null
...
In some instances yes. In others where a human being would tell you, "I don't know the answer, but let me research it"
It try to cobble together nonsense and output lies.
it is worse than that. you tell it to exclude an assumption or that something is wrong and it will often loop back to the same behaviour because it is incapable of following a multi-stage argument, while keeping salient points in scope and order of relevance.
and how could it? it is not reasoning anyway.
how it forgets even the simple instruction to give short direct answers, is beyond me.
why should it talk less? well apart from muddying the waters for the reader, it uses its own output as input. so conclusions it draws in long paragraphs of unwanted babble affect successive output. even if those inferences are off the beaten track.
it's a high functioning autist, characterised as having a predilection for verbosity, misinterpretation and fabrication, with an inability to reason, follow a train of argument or relatively contextualise past exchanges; all while possessing a very short memory.
borderline sociopathic and not quite the personality profile of a desirable employee.
it's built from a hodgepodge of millions of sometimes incorrect, sometimes contradictory, opinions and ways of doing things (over time). so accordingly, once past the veneer of open AI's behavioural controls it is the living embodiment of "design by [internet] committee".

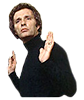
That becomes dangerous if you have no clue what you are doing but are trusting AI to give you the right answer.
The ability to say, I do not know is a skill in itself.
Last edited: