluate our method on benchmarks for fashion video and human dance synthesis, achieving state-of-the-art results.
Method
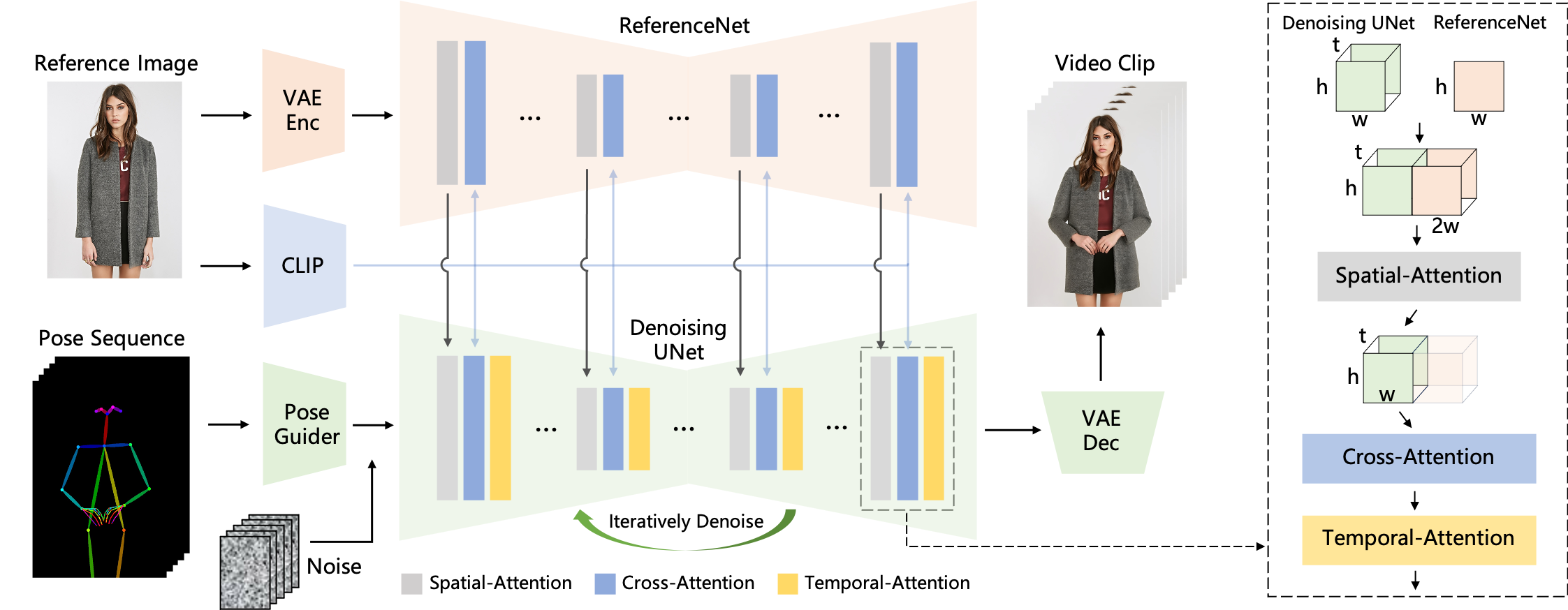
The overview of our method. The pose sequence is initially encoded using Pose Guider and fused with multi-frame noise, followed by the Denoising UNet conducting the denoising process for video generation. The computational block of the Denoising UNet consists of Spatial-Attention, Cross-Attention, and Temporal-Attention, as illustrated in the dashed box on the right. The integration of reference image involves two aspects. Firstly, detailed features are extracted through ReferenceNet and utilized for Spatial-Attention. Secondly, semantic features are extracted through the CLIP image encoder for Cross-Attention. Temporal-Attention operates in the temporal dimension. Finally, the VAE decoder decodes the result into a video clip.
GitHub - HumanAIGC/AnimateAnyone: Animate Anyone: Consistent and Controllable Image-to-Video Synthesis for Character Animation
Animate Anyone: Consistent and Controllable Image-to-Video Synthesis for Character Animation - HumanAIGC/AnimateAnyone
AnimateAnyone
Animate Anyone: Consistent and Controllable Image-to-Video Synthesis for Character AnimationLi Hu, Xin Gao, Peng Zhang, Ke Sun, Bang Zhang, Liefeng Bo
