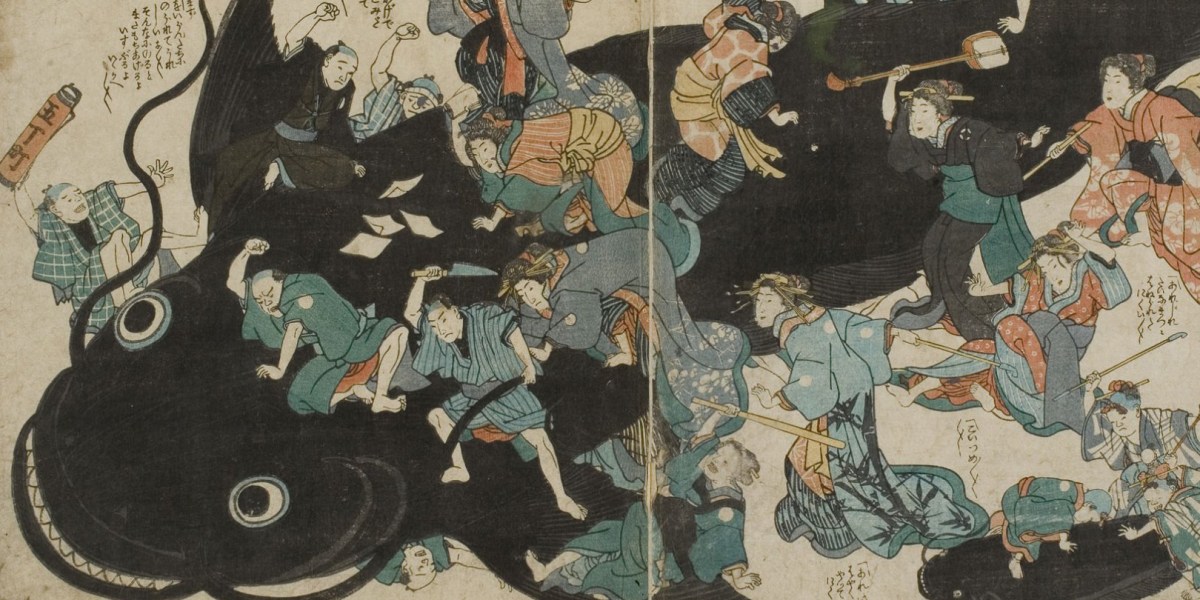
How machine learning might unlock earthquake prediction
Researchers are applying artificial intelligence and other techniques in the quest to forecast quakes in time to help people find safety.
How machine learning might unlock earthquake prediction
Researchers are applying artificial intelligence and other techniques in the quest to forecast quakes in time to help people find safety.By Allie Hutchisona rchive page
December 29, 2023
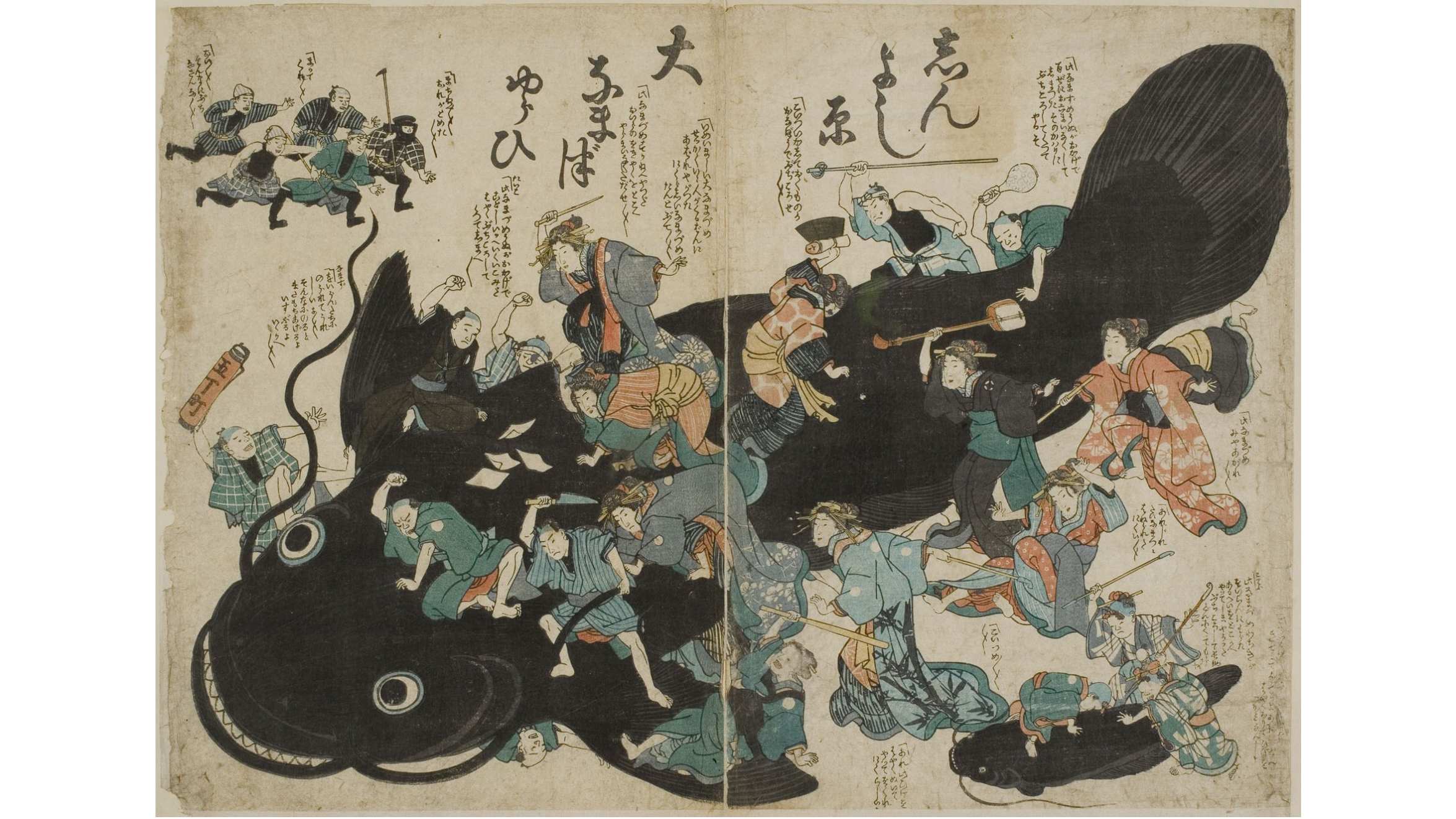
This Japanese woodblock print from 1855 depicts the namazu, a giant mythical catfish said to be responsible for earthquakes. In this scene, craftsmen who will profit from the reconstruction of the city hurry to rescue it from attacking peasants.WIKIMEDIA COMMONS
In September 2017, about two minutes before a magnitude 8.2 earthquake struck Mexico City, blaring sirens alerted residents that a quake was coming. Such alerts, which are now available in the United States, Japan, Turkey, Italy, and Romania, among other countries, have changed the way we think about the threat of earthquakes. They no longer have to take us entirely by surprise.
Earthquake early warning systems can send alarms through phones or transmit a loud signal to affected regions three to five seconds after a potentially damaging earthquake begins. First, seismometers close to the fault pick up the beginnings of the quake, and finely programmed algorithms determine its probable size. If it is moderate or large, the resulting alert then travels faster than the earthquake itself, giving seconds to minutes of warning. This window of time is crucial: in these brief moments, people can shut off electricity and gas lines, move fire trucks into the streets, and find safe places to go.
