
Generative AI Has a Visual Plagiarism Problem
Experiments with Midjourney and DALL-E 3 show a copyright minefield

Generative AI Has a Visual Plagiarism Problem
Experiments with Midjourney and DALL-E 3 show a copyright minefield
GARY MARCUS REID SOUTHEN
06 JAN 2024
19 MIN READ
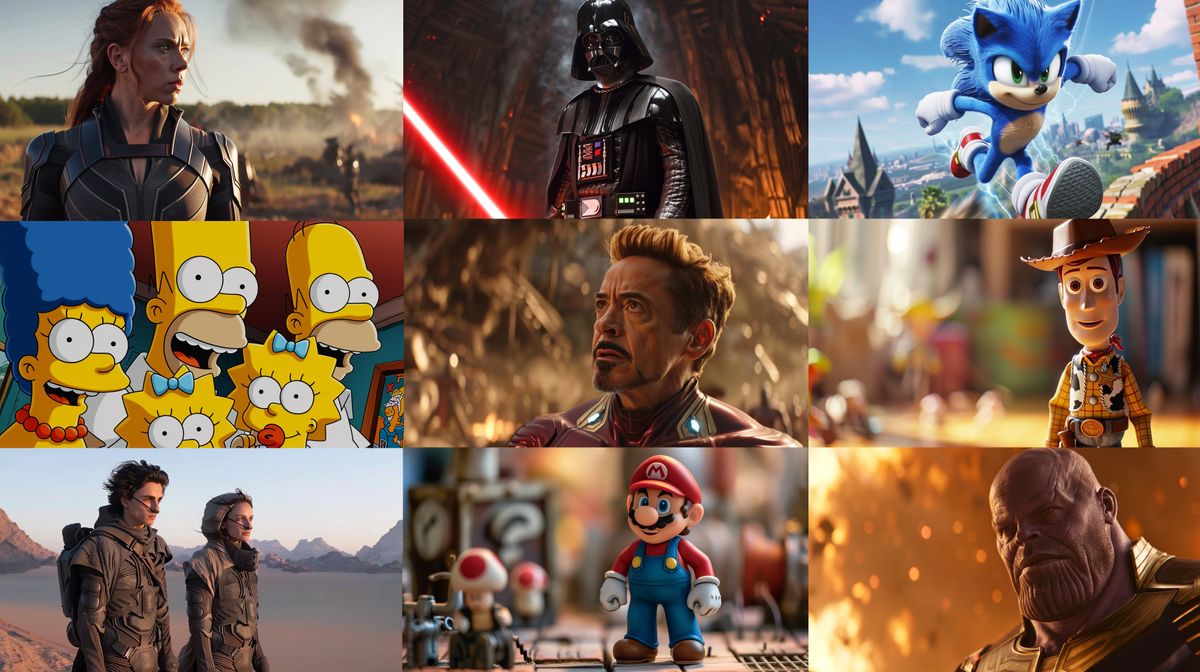
The authors found that Midjourney could create all these images, which appear to display copyrighted material.
GARY MARCUS AND REID SOUTHEN VIA MIDJOURNEY
GENERATIVE AI MIDJOURNEY OPENAI DALL-E 3 COPYRIGHT GENERATIVE AI
This is a guest post. The views expressed here are solely those of the authors and do not represent positions of IEEE Spectrum or the IEEE.
The degree to which large language models (LLMs) might “memorize” some of their training inputs has long been a question, raised by scholars including Google DeepMind’s Nicholas Carlini and the first author of this article (Gary Marcus). Recent empirical work has shown that LLMs are in some instances capable of reproducing, or reproducing with minor changes, substantial chunks of text that appear in their training sets.
For example, a 2023 paper by Milad Nasr and colleagues showed that LLMs can be prompted into dumping private information such as email address and phone numbers. Carlini and coauthors recently showed that larger chatbot models (though not smaller ones) sometimes regurgitated large chunks of text verbatim.
Similarly, the recent lawsuit that The New York Times filed against OpenAI showed many examples in which OpenAI software recreated New York Times stories nearly verbatim (words in red are verbatim):
An exhibit from a lawsuit shows seemingly plagiaristic outputs by OpenAI’s GPT-4. NEW YORK TIMES
We will call such near-verbatim outputs “plagiaristic outputs,” because prima facie if a human created them we would call them instances of plagiarism. Aside from a few brief remarks later, we leave it to lawyers to reflect on how such materials might be treated in full legal context.
