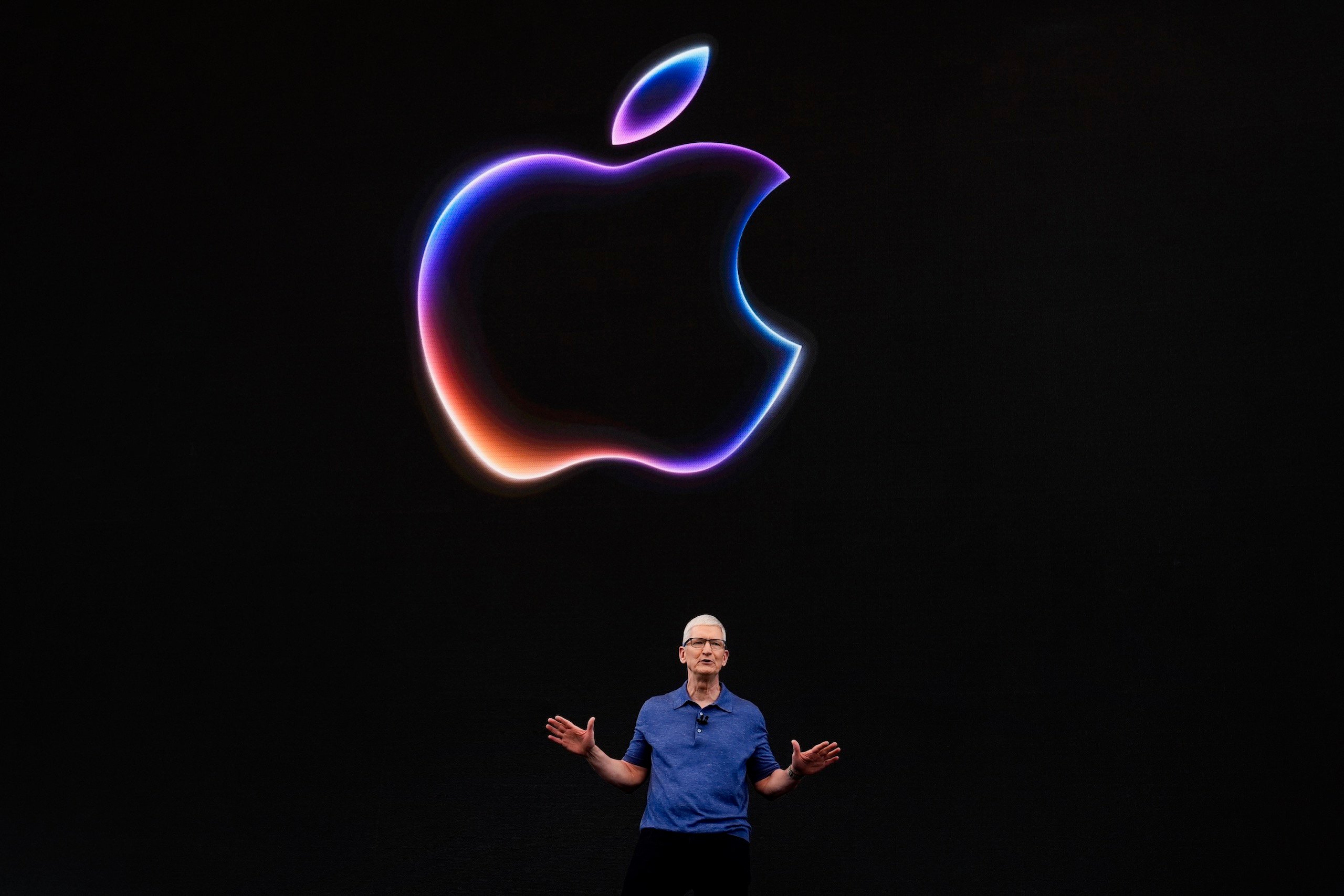
Apple leaps into AI with an array of upcoming iPhone features and a ChatGPT deal to smarten up Siri
Monday's showcase seemed aimed at allaying concerns Apple might be losing its edge during the advent of AI technology.

Apple leaps into AI with an array of upcoming iPhone features and a ChatGPT deal to smarten up Siri
Monday's showcase seemed aimed at allaying concerns Apple might be losing its edge during the advent of AI technology.ASSOCIATED PRESS / June 10, 2024
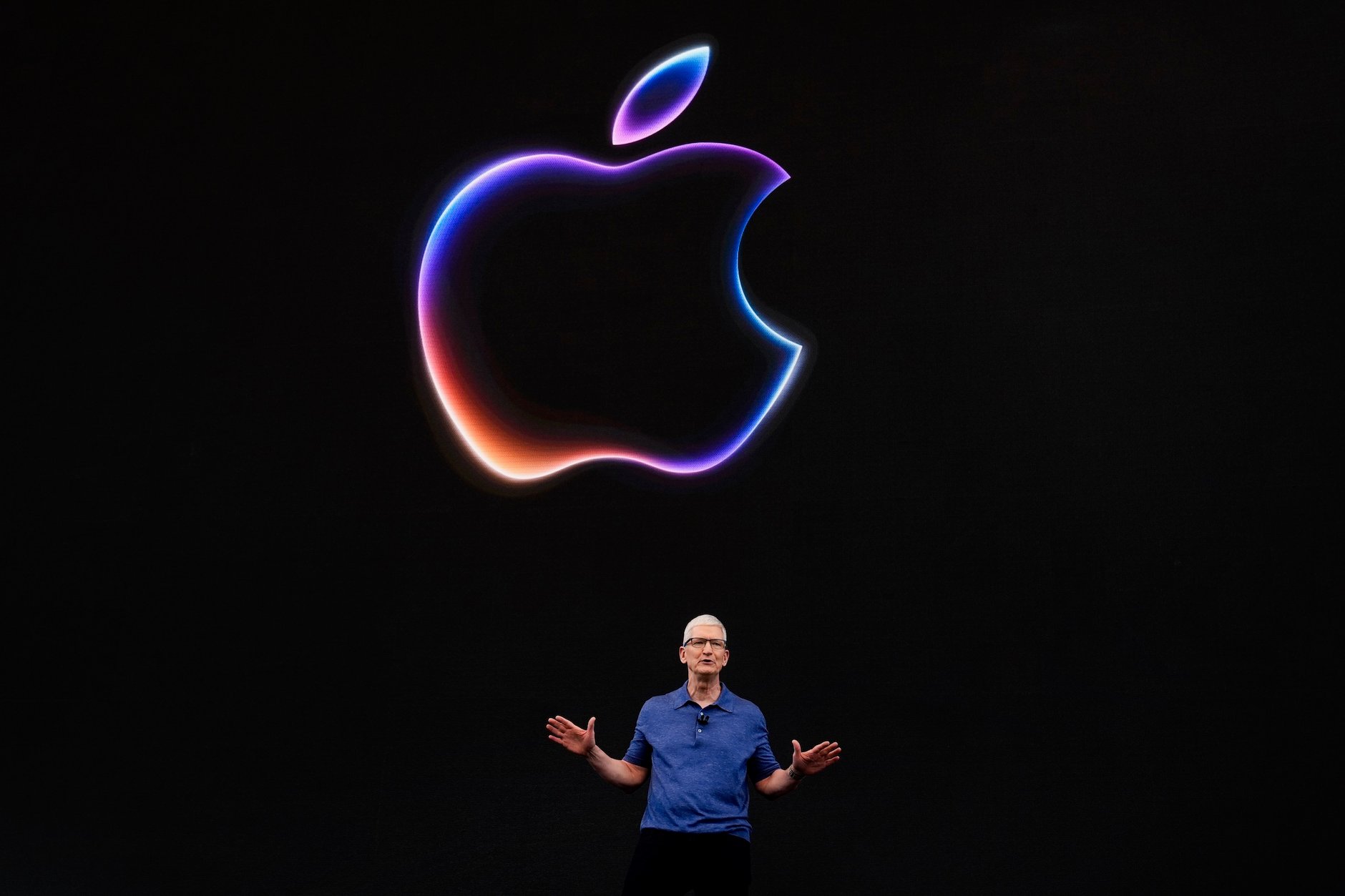
CUPERTINO, Calif. (AP) — Apple jumped into the race to bring generative artificial intelligence to the masses during its World Wide Developers Conference Monday that spotlighted an onslaught of features designed to soup up the iPhone, iPad and Mac.
And in a move befitting a company known for its marketing prowess, the AI technology coming as part of free software updates later this year, is being billed as “Apple Intelligence.”
Even as it tried to put its own stamp on the hottest area of technology, Apple tacitly acknowledged it needed help to catch up with companies like Microsoft and Google, who have emerged as the early leaders in the AI field. Apple is leaning on ChatGPT, made by the San Francisco startup OpenAI, to help make its often-bumbling virtual assistant Siri smarter and more helpful.
“All of this goes beyond artificial intelligence, it's personal intelligence, and it is the next big step for Apple,” Apple CEO Tim Cook said.
Click to accept the cookies for this service
Courthouse News’ podcast Sidebar tackles the stories you need to know from the legal world. Join our hosts as they take you in and out of courtrooms in the U.S. and beyond.
Siri's gateway to ChatGPT will be free to all iPhone users and made available on other Apple products once the option is baked into the next generation of Apple's operating systems. ChatGPT subscribers are supposed to be able to easily sync their existing accounts when using the iPhone, and should get more advanced features than free users would.
To herald the alliance with Apple, OpenAI CEO Sam Altman sat in the front row of the packed conference, which included developers attending from more than 60 countries worldwide.
“Think you will really like it,” Altman predicted in a post about their partnership with Apple.
Beyond giving Siri the ability to tap into ChatGPT's knowledge base. Apple is giving its 13-year-old virtual assistant an extensive makeover designed to make it more personable and versatile, even as it currently fields about 1.5 billion queries a day.
When Apple releases free updates to the software powering the iPhone and its other products this autumn, Siri will signal its presence with flashing lights along the edges of the display screen, and be able to handle hundreds of more tasks — including chores that may require tapping into third-party devices — than it can now, based on Monday's presentations.
The AI-packed updates coming to the next versions of Apple software are meant to enable the billions of people who use its devices to get more done in less time, while also giving them access to creative tools that could liven things up. For instance, Apple will deploy AI to allow people to create emojis, dubbed “Genmojis” on the fly to fit the vibe they are trying to convey.
Monday's showcase seemed aimed at allaying concerns Apple might be losing its edge during the advent of AI technology that is expected to be as revolutionary as the 2007 invention of the Phone. Both Google and Samsung have already released smartphone models touting AI features as their main attractions while Apple has been stuck in an uncharacteristically extended slump in the company’s sales.
AI mania is the main reason that Nvidia, the dominant maker of the chips underlying the technology, has seen its market value rocket from about $300 billion at the end of 2022 to about $3 trillion. The meteoric ride allowed Nvidia to surpass Apple as the second most valuable company in the U.S. Earlier this year, Microsoft also eclipsed the iPhone maker on the strength of its so-far successful push into AI.
Investors didn't seem as impressed with Apple's AI presentation as the crowd that came to the company's Cupertino, California, headquarters to see it. Apple's stock price declined nearly 2% in Monday's trading after Cook walked off the stage.
Despite that negative reaction, Wedbush Securities analyst Dan Ives asserted that Apple is “taking the right path” in a research note that hailed the presentation as a “historical” day for a company that already has reshaped the tech industry and society.
Besides pulling AI tricks out of its toolbox, Apple also used the conference to confirm that it will be rolling out a technology called Rich Communications Service, or RCS, to its iMessage app that should improve the quality and security of texting between iPhones and devices powered by Android software, such as the Samsung Galaxy and Google Pixel.
The change, due out with the next version of iPhone's operating software won't eliminate the blue bubbles denoting texts originating from iPhones and the green bubbles marking text sent from Android devices — a distinction that has become a source of social stigma.
This marked the second straight year that Apple has created a stir at its developers conference by using it to usher in a trendy form of technology that other companies already are on the market with.
Last year, Apple provided an early look at its mixed-reality headset, the Vision Pro, which wasn't released until early 2024. Nevertheless, Apple's push into mixed reality — with a twist that it bills as “spatial computing” — has raised hopes that there will be more consumer interest in this niche technology.
Part of that optimism stems from Apple's history of releasing technology later than others, then using sleek designs and slick marketing campaigns to overcome its tardy start.
Bringing more AI to the iPhone will likely raise privacy concerns — a topic that Apple has gone to great lengths to assure its loyal customers it can be trusted not to peer too deeply into their personal lives. Apple did talk extensively Monday about its efforts to build strong privacy protections and controls around its AI technology.
One way Apple is trying to convince consumers that the iPhone won't be used to spy on them is harnessing its chip technology so most of its AI-powered features are handled on the device itself instead of at remote data centers, often called “the cloud.” Going down this route would also help protect Apple's profit margins because AI processing through the cloud is far more expensive than when it is run solely on a device.
Apple's AI “will be aware of your personal l data without collecting your personal data,” said Craig Federighi, Apple's senior vice president of software engineering.
__
By MICHAEL LIEDTKE AP Technology Writer