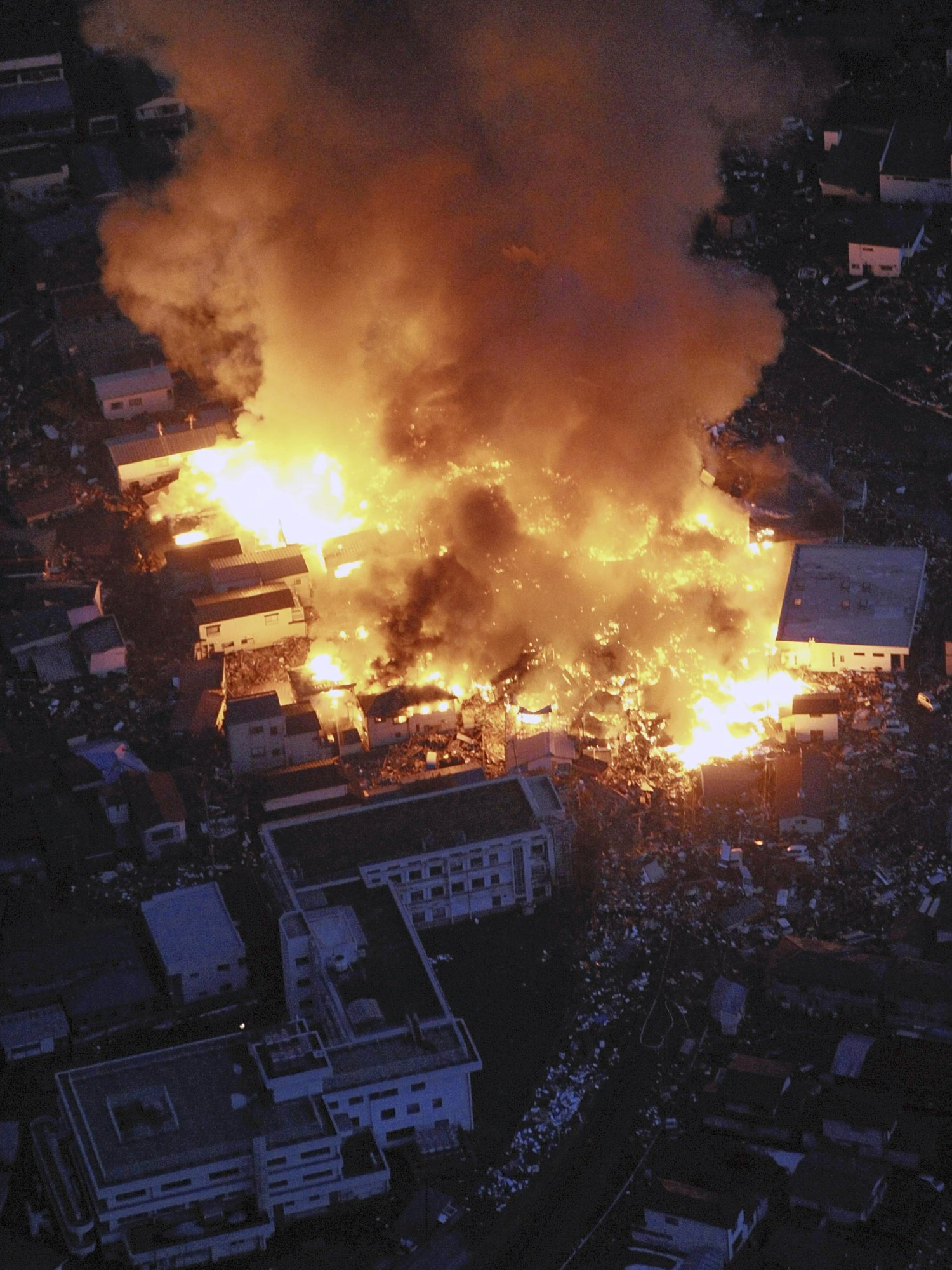
The magnitude 9 Tohoku-Oki earthquake of 2011 was preceded by two slow earthquakes.
AP IMAGES
But these systems have limitations. There are false positives and false negatives. What’s more, they react only to an earthquake that has already begun—we can’t predict an earthquake the way we can forecast the weather. And so many earthquake-prone regions are left in a state of constant suspense. A proper forecast could let us do a lot more to manage risk, from shutting down the power grid to evacuating residents.
When I started my PhD in seismology in 2013, the very topic of earthquake prediction was deemed unserious, as outside the realm of mainstream research as the hunt for the Loch Ness Monster.
But just seven years later, a lot had changed. When I began my second postdoc in 2020, I observed that scientists in the field had become much more open to earthquake prediction. The project I was a part of, Tectonic, was using machine learning to advance earthquake prediction. The European Research Council was sufficiently convinced of its potential to award it a four-year, €3.4 million grant that same year.
Today, a number of well-respected scientists are getting serious about the prospect of prediction and are making progress in their respective subdisciplines. Some are studying a different kind of slow-motion behavior along fault lines, which could turn out to be a useful indicator that the devastating kind of earthquake we all know and fear is on the way. Others are hoping to tease out hints from other data—signals in seismic noise, animal behavior, and electromagnetism—to push earthquake science toward the possibility of issuing warnings before the shaking begins.
In the dark
Earthquake physics can seem especially opaque. Astronomers can view the stars; biologists can observe an animal. But those of us who study earthquakes cannot see into the ground—at least not directly. Instead, we use proxies to understand what happens inside the Earth when its crust shakes: seismology, the study of the sound waves generated by movement within the interior; geodesy, the application of tools like GPS to measure how Earth’s surface changes over time; and paleoseismology, the study of relics of past earthquakes concealed in geologic layers of the landscape.Without good knowledge of what’s happening under the ground, it’s impossible to intuit any sense of order.
There is much we still don’t know. Decades after the theory of plate tectonics was widely accepted in the 1960s, our understanding of earthquake genesis hasn’t progressed far beyond the idea that stress builds to a critical threshold, at which point it is released through a quake. Different factors can make a fault more susceptible to reaching that point. The presence of fluids, for instance, is significant: the injection of wastewater fluid from oil and gas production has caused huge increases in tectonic activity across the central US in the last decade. But when it comes to knowing what is happening along a given fault line, we’re largely in the dark. We can construct an approximate map of a fault by using seismic waves and mapping earthquake locations, but we can’t directly measure the stress it is experiencing, nor can we quantify the threshold beyond which the ground will move.
For a long time, the best we could do regarding prediction was to get a sense of how often earthquakes happen in a particular region. For example, the last earthquake to rupture the entire length of the southern San Andreas Fault in California was in 1857. The average time period between big quakes there is estimated to be somewhere between 100 and 180 years. According to a back-of-the-envelope calculation, we could be “overdue.” But as the wide range suggests, recurrence intervals can vary wildly and may be misleading. The sample size is limited to the scope of human history and what we can still observe in the geologic record, which represents a small fraction of the earthquakes that have occurred over Earth’s history.
Related Story
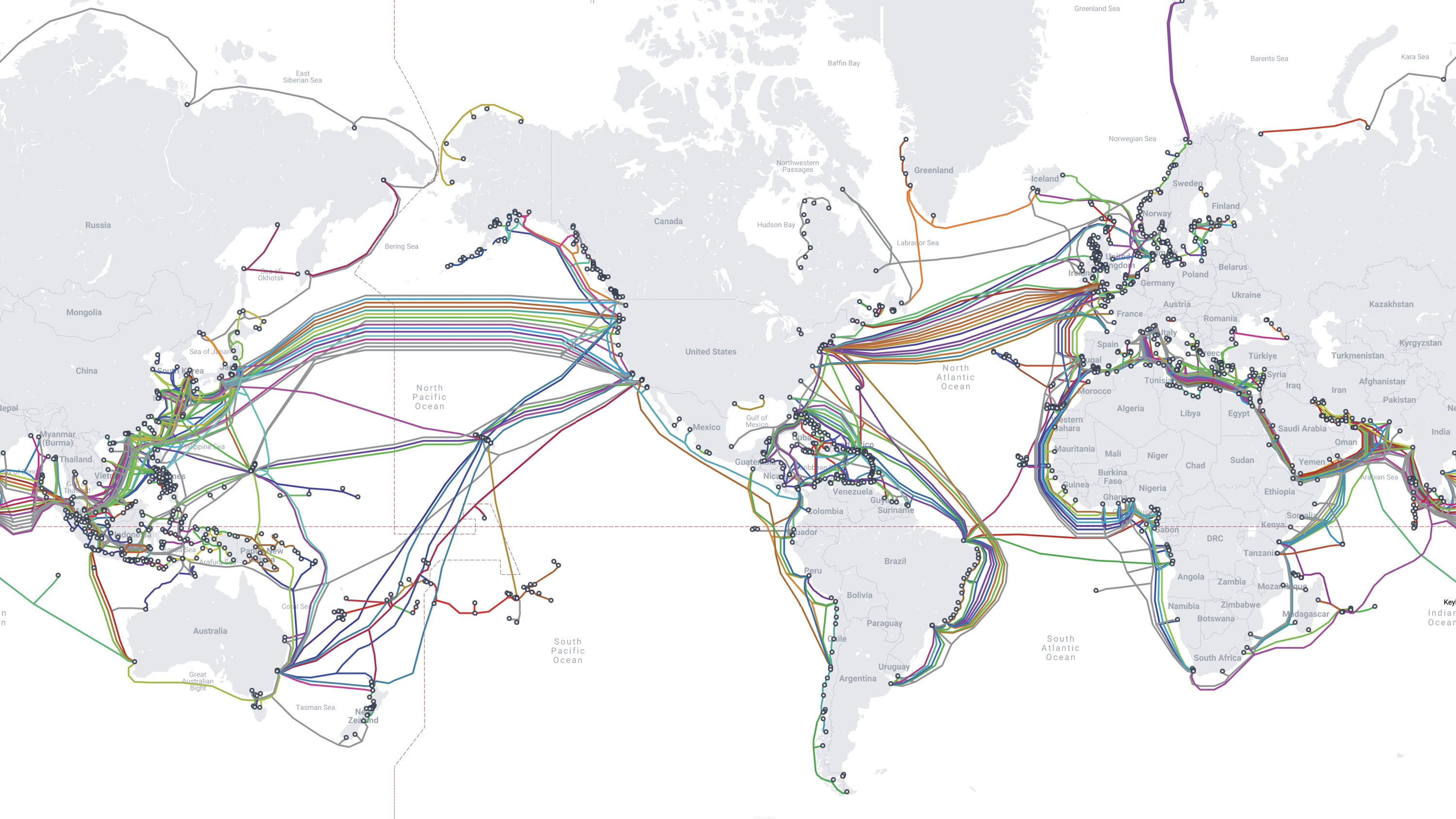
These underwater cables can improve tsunami detection
Telecom companies have long resisted letting scientific sensors piggyback on their subsea cables—until now.
In 1985, scientists began installing seismometers and other earthquake monitoring equipment along the Parkfield section of the San Andreas Fault, in central California. Six earthquakes in that section had occurred at unusually regular intervals compared to earthquakes along other faults, so scientists from the US Geological Survey (USGS) forecasted with a high degree of confidence that the next earthquake of a similar magnitude would occur before 1993. The experiment is largely considered a failure—the earthquake didn’t come until 2004.
Instances of regular intervals between earthquakes of similar magnitudes have been noted in other places, including Hawaii, but these are the exception, not the rule. Far more often, recurrence intervals are given as averages with large margins of error. For areas prone to large earthquakes, these intervals can be on the scale of hundreds of years, with uncertainty bars that also span hundreds of years. Clearly, this method of forecasting is far from an exact science.
Tom Heaton, a geophysicist at Caltech and a former senior scientist at the USGS, is skeptical that we will ever be able to predict earthquakes. He treats them largely as stochastic processes, meaning we can attach probabilities to events, but we can’t forecast them with any accuracy.
“In terms of physics, it’s a chaotic system,” Heaton says. Underlying it all is significant evidence that Earth’s behavior is ordered and deterministic. But without good knowledge of what’s happening under the ground, it’s impossible to intuit any sense of that order. “Sometimes when you say the word ‘chaos,’ people think [you] mean it’s a random system,” he says. “Chaotic means that it’s so complicated you cannot make predictions.”
But as scientists’ understanding of what’s happening inside Earth’s crust evolves and their tools become more advanced, it’s not unreasonable to expect that their ability to make predictions will improve.