In the language of mathematics, these example of near-verbatim reproduction are existence proofs. They do not directly answer the questions of how often such plagiaristic outputs occur or under precisely what circumstances they occur.
These results provide powerful evidence ... that at least some generative AI systems may produce plagiaristic outputs, even when not directly asked to do so, potentially exposing users to copyright infringement claims.
Such questions are hard to answer with precision, in part because LLMs are “black boxes”—systems in which we do not fully understand the relation between input (training data) and outputs. What’s more, outputs can vary unpredictably from one moment to the next. The prevalence of plagiaristic responses likely depends heavily on factors such as the size of the model and the exact nature of the training set. Since LLMs are fundamentally black boxes (even to their own makers, whether open-sourced or not), questions about plagiaristic prevalence can probably only be answered experimentally, and perhaps even then only tentatively.
Even though prevalence may vary, the mere existence of plagiaristic outputs raise many important questions, including technical questions (can anything be done to suppress such outputs?), sociological questions (what could happen to journalism as a consequence?), legal questions (would these outputs count as copyright infringement?), and practical questions (when an end-user generates something with a LLM, can the user feel comfortable that they are not infringing on copyright? Is there any way for a user who wishes not to infringe to be assured that they are not?).
The New York Times v. OpenAI lawsuit arguably makes a good case that these kinds of outputs do constitute copyright infringement. Lawyers may of course disagree, but it’s clear that quite a lot is riding on the very existence of these kinds of outputs—as well as on the outcome of that particular lawsuit, which could have significant financial and structural implications for the field of generative AI going forward.
Exactly parallel questions can be raised in the visual domain. Can image-generating models be induced to produce plagiaristic outputs based on copyright materials?
Case study: Plagiaristic visual outputs in Midjourney v6
Just before The New York Times v. OpenAI lawsuit was made public, we found that the answer is clearly yes, even without directly soliciting plagiaristic outputs. Here are some examples elicited from the “alpha” version of Midjourney V6 by the second author of this article, a visual artist who was worked on a number of major films (including The Matrix Resurrections, Blue Beetle, and The Hunger Games) with many of Hollywood’s best-known studios (including Marvel and Warner Bros.).
After a bit of experimentation (and in a discovery that led us to collaborate), Southen found that it was in fact easy to generate many plagiaristic outputs, with brief prompts related to commercial films (prompts are shown).
Midjourney produced images that are nearly identical to shots from well-known movies and video games.
We also found that cartoon characters could be easily replicated, as evinced by these generated images of the Simpsons.
Midjourney produced these recognizable images of The Simpsons.
In light of these results, it seems all but certain that Midjourney V6 has been trained on copyrighted materials (whether or not they have been licensed, we do not know) and that their tools could be used to create outputs that infringe. Just as we were sending this to press, we also found important related work by Carlini on visual images on the Stable Diffusion platform that converged on similar conclusions, albeit using a more complex, automated adversarial technique.
After this, we (Marcus and Southen) began to collaborate, and conduct further experiments.
Visual models can produce near replicas of trademarked characters with indirect prompts
In many of the examples above, we directly referenced a film (for example, Avengers: Infinity War); this established that Midjourney can recreate copyrighted materials knowingly, but left open a question of whether some one could potentially infringe without the user doing so deliberately.
In some ways the most compelling part of The New York Times complaint is that the plaintiffs established that plagiaristic responses could be elicited without invoking The New York Times at all. Rather than addressing the system with a prompt like “could you write an article in the style of The New York Times about such-and-such,” the plaintiffs elicited some plagiaristic responses simply by giving the first few words from a Times story, as in this example.
An exhibit from a lawsuit shows that GPT-4 produced seemingly plagiaristic text when prompted with the first few words of an actual article. NEW YORK TIMES
Such examples are particularly compelling because they raise the possibility that an end user might inadvertently produce infringing materials. We then asked whether a similar thing might happen in the visual domain.
The answer was a resounding yes. In each sample, we present a prompt and an output. In each image, the system has generated clearly recognizable characters (the Mandalorian, Darth Vader, Luke Skywalker, and more) that we assume are both copyrighted and trademarked; in no case were the source films or specific characters directly evoked by name. Crucially, the system was not asked to infringe, but the system yielded potentially infringing artwork, anyway.
Midjourney produced these recognizable images of Star Wars characters even though the prompts did not name the movies.
We saw this phenomenon play out with both movie and video game characters.
Midjourney generated these recognizable images of movie and video game characters even though the movies and games were not